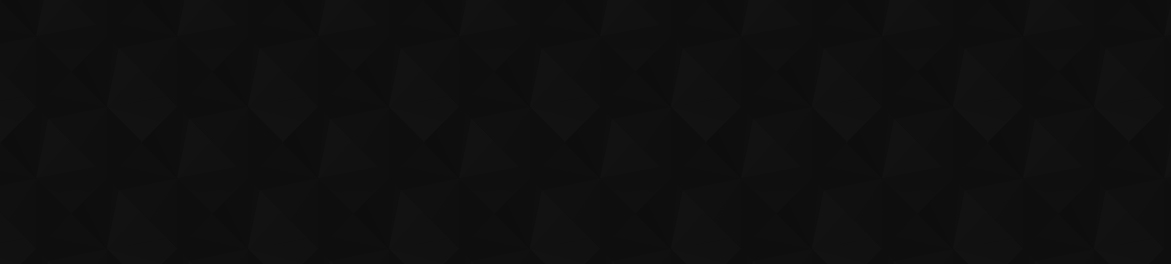
- Видео 35
- Просмотров 201 389
Learn Statistics with Brian
Добавлен 16 июн 2021
Statistics, made easy.
The easiest way to support the channel is to follow the linktree below to my full-length statistics courses offered on Udemy. The courses provide a more structured way to learn introductory statistics, mathematical statistics, Bayesian statistics, R, and SQL.
The easiest way to support the channel is to follow the linktree below to my full-length statistics courses offered on Udemy. The courses provide a more structured way to learn introductory statistics, mathematical statistics, Bayesian statistics, R, and SQL.
What is Least Squares Linear Regression?
What do we mean by least squares, and why do we use it?
Просмотров: 280
Видео
Likelihood Ratio Tests Clearly Explained
Просмотров 63314 дней назад
What is a likelihood ratio test (LRT) in statistics?
The Physics Behind Probability Mass and Probability Density Functions
Просмотров 1 тыс.28 дней назад
Buy my full-length statistics, data science, and SQL courses: linktr.ee/briangreco Why are they called probability mass and probability density functions? What's the difference between a pmf and a pdf?
Fail to reject the null hypothesis?
Просмотров 720Месяц назад
Why do people say "I fail to reject the null hypothesis"?????
What is a consistent estimator in statistics?
Просмотров 1,2 тыс.Месяц назад
What does it mean for a statistical estimator to be consistent? Buy my full-length statistics, data science, and SQL courses here: linktr.ee/briangreco
Complete Statistics, Ancillary Statistics, and Basu's Theorem
Просмотров 450Месяц назад
Learn about ancillarity, complete statistics, and Basu’s Theorem! Sufficient Statistics: ruclips.net/video/J-TTqCgRzbM/видео.html Minimal Sufficient Statistics: ruclips.net/video/IsgteDaNTFk/видео.html Buy my full-length statistics, data science, and SQL courses here: linktr.ee/briangreco
Minimal Sufficient Statistics Clearly Explained
Просмотров 627Месяц назад
You’ve learned what a sufficient statistic is, but what does it mean to be minimal sufficient? Sufficient Statistics: ruclips.net/video/J-TTqCgRzbM/видео.html Buy my full-length statistics, data science, and SQL courses here: linktr.ee/briangreco
Chi-squared tests ... MADE EASY!!!
Просмотров 2902 месяца назад
Learn about Chi-Squared Tests - Goodness of fit (GOF), Independence, and Homogeneity, oh my! Buy my full-length statistics, data science, and SQL courses here: linktr.ee/briangreco
The Rao-Blackwell Theorem Explained
Просмотров 1,5 тыс.2 месяца назад
Learn about Rao-Blackwellization, a way to use sufficient statistics to improve your statistical estimators! Learn more about Sufficient Statistics and the Factorization Theorem: ruclips.net/video/J-TTqCgRzbM/видео.html Buy my full-length statistics, data science, and SQL courses here: linktr.ee/briangreco
The EM Algorithm Clearly Explained (Expectation-Maximization Algorithm)
Просмотров 5 тыс.2 месяца назад
Learn all about the EM algorithm, a way to find maximum likelihood estimates in problems with missing data. Buy my full-length statistics, data science, and SQL courses here: linktr.ee/briangreco
p-values explained, in 5 levels of complexity
Просмотров 3,3 тыс.3 месяца назад
Learn all about what p-values and how to interpret them in statistical hypothesis testing! Buy my full-length statistics, data science, and SQL courses here: linktr.ee/briangreco
What is a degree of freedom?
Просмотров 1,1 тыс.3 месяца назад
Learn about the mysterious, hard-to-interpret number used in many statistical methods, degrees of freedom (df). Buy my full-length statistics, data science, and SQL courses here: linktr.ee/briangreco
The Monty Hall Problem without probability
Просмотров 8004 месяца назад
The Monty Hall Problem is a classic probability puzzle with a counter-intuitive result. Instead of using probability trees, let's try to deeply understand the problem!
Analysis of Variance (ANOVA) and F statistics .... MADE EASY!!!
Просмотров 3934 месяца назад
Learn the intuition behind ANOVA and calculating F statistics! Buy my full-length statistics, data science, and SQL courses here: linktr.ee/briangreco
The Cramer-Rao Lower Bound ... MADE EASY!!!
Просмотров 4,3 тыс.5 месяцев назад
What is a Cramer-Rao Lower Bound? How can we prove an estimator is the best possible estimator? What is the efficiency of an estimator? Buy my full-length statistics, data science, and SQL courses here: linktr.ee/briangreco
Outliers in Data Analysis... and how to deal with them!
Просмотров 3,2 тыс.6 месяцев назад
Outliers in Data Analysis... and how to deal with them!
Link functions for GLMs... MADE EASY!!!
Просмотров 1 тыс.7 месяцев назад
Link functions for GLMs... MADE EASY!!!
The Uniform Distribution MLE is... very UNLIKELY
Просмотров 6857 месяцев назад
The Uniform Distribution MLE is... very UNLIKELY
Bayesian vs. Frequentist Statistics ... MADE EASY!!!
Просмотров 16 тыс.7 месяцев назад
Bayesian vs. Frequentist Statistics ... MADE EASY!!!
Maximum Likelihood Estimation ... MADE EASY!!!
Просмотров 25 тыс.7 месяцев назад
Maximum Likelihood Estimation ... MADE EASY!!!
The simplest non-parametric test... The Sign Test
Просмотров 1,6 тыс.7 месяцев назад
The simplest non-parametric test... The Sign Test
Unbiased Estimators ... Made Easy!
Просмотров 5 тыс.7 месяцев назад
Unbiased Estimators ... Made Easy!
Can more data be BAD??? (The 10% rule and Finite Population Correction)
Просмотров 2927 месяцев назад
Can more data be BAD??? (The 10% rule and Finite Population Correction)
Inverse Transform Sampling ... MADE EASY!!!
Просмотров 2,5 тыс.7 месяцев назад
Inverse Transform Sampling ... MADE EASY!!!
Heteroskedasticity and Homoskedasticity... What are they???
Просмотров 2,8 тыс.7 месяцев назад
Heteroskedasticity and Homoskedasticity... What are they???
Independent vs Mutually Exclusive Events ... MADE EASY!!!
Просмотров 2,1 тыс.7 месяцев назад
Independent vs Mutually Exclusive Events ... MADE EASY!!!
Probability vs. Likelihood ... MADE EASY!!!
Просмотров 38 тыс.8 месяцев назад
Probability vs. Likelihood ... MADE EASY!!!
The Method of Moments ... Made Easy!
Просмотров 23 тыс.8 месяцев назад
The Method of Moments ... Made Easy!
If the one explaining truly understands the concept well, his explanation will be easy to understand. So, if I haven't been able to grasp my professor's convoluted explanations all this time, it could be because he doesn't actually understand the concept very well.
thank you so much! this helped a lot 😊
awesome explanation.
9:42 Is the log(0.8) meant to be log(1.8)? Nice video btw.
Yes, exactly, good catch.
Thank you, but one thing I still don't get about about the Bayesian way of thinking: if one is supposed to constantly update a belief via data, then where did the prior come from? How does one quantify a belief based on zero data?
Great explanation Brian! I have a small question, though. If the response variable has to be normal (in a normal linear regression), why do you think most statistics articles insist that only the residuals have to be normal and not the variable? What tests do you think should be done before a GLM, besides residual plots?
Saying the response is normal and the residuals are normal means the same thing basically. The response is normal (around the mean for that X value), which just means the response’s distance from the mean (residual) is normal with mean 0. If we want to evaluate normality of residuals, it’s then easier to look at a graph of residual since they all have the same mean so we can easily visualize if they seem normally distributed.
Amazing. Enlightening
Love the video. These concepts are explained very nicely and in a easy to understand manner.
Very clear explanation. Thanks.
great content, keep it up
Is there any reason to have two different ways of solving it? One at 5:43 and another at the end. Which one do you think is better and why ?
They are the same way, least squares. The last part is just a little extra justification on “why” - least squares is very related to normal distributions.
you have great videos !! thank you so much <3
Thank you! :)
perfect and easiest explanation in utube....thanku so much sir it is really helpful
Great Content
Good examples
❤
if I can i will give a 100 likes, best explanation that i have found for this topic so far
Excellent video! It really explains this concept in a simple manner!
I got this question that says let 𝑋 be a discrete random variable where P(𝑋=x) are some function of 𝜃(e.g. 𝜃/2, 1-𝜃) for some number x, and it ask "Find the Method of Moments Estimator of 𝜃 by using 𝑛 copies of 𝑋, where 𝑛 > 1.", what does " using 𝑛 copies of 𝑋" means?
A sample of size n. They mean the same thing as in this video - set xbar (which is based on the n data points) equal to E(X) which is a function of theta
Please, don't stop creating the content! I am so grateful for you! The stats at universities are not properly explained, infortunately, you make difficult things seem easy indeed!
Thank you, much more to come! :)
This is an absolutely incredible video. Amazing information. Please keep doing your thing
Universe!!! It had broken my stone, my head. Thank you!
Finally got the point. Not even ChatGPT or Claude explained this topic as easily as you did. Thanks !!
Brooo, i am very thankful someone is choosing the things to explain where on avg every student feel confused and tensed. Thank you very much. It was helpful ❤️
Thank you very much bro. This was really helpful, nicely explained ❤️🍁. Have a bright day🌻
Best and clear cut video. It made sense to me. Thanks, man 👏🏽
Amazing explanation
Could you do a video on the Neyman Pearson lemma ?
I talk about it a little in this video, and I don't have a lot more to say about it than I included here, so probably not. Is there something in particular about it you're looking to learn?
You could ask which country you are in 😂 Great video tho
The video would have been very short. 😂 I laughed out loud, thanks!
Excellent!
Seriously very clean and simple explanation , keep continuing these lectures
I'm a fan of your videos! So well explained! Keep up the good work :)
Thank you!
Thanks!
Thank you!
Thanks!
Thank you!
I am on my second year of education in uni and these theme was explained awful and I had no idea of what was going on but after watching I am wondering how professor managed to explain such an easy thing in such difficult way thank you so much❤
Exactly what the title says! Thank you
05:30 The probabiliy that X=1 is 1. It's certain since we've already observed it. The question makes no sense simply because it makes no sense to talk about probability for something that already happened. The same holds true even for discrete probability.
The implication is that our observation X=1 is just one *sample* from a probability distribution of a random variable X that has a distribution, and other samples will not all be X=1. So the P(X=1) is not one, but P(this particular realization of X is 1)=1, yes.
@@statswithbrianI see. You meant to ask what is the probability that the next observation will be one given that we have already one observation with X=1.
@lbognini I definitely did not mean that. That is a Bayesian concept (a posterior predictive distribution) that is not related to this.
thank's Mr. Brian
I don't understand the first "not-complete" example. E[X_1 - X_2] = E[X_1] - E[X_2] = mu - mu = 0, no?
Correct - it’s 0. The definition of completeness tells us that if that expected value is zero, then the function must also be 0 everywhere. But X1-X2 is basically never 0.
i liked it
So many people say physics does not apply to the stock market, but after watching this video I must disagree.
Great video, sincerely a second year stat major.
Great explanation, thanks.
This content is excellent. Would a better microphone or audio setup be a bad idea?
Working on it. Next videos will be improved.
I round up. I'm not 70.238749327429 inches tall, I'm 71 and I'm sticking to it.
why we use a formula of (predicted weight = -500+10*height)? Why 500 and 10?
We don't - I just made those numbers up for the example for easy computation. In reality, we will use the least-squares regression line (which in this example was the predicted weight = -439.286+8.929*height). That's not obvious at all, you'd have to have the data and compute a regression line.
Great straightforward intuitive explanation.
Soo good! Didn't get it in class at all
I'm so grateful for all the videos you make that inspire our curiosity!
Thank you! :)
Constant question, what is the probability of??? Happens with recruiting