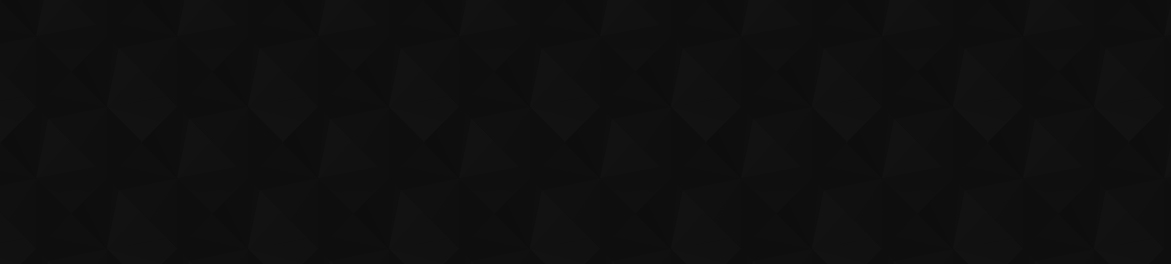
- Видео 38
- Просмотров 113 714
Nicolas Vandeput
Добавлен 15 окт 2011
Join me for content related to Inventory Optimization and Demand Forecasting.
Demand planners shouldn't do this
Demand planners are not computers.
So don't ask them to do what computers should do.
They shouldn't spend a minute finetuning or selecting forecasting models.
Let an algorithm do this. It will be faster and more reliable.
I don't clean outliers, and so should you;
If you have outliers,
- Invest more time in (automated) data cleaning and processing.
- Include more business drivers in your forecasting engine (promotions, shortages, price changes)
- If you really have some exceptional periods (covid), bypass them - don't correct them.
Finally, if your demand planners worry (or track) about current and future shortages, it means that you are forecasting sales and not demand. This will result in a vic...
So don't ask them to do what computers should do.
They shouldn't spend a minute finetuning or selecting forecasting models.
Let an algorithm do this. It will be faster and more reliable.
I don't clean outliers, and so should you;
If you have outliers,
- Invest more time in (automated) data cleaning and processing.
- Include more business drivers in your forecasting engine (promotions, shortages, price changes)
- If you really have some exceptional periods (covid), bypass them - don't correct them.
Finally, if your demand planners worry (or track) about current and future shortages, it means that you are forecasting sales and not demand. This will result in a vic...
Просмотров: 47
Видео
Don't hire demand planners to review forecasting models
Просмотров 9314 дней назад
Don't hire demand planners to review forecasting models. Data scientists should create a fully automated forecasting engine. If you need a human to manually check it, it means it's not bulletproof, and it should be improved. Demand planners should care about the quality of the data fed into the forecasting engine (historical transactions, promotions, historical inventory levels, etc.) and about...
How to make an efficient demand planning process
Просмотров 33921 день назад
If you want to learn more about demand and inventory planning, I predict you'll enjoy my mailing list: mailchi.mp/supchains.com/newsletter. My 3 books: www.amazon.com/stores/Nicolas-Vandeput/author/B07KL86HMV
Which product should demand planners review first?
Просмотров 5821 день назад
Have you heard something from your clients or marketing colleagues, or is there a new product launch? If so, you should enrich the forecast for these products/clients. I call this "insight-driven enrichments": instead of going through a list of products from most to least important, gather business insights and only enrich forecasts when you know something specific. It's unlikely you can add va...
Planners only have a 50% chance of improving forecasts.
Просмотров 56Месяц назад
Planners only have a 50% chance of improving a forecast when modifying it. Academics say. That's really low, unfortunately. This means that the overall added value is low as they have a 50/50 chance of improving or worsening the underlying forecast. So, what can we do to improve this ratio? 1️⃣ Track Forecast Value Added. Without this, you are in the dark. You know what they say: if you can't m...
Don't freeze forecasts!
Просмотров 80Месяц назад
Never freeze forecasts! It's not because you have a frozen production plan that you should freeze your forecasts. In short, forecasts are just pieces of information. Freezing them is like refusing to take your temperature today because your doctor's appointment is tomorrow. It makes no sense. Let's say your production plan is frozen for the next three weeks. Is there any value in updating your ...
Don't use ABC XYZ to set inventory targets
Просмотров 121Месяц назад
Don't use ABC XYZ to set inventory targets. (Why would you ever need to do this anyway?) To set the right inventory policy, you need to take into account, - Service level objectives - Forecasting accuracy - Supply reliability - Lead times - Seasonality There is no way you can segment your products into nine meaningful classes that cover the cases above. But also, why would you ever need to do t...
Demand planners shouldn't care about supply and sales.
Просмотров 138Месяц назад
Demand planners shouldn't care about supply and sales. They should forecast demand. Not sales. And should *not* consider supply. If you forecast supply-constrained sales, you will end up in a vicious circle: 1️⃣ Let's imagine you are currently facing a shortage of one of your products. 2️⃣ Your best sales forecast (at least short term) is 0. No one will buy your product because you don't have a...
3 Things you need to be great at demand planning
Просмотров 2452 месяца назад
Do you want your supply chain to be great at demand planning? Here's what you need, 1️⃣ Forecast Value Added. Without this, you are literally in the dark: you have no clue if your team (or software) is adding any value. (And no, tracking forecasting accuracy and bias isn't enough.) 2️⃣ See Forecasting as an Information Game. What do you know? How does this impact forecasts? Here are the basics:...
How to Review Forecasts?
Просмотров 2 тыс.6 месяцев назад
50% of planners’ forecast reviews worsen accuracy, academics say. How can we do better? In this webinar, Professor Emeritus Robert Fildes and I introduce you to the best (and worst!) practices for demand planners to review forecasts. We first look at 15 years of historical research to understand what works and what does not. Then, I explain how demand planners can (and will) still add value to ...
How Machine Learning impacts Demand Planning
Просмотров 1,6 тыс.6 месяцев назад
In this keynote I explain how to achieve demand planning excellence using machine learning. I also discuss why planners often fail to add value to their demand forecasts and what we can do about it.
Machine Learning for Demand Planning
Просмотров 4,3 тыс.6 месяцев назад
Machine Learning for Demand Planning
50% of planners fail to add value to their forecasts
Просмотров 6887 месяцев назад
50% of planners fail to add value to their forecasts
Why MAPE is the Worst Forecast KPI.
Просмотров 3,5 тыс.10 месяцев назад
Why MAPE is the Worst Forecast KPI.
Forecast Value Added: Concept and Case Studies
Просмотров 2,3 тыс.11 месяцев назад
Forecast Value Added: Concept and Case Studies
Demand Forecasting Best Practices - Chapter 1
Просмотров 615Год назад
Demand Forecasting Best Practices - Chapter 1
Outlier Management - Detection and Correction
Просмотров 3,3 тыс.Год назад
Outlier Management - Detection and Correction
Data Science & Machine Learning for Demand Forecasting
Просмотров 13 тыс.Год назад
Data Science & Machine Learning for Demand Forecasting
How to improve forecasting for fun and profit
Просмотров 9522 года назад
How to improve forecasting for fun and profit
Forecasting for Supply Chain Planning
Просмотров 2,6 тыс.2 года назад
Forecasting for Supply Chain Planning
How to deal with unpredictable lead times?
Просмотров 1,3 тыс.2 года назад
How to deal with unpredictable lead times?
Best Practices for Inventory Management
Просмотров 8 тыс.2 года назад
Best Practices for Inventory Management
Best Practices for Demand Forecasting
Просмотров 17 тыс.2 года назад
Best Practices for Demand Forecasting
Machine Learning for Demand Forecasting in Supply Chains
Просмотров 19 тыс.2 года назад
Machine Learning for Demand Forecasting in Supply Chains
where is link to competition ?
www.datasource.ai/en/home/data-science-competitions-for-startups/vn1-forecasting-accuracy-challenge-phase-1/description There you go!
@@nicolasvandeput-SupChains competition is on hold till sep12
meantime may you share link to another data set to download for demand prediction ML task? As you wrote : Why you should never forecast sales On the other hand, sales are constrained by (lack of) supply. So data set to predict demand but for real live situation when sales are constrained by (lack of) supply
@@sndrstpnv8419 you can also always look at the M5 forecasting competition.
@@nicolasvandeput-SupChains M5 is not for case when sales are constrained by (lack of) supply?
may you share link to your data science completion with 20k prize as you announced ?
www.datasource.ai/en/home/data-science-competitions-for-startups/vn1-forecasting-accuracy-challenge-phase-1/description Sure, there you go. We had to move the starting date to 12th of September
What is the paper you reference in here (at 52:00) related to changing forecasts for minimal amounts? And tradeoffs for time?
very nice explanation. Is geometric mean being used for evaluation as well? You could get more "typical" error compared to average.
is there any way to connect you?
LinkedIn is best: www.linkedin.com/in/vandeputnicolas/
Great speech! Where do you recommend people learn more about ML as it pertains to forecasting?
Hello, if you want to learn how to make your own models: www.amazon.com/Data-Science-Supply-Chain-Forecasting/dp/3110671107 (It's a step by step approach starting from 0, so don't worry if you are not an expert today.) If you want to understand how ML impacts demand planning and how your teams should work with it. www.amazon.com/Demand-Forecasting-Practices-Nicolas-Vandeput/dp/1633438090
Nicholas that’s a good advice! I saw that you wrote an article on How to Forecast Intermediate Demand on medium but it’s behind the paywall! 😢
Hi I am an S&OP manager based in Pakistan, I wanted to know how can I get access to your book? also I have been working to create baseline forecast in my organization but since there are no historic demand driver details available its very difficult to generate baseline forecast, any mathematical approach that I can use to atleast begin with forecasting for longer period of months?
Hi Nicholas, when it comes to feature engineering for future covariates, which features are a must according to you? The only future feature I've been able to implement is the lag features, however one is then constrained by the lowest lag feature, i.e if you have lag 7 day feature, you can't predict further than 7 days into the future. What other future variables are there that one would know in the future, apart from holidays and company specific things like marketing costs, promotions etc?
🎯 Key points for quick navigation: 00:29 *📊 Forecast Value Added (FVA) assesses how different teams contribute to improving or worsening forecasting accuracy.* 02:05 *🔄 Demand planning processes typically involve automated baseline forecasts adjusted by teams to enhance accuracy.* 04:19 *🎯 FVA aims to ensure forecast accuracy improvements without excessive time spent on adjustments.* 05:02 *📉 FVA framework tracks how each team's adjustments impact forecast accuracy positively or negatively.* 08:16 *📈 Comparing forecasts to benchmarks like moving averages helps assess the added value of forecasting models.* 11:16 *🎯 Setting accuracy improvement targets relative to baseline performance can be more effective than absolute accuracy targets.* 14:41 *💰 Evaluating forecast errors based on value helps prioritize improvements on high-value products over low-value ones.* 19:28 *🌐 Forecasting across various time horizons (short, medium, long-term) supports strategic supply chain decisions.* 23:09 *📊 Forecast Value Added (FVA) helps identify SKU-level performance, guiding decisions on where to focus and where improvements areneeded.* 23:38 *🔄 FVA encourages a positive feedback loop by comparing market performance against statistical baselines, fostering model improvements.* 24:31 *🌐 Different forecast horizons (short-term vs. mid-to-long-term) require varying model strengths, prompting discussions on model integration.* 25:12 *🤝 Collaborative discussions using FVA help align marketing and finance teams by highlighting where judgmental adjustments add value.* 25:49 *📉 Separating positive and negative adjustments in FVA reveals insights into which adjustments enhance or diminish forecast accuracy.* 27:01 *🎯 Forecasting supports supply chain decisions, aiding in manufacturing and procurement planning crucial for business operations.* 46:55 *🌍 Different countries and industries may require tailored risk management strategies in pharmaceutical production to ensure patient needs are met without compromise.* 47:22 *🤝 Collaborative relationships between planning teams and sales are crucial for mitigating forecast overrides, emphasizing education on supply chain dynamics and outcomes.* 48:27 *📊 Presenting a range of forecast possibilities enhances decision-making by providing stakeholders with more nuanced insights and flexibility.* 49:20 *💡 Implementing statistical engines requires effective change management strategies to shift from manual to automated forecasting processes, emphasizing education and gradual adoption.* 51:12 *💼 For small to medium-sized businesses, affordability and implementation time of forecasting tools can pose significant challenges despite their potential benefits.* 54:04 *📈 Transitioning from manual to automated forecasting involves proving benefits through accuracy metrics and building confidence in system outputs to foster acceptance among demand planners.* Made with HARPA AI
Thanks for the vides Nicolas! I have read all your books - what a fresh take! Please make a video for niche demand planners and inventory controllers to which I belong. That is MRO spare parts inventory which is fraught with intermittent demand and skewed probability distributions. If you ever revise your books 'Data Science for Supply Chain Forecast' and 'Inventory Optimization' please include these topics. I read your article on Croston Method in towards data science and it was very well written with a practitioner's perspective. Whenever possible please make a video on demand forecasting and inventory control for spare parts. Thanks!
Second this! Well said 👏
Love it! 🙏 Thanks for sharing Nicolas! So once we have a great forecast using these three steps, how does one implement the inventory optimization element? I’d be curious to hear your top 3 on the IO portion. Of course, your IO book goes into this quite well already!
Hi Nicolas, it is a very explanatory webinar about outliers. I have a question. Do we need to apply outlier detection process based on train data, or whole data (train+test)? I hope my question is clear.
I would try not to do any statistical outlier detection. I would invest more in data cleaning. If you remove outliers from the test set, you are somehow overfitting - so I would not do it.
Beautiful and intelligent Forecasting session
Muy buen vídeo; la explicación quedó clara con el ejemplo desarrollado en Excel
This is really nice.
Hello dear , I want to congratulate you for the content and the skills that you teach people . I have a question : the forcast ,like you describe it , is applicable for the retail demand planning and the MTO strategy , that's right .Because I don't think that this kind of forcasting is relevent in an MTO industry where the demand is not stable .
Thank you for your webinar. I have a question regarding outliers. I am conducting a serum biomarker research (medical research) consisting 50 patients vs. 50 controls. I have 3 cases having non-detectable values (above the detection level) in the same group. This group is already have higher levels than the other one. I do not want to remove those cases and lose the data. Which strategy should I use ? Should I imputate them with the mean value of the relevant group? or Should I enter the measured highest value/s instead of undetectable ones ? or else ? Thank you in advanced.
Amazing! Thank you for sharing Nicolas!!
Hi Nicolas, to calculate the bias in case of many unpredictable new products introduction and phase out, the latter are not considered because the time series are not available in the forecast period while the former are considered and demand is greatly overestimated, as the 2 cases do not compensate, the overall bias on the product portfolio is always positive. How do you recommend managing this case? Should I consider as a forecast error also the forecast 0 on NPI even if their time series were not available at the time of the forecast?
Hello, this is quite a complicated case. You could compute bias in two different flavors, with and without NPIs. The idea is that you don't want to bring the message that bias is close to 0% whereas obviously, you missed 10% of NPIs. But the responsibility for these NPIs might lie with another team.
Thank you Nicolas. Actually what happens is that if I include NPIs I get an unbiased forecast overall because NPIs compensate unpredictable phase out products, while if I don’t include NPIs I get a positive bias (globally on the product portfolio). Maybe global metrics in this case are not meaningful and I should look at the distribution of Bias/MAE of product time series.
Hi Nicholas, you explained it very well. Could we access the PowerPoint presentation you used?
Thanks Nicolas for amazing content.. how can i join your live sessions?
You can register here to be informed of future webinars: mailchi.mp/supchains.com/newsletter
Awesome session! I'm curious, how would we forecast zeroes? lets say we have inventory for such items but they do no sell at particular time period may be.
use static rules based on competitor price
please make a detailed video on forecasting slow moving intermittent demanded items!
Good summary! I used this to explain to my colleagues
Please which certification would you recommend for a demand planner?
I was waiting for it, Thanks
Do you have the github python code available for these?
No, but I share them in books available here: www.amazon.com/stores/Nicolas-Vandeput/author/B07KL86HMV?ref=sr_ntt_srch_lnk_2
Hello Nicolas, what do you think of training a ML model using as input in addition to past demand also the previous month ML forecast enriched by sales? For example, to predict December 2023 demand (M+2) I would use as input features summarizing historical demand + the forecast submitted last month so in september for december (which was M+3) possibly enriched by sales. So if sales enrich a forecast because they are aware of future trends, the following month this information will be captured by the model.
Hi Nicolas, thank you for sharing this. I have a question for you on forecasting error metrics, I know you don’t like MAPE and I agree, but what do you think of WAPE i.e. sum of SKU (actual - forecast) divided by sum of all SKU actuals ? I think it’s a quite good accuracy metric and also easy to explain to business stakeholders as it is a percentage.
Hello, indeed that's the one I like to use. I call it MAE%. Don't forget to look at it in combination with bias.
Created one year ago, and is still relevant today! Watched the whole thing and probably will watch it again. Thanks Nicolas! Love this!
any code or tutorial?
I share the code in my books: www.amazon.com/stores/Nicolas-Vandeput/author/B07KL86HMV?ref=sr_ntt_srch_lnk_2
BonjourMr , pourquoi vous ne fairiez pas une formation en ligne (payante bien entendu) où vous enseignez le demand planing d'une façon théorique et pratique avec des cas réels , des exercices de prévisions sur excel .....? Nous sommes une génération qui n'aime pas trop recevoir l'information en lisons (même si je ne doute pas que le contenu du livre est pertinant )
Je vais probablement publier une vidéo à l'occasion cette année à propos des KPIs.
Thank you for posting these webinars. Even with all the Q&A on shortages I'm still confused on figuring out unconstrained demand. On your slide you say to bypass it, but in your book it says to censor it. Are you meaning the same thing for both the slide and book? Also, on the slide in this webinar, that's also book, it looks like you're using a default value for demand, which looks to be the last demand value before the shortage for the duration of the shortage. Is that what you use? Your book mentions forecasting techniques that might help estimate unconstrained demand, but I can't find any examples. Can you share those techniques? Do you use machine learning techniques, or an equation? Thanks!
I usually don't use equations to clean shortages. Nowadays, I just censor them. nicolas-vandeput.medium.com/forecasting-demand-despite-shortages-fee899120c08
What great content! I just finished reading demand forecasting best practices and found this video in the footnotes. Very cool, several learnings, thank you!
How do you capture a demand for a manufacturer in a b2b setting. As orders are been placed and stored in the erp system. Do you use the quantity of order placed as the are intermittent in nature.
Track historical orders (and even preorders) and censor periods with shortages: nicolas-vandeput.medium.com/forecasting-demand-despite-shortages-fee899120c08
what if my lead time is different from material to material?
Great content !!! Thank you for sharing.
Hi there I have a question, exist a tool like COV for trends or seasonality.
Please make video on forecasting intermittent time series data. I tried croston, tsb etc but results are pretty bad.I have only 8 months data . Will you please suggest some methods.
I just have a question on the first one; why do we focus til' M5; why not further and then how further do we forecast? Like a dynamic programming problem; we can keep focusing til' the end of the planning horizon to assess what's a good position at M5, M4,... right?
Hello Pras, You have two problems: - On which horizon should you focus your forecasting effort - On which horizon should you focus your planning effort For both, if you use models (anything automated), you could do as much as possible. But if you need human resources (to do the baseline or enrich a model), you'll have to focus on what's the most important. You only have limited time/resources.
Would be interesting to get you opinion on MAPE to compare multiple forecasts (or to use as performance metric for to evaluate multiple time series), since RMSE, MAE are not suitable to do so.
Hello David, long story short: MAPE is never a good idea. MAE is fine for comparing different products.
@@nicolasvandeput-SupChains how would you use MAE to compare different product on different scale? Since the MAE does reflect the different scale and is therefore hard to use for comparison or as an aggregated metric of multiple products.
I just noticed your definition of MAE might be different to the standard one (en.wikipedia.org/wiki/Mean_absolute_error) since you represent it as a percentage value. Would be great if you can clarify this.
@@davidtiefenthaler7753 MAE scales perfectly if you have many products. %MAE doesn't scale across different product. it's all explained here: - www.manning.com/books/demand-forecasting-best-practices - towardsdatascience.com/forecast-kpi-rmse-mae-mape-bias-cdc5703d242d In general, no KPIs are perfect. Especially when looking at broad portfolio.
please make a video on forecasting of slow moving intermittent and lumpy demand patterns such as those encountered in MRO parts demands. How to use Croston method to forecast mean demand and its variance/std dev and then how datascience forecasting can help in such cases.
Croston is not a good idea: towardsdatascience.com/croston-forecast-model-for-intermittent-demand-360287a17f5f
@@nicolasvandeput-SupChains yes I noticed it did poorly with my dataset. TSB and ADIDA did better.
@@nwabuezeprecious457 How do they compare to a moving average 12 months?
@@nicolasvandeput-SupChains TSB performed better. but this due to the volatility of my demand data. but if one has a relatively stable demand then a 12 months rolling forecast is suitable. But as you know @Nicolas whatever the technique for forecasting you intend to use will really depend on your case study. Thanks Nicolas for responding reading your book currently.
Great presentation.
Thank you!
Excellent
Hello Nicolas , Kindly i need your email to contact you
/watch?v=kkpFZA1sVSA&t=376s
In the next edition please include the forecasting and inventory control models for intermittent items especially spare parts. For example MCROST and (S,S-1) and how to implement them in Python.
Do you have any Kaggle notebook or GitHub repo for this work?
Thanks for this webinar. You did a great job giving a high level explanation on the ML concept. I was expecting to have comparaisons between models. Data Scientists should focus more on showing the results of experimentation then advertising the concept. I still don’t know of I should invest money and time to build a POC