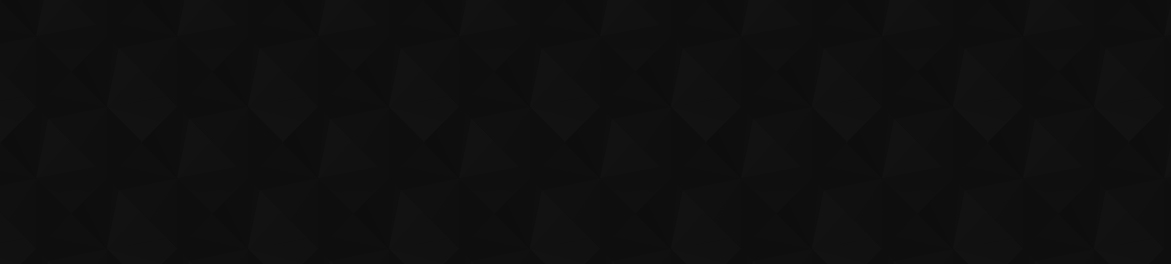
- Видео 54
- Просмотров 21 350
USC Probability and Statistics Seminar
США
Добавлен 30 апр 2021
Richard Y. Zhang: Rank Overparameterization and Global Optimality Certification ... (UIUC)
Numerous important problems across applied statistics reduce into nonconvex estimation / optimization over a low-rank matrix. In principle, these can be reliably solved to global optimality via convex relaxation, but the computational costs can become prohibitive on a large scale. In practice, it is much more common to optimize over the low-rank matrices directly, as in the Burer-Monteiro approach, but their nonconvexity can cause failure by getting stuck at a spurious local minimum. For safety-critical societal applications, such as the operation and planning of an electricity grid, our inability to reliably achieve global optimality can have significant real-world consequences.
In the fi...
In the fi...
Просмотров: 13
Видео
Weixin Yao: New Regression Model: Modal Regression (UC Riverside)
Просмотров 27314 дней назад
Built on the ideas of mean and quantile, mean regression and quantile regression are extensively investigated and popularly used to model the relationship between a dependent variable Y and covariates x. However, the research about the regression model built on the mode is rather limited. In this talk, we propose a new regression tool, named modal regression, that aims to find the most probable...
Morris Yau: Are Neural Networks Optimal Approximation Algorithms (MIT)
Просмотров 2,1 тыс.7 месяцев назад
In this talk, we discuss the power of neural networks to compute solutions to NP-hard optimization problems focusing on the class of constraint satisfaction problems (boolean sat, Sudoku, etc.). We find there is a graph neural network architecture (OptGNN) that captures the optimal approximation algorithm for constrainst satisfaction, up to complexity theoretic assumptions via tools in semidefi...
Mahdi Soltanolkotabi: Foundations for feature learning via gradient descent (USC)
Просмотров 4848 месяцев назад
One of the key mysteries in modern learning is that a variety of models such as deep neural networks when trained via (stochastic) gradient descent can extract useful features and learn high quality representations directly from data simultaneously with fitting the labels. This feature learning capability is also at the forefront of the recent success of a variety of contemporary paradigms such...
Larry Goldstein: Classical and Free Zero Bias for Infinite Divisibility (USC)
Просмотров 14210 месяцев назад
dornsife.usc.edu/probability-and-statistics-seminars/wp-content/uploads/sites/244/2023/11/abstract.pdf
Yuhua Zhu: Continuous-in-time Limit for Bandits (UCSD)
Просмотров 19711 месяцев назад
In this talk, I will build the connection between Hamilton-Jacobi-Bellman equations and the multi-armed bandit (MAB) problems. MAB is a widely used paradigm for studying the exploration-exploitation trade-off in sequential decision making under uncertainty. This is the first work that establishes this connection in a general setting. I will present an efficient algorithm for solving MAB problem...
Yier Lin: The atypical growth in a random interface (U Chicago)
Просмотров 21511 месяцев назад
Random interface growth is all around us: tumors, bacterial colonies, infections, and propagating flame fronts. The KPZ equation is a stochastic PDE central to a class of random growth phenomena. In this talk, I will explain how to combine tools from probability, partial differential equations, and integrable systems to understand the behavior of the KPZ equation when it exhibits unusual growth...
Vatsal Sharan: Sample Amplification: Increasing Dataset Size even when Learning is Impossible (USC)
Просмотров 13011 месяцев назад
Is learning a distribution always necessary for generating new samples from the distribution? To study this, we introduce the problem of “sample amplification”: given n independent draws from an unknown distribution, D, to what extent is it possible to output a set of m datapoints that are indistinguishable from m i.i.d. draws from D (where m is larger than n)? Curiously, we show that nontrivia...
Mladen Kolar: Latent multimodal functional graphical model estimation (U Chicago)
Просмотров 17911 месяцев назад
oint multimodal functional data acquisition, where data multiple modes of functional data are measured from the same subject simultaneously, has emerged as an exciting modern approach enabled by recent engineering breakthroughs in the neurological and biological sciences. One prominent motivation for acquiring such data is to enable new discoveries of the underlying connectivity by combining si...
Omer Tamuz: On the origin of the Boltzmann distribution (Caltech)
Просмотров 334Год назад
The Boltzmann distribution is used in statistical mechanics to describe the distribution of states in systems with a given temperature. We give a novel characterization of this distribution as the unique one satisfying independence for uncoupled systems. The theorem boils down to a statement about symmetries of the convolution semigroup of finitely supported probability measures on the natural ...
Jasper Lee: Mean and Location Estimation with Optimal Constants (UW Madison)
Просмотров 615Год назад
Mean and location estimation are two of the most fundamental problems in statistics. How do we most accurately estimate the mean of a distribution, given i.i.d. samples? What if we additionally know the shape of the distribution, and we only need to estimate its translation/location? Both problems have well-established asymptotic theories: use the sample mean for mean estimation and maximum lik...
Lenny Fukshansky: Geometric constructions for sparse integer signal recovery (Claremont McKenna)
Просмотров 314Год назад
We investigate the problem of constructing m x d integer matrices with small entries and d large comparing to m so that for all vectors x in Z^d with at most s ≤ m nonzero coordinates the image vector Ax is not 0. Such constructions allow for robust recovery of the original vector x from its image Ax. This problem is motivated by the compressed sensing paradigm and has numerous potential applic...
Andrew Lowy: Private Stochastic Optimization with Large Worst-Case Lipschitz Parameter... (USC)
Просмотров 970Год назад
We study differentially private (DP) stochastic optimization (SO) with loss functions whose worst-case Lipschitz parameter over all data points may be extremely large. To date, the vast majority of work on DP SO assumes that the loss is uniformly Lipschitz continuous over data (i.e. stochastic gradients are uniformly bounded over all data points). While this assumption is convenient, it often l...
Robi Bhattacharjee: Online k-means Clustering on Arbitrary Data Streams (UCSD)
Просмотров 618Год назад
We consider online k-means clustering where each new point is assigned to the nearest cluster center, after which the algorithm may update its centers. The loss incurred is the sum of squared distances from new points to their assigned cluster centers. The goal over a data stream X is to achieve loss that is a constant factor of L(X,OPTk), the best possible loss using k fixed points in hindsigh...
Jorge Vargas: Spectral stability under real random absolutely continuous perturbations (Caltech)
Просмотров 687Год назад
In this talk I will discuss the following random matrix phenomenon (relevant in the design of numerical linear algebra algorithms): if one adds independent (tiny) random variables to the entries of an arbitrary deterministic matrix A, with high probability, the resulting matrix A′ will have (relatively) stable eigenvalues and eigenvectors. More concretely, I will explain the key ideas behind ob...
Mateo Díaz: Clustering a mixture of Gaussians with unknown covariance (Caltech)
Просмотров 497Год назад
Mateo Díaz: Clustering a mixture of Gaussians with unknown covariance (Caltech)
Ying Tan: A Generalized Kyle-Back Insider Trading Model with Dynamic Information (USC)
Просмотров 763Год назад
Ying Tan: A Generalized Kyle-Back Insider Trading Model with Dynamic Information (USC)
Mei Yin: Probabilistic parking functions (Denver)
Просмотров 540Год назад
Mei Yin: Probabilistic parking functions (Denver)
Xin Sun: Two types of integrability in Liouville quantum gravity (UPenn)
Просмотров 874Год назад
Xin Sun: Two types of integrability in Liouville quantum gravity (UPenn)
Matan Harel: On delocalization of planar integer-valued height functions ... (Northeastern)
Просмотров 781Год назад
Matan Harel: On delocalization of planar integer-valued height functions ... (Northeastern)
Vadim Gorin: Lozenge tilings via the dynamic loop equation (Berkeley)
Просмотров 706Год назад
Vadim Gorin: Lozenge tilings via the dynamic loop equation (Berkeley)
Melih Iseri: Set Valued HJB Equations (USC)
Просмотров 692Год назад
Melih Iseri: Set Valued HJB Equations (USC)
Çağın Ararat: Set-valued martingales and backward stochastic differential equations (Bilkent)
Просмотров 569Год назад
Çağın Ararat: Set-valued martingales and backward stochastic differential equations (Bilkent)
Guang Cheng: A Statistical Journey through Trustworthy AI (UCLA)
Просмотров 947Год назад
Guang Cheng: A Statistical Journey through Trustworthy AI (UCLA)
Simo Ndaoud: Adaptive robustness and sub-Gaussian deviations in sparse linear regression... (ESSEC)
Просмотров 4812 года назад
Simo Ndaoud: Adaptive robustness and sub-Gaussian deviations in sparse linear regression... (ESSEC)
Lisa Sauermann: Anticoncentration in Ramsey graphs and a proof of the Erdös-McKay Conjecture (MIT)
Просмотров 8672 года назад
Lisa Sauermann: Anticoncentration in Ramsey graphs and a proof of the Erdös-McKay Conjecture (MIT)
Tom Hutchcroft: Critical behaviour in hierarchical percolation (Caltech)
Просмотров 3382 года назад
Tom Hutchcroft: Critical behaviour in hierarchical percolation (Caltech)
Ken Alexander: Disjointness of geodesics for first passage percolation in the plane (USC)
Просмотров 2582 года назад
Ken Alexander: Disjointness of geodesics for first passage percolation in the plane (USC)
Daniel Kane: Ak Testing of Distributions (UCSD)
Просмотров 3902 года назад
Daniel Kane: Ak Testing of Distributions (UCSD)
Robert Webber: Randomized low-rank approximation with higher accuracy and reduced costs (Caltech)
Просмотров 3912 года назад
Robert Webber: Randomized low-rank approximation with higher accuracy and reduced costs (Caltech)
No paper references?
Is there any repository of code so we can try this method?
I would also like to know
The speaker mentioned it would eventually be uploaded publicly!@@xlad_
We have now published the source code. Unfortunately, RUclips does not allow links, so I will instead tell you that the GitHub repository is penlu/bespoke-gnn4do.
@joshuascholar3220 @xlad_ We have now published the source code, but we are unable to say where due to RUclips's community guidelines and automatic comment filtering.
Let's see if this goes. Repo name is "bespoke-gnn4do" and username is "penlu".
audio starts at 10:00
☹️ "PromoSM"
Audio starts at 2:40
The camera focuses after 17:00
More details on set valued calculus: ruclips.net/video/A9Qt9Ho1Gg8/видео.htmlsi=aidhIDzFA1MPsgk1&t=273
i could of listened to it but er you know er just can er say er anything er without er :) just a tip.