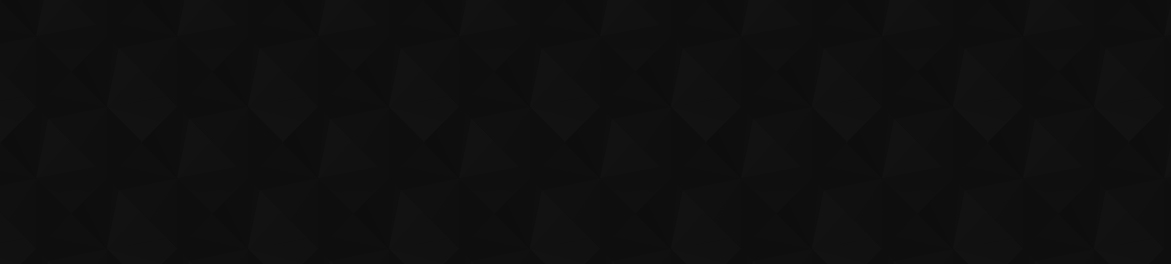
- Видео 220
- Просмотров 76 398
Hany Farid, Professor at UC Berkeley
США
Добавлен 17 авг 2018
Deepfakes
The technology that can distort and manipulate digital media is developing at break-neck speeds, and it is imperative that the technology that can detect such alterations develop just as quickly. The field of photo forensics seeks to restore some trust to photography.
At their foundation, photo forensic techniques rely on understanding and modeling the imaging pipeline, from the interaction of light with the physical 3-D world, the refraction of light as it passes through the camera lenses, the transformation of light to electrical signals in the camera sensor, and, finally, the conversion of electrical signals into a digital image file. This set of lectures focuses on the first part of t...
At their foundation, photo forensic techniques rely on understanding and modeling the imaging pipeline, from the interaction of light with the physical 3-D world, the refraction of light as it passes through the camera lenses, the transformation of light to electrical signals in the camera sensor, and, finally, the conversion of electrical signals into a digital image file. This set of lectures focuses on the first part of t...
Просмотров: 668
Видео
Ballistic Motion
Просмотров 1509 месяцев назад
The technology that can distort and manipulate digital media is developing at break-neck speeds, and it is imperative that the technology that can detect such alterations develop just as quickly. The field of photo forensics seeks to restore some trust to photography. At their foundation, photo forensic techniques rely on understanding and modeling the imaging pipeline, from the interaction of ...
3D Modelling
Просмотров 1349 месяцев назад
The technology that can distort and manipulate digital media is developing at break-neck speeds, and it is imperative that the technology that can detect such alterations develop just as quickly. The field of photo forensics seeks to restore some trust to photography. At their foundation, photo forensic techniques rely on understanding and modeling the imaging pipeline, from the interaction of ...
Specularities
Просмотров 699 месяцев назад
The technology that can distort and manipulate digital media is developing at break-neck speeds, and it is imperative that the technology that can detect such alterations develop just as quickly. The field of photo forensics seeks to restore some trust to photography. At their foundation, photo forensic techniques rely on understanding and modeling the imaging pipeline, from the interaction of ...
Lighting
Просмотров 899 месяцев назад
The technology that can distort and manipulate digital media is developing at break-neck speeds, and it is imperative that the technology that can detect such alterations develop just as quickly. The field of photo forensics seeks to restore some trust to photography. At their foundation, photo forensic techniques rely on understanding and modeling the imaging pipeline, from the interaction of ...
Human Abilities and Limits
Просмотров 939 месяцев назад
The technology that can distort and manipulate digital media is developing at break-neck speeds, and it is imperative that the technology that can detect such alterations develop just as quickly. The field of photo forensics seeks to restore some trust to photography. At their foundation, photo forensic techniques rely on understanding and modeling the imaging pipeline, from the interaction of ...
Lens Flare
Просмотров 829 месяцев назад
The technology that can distort and manipulate digital media is developing at break-neck speeds, and it is imperative that the technology that can detect such alterations develop just as quickly. The field of photo forensics seeks to restore some trust to photography. At their foundation, photo forensic techniques rely on understanding and modeling the imaging pipeline, from the interaction of ...
Shadows
Просмотров 1319 месяцев назад
The technology that can distort and manipulate digital media is developing at break-neck speeds, and it is imperative that the technology that can detect such alterations develop just as quickly. The field of photo forensics seeks to restore some trust to photography. At their foundation, photo forensic techniques rely on understanding and modeling the imaging pipeline, from the interaction of ...
Vanishing Points and Lines
Просмотров 3029 месяцев назад
The technology that can distort and manipulate digital media is developing at break-neck speeds, and it is imperative that the technology that can detect such alterations develop just as quickly. The field of photo forensics seeks to restore some trust to photography. At their foundation, photo forensic techniques rely on understanding and modeling the imaging pipeline, from the interaction of ...
Reflections
Просмотров 1329 месяцев назад
The technology that can distort and manipulate digital media is developing at break-neck speeds, and it is imperative that the technology that can detect such alterations develop just as quickly. The field of photo forensics seeks to restore some trust to photography. At their foundation, photo forensic techniques rely on understanding and modeling the imaging pipeline, from the interaction of ...
Introduction
Просмотров 1,1 тыс.9 месяцев назад
The technology that can distort and manipulate digital media is developing at break-neck speeds, and it is imperative that the technology that can detect such alterations develop just as quickly. The field of photo forensics seeks to restore some trust to photography. At their foundation, photo forensic techniques rely on understanding and modeling the imaging pipeline, from the interaction of ...
Closing: parting thoughts
Просмотров 126Год назад
Learn Computer Vision: These lectures introduce the theoretical and practical aspects of computer vision from the basics of the image formation process in digital cameras, through basic image processing, space/frequency representations, and techniques for image analysis, recognition, and understanding.
Image understanding: unsupervised learning: tSNE: implementation
Просмотров 183Год назад
Learn Computer Vision: These lectures introduce the theoretical and practical aspects of computer vision from the basics of the image formation process in digital cameras, through basic image processing, space/frequency representations, and techniques for image analysis, recognition, and understanding.
Image understanding: unsupervised learning: t-distributed stochastic neighbor embedding (tSNE)
Просмотров 158Год назад
Learn Computer Vision: These lectures introduce the theoretical and practical aspects of computer vision from the basics of the image formation process in digital cameras, through basic image processing, space/frequency representations, and techniques for image analysis, recognition, and understanding.
Image understanding: unsupervised learning: principal component analysis (PCA): eigenfaces
Просмотров 120Год назад
Learn Computer Vision: These lectures introduce the theoretical and practical aspects of computer vision from the basics of the image formation process in digital cameras, through basic image processing, space/frequency representations, and techniques for image analysis, recognition, and understanding.
Image understanding: unsupervised learning: principal component analysis (PCA): computation
Просмотров 86Год назад
Image understanding: unsupervised learning: principal component analysis (PCA): computation
Image understanding: unsupervised learning: principal component analysis (PCA): implementation
Просмотров 98Год назад
Image understanding: unsupervised learning: principal component analysis (PCA): implementation
Image understanding: unsupervised learning: principal component analysis (PCA): eigenvectors
Просмотров 105Год назад
Image understanding: unsupervised learning: principal component analysis (PCA): eigenvectors
Image understanding: unsupervised learning: principal component analysis (PCA): covariance matrix
Просмотров 150Год назад
Image understanding: unsupervised learning: principal component analysis (PCA): covariance matrix
Image understanding: unsupervised learning: principal component analysis (PCA): canonical basis
Просмотров 107Год назад
Image understanding: unsupervised learning: principal component analysis (PCA): canonical basis
Image understanding: unsupervised learning: expectation/maximization: EM implementation
Просмотров 55Год назад
Image understanding: unsupervised learning: expectation/maximization: EM implementation
Image understanding: unsupervised learning: expectation/maximization: M-step
Просмотров 54Год назад
Image understanding: unsupervised learning: expectation/maximization: M-step
Image understanding: unsupervised learning: expectation/maximization: E-step
Просмотров 67Год назад
Image understanding: unsupervised learning: expectation/maximization: E-step
Image understanding: unsupervised learning: expectation/maximization: EM
Просмотров 74Год назад
Image understanding: unsupervised learning: expectation/maximization: EM
Image understanding: unsupervised learning: clustering: k-means implementation
Просмотров 88Год назад
Image understanding: unsupervised learning: clustering: k-means implementation
Image understanding: unsupervised learning: clustering: k-means
Просмотров 129Год назад
Image understanding: unsupervised learning: clustering: k-means
Image understanding: supervised learning: classification: ANN: convolutional
Просмотров 93Год назад
Image understanding: supervised learning: classification: ANN: convolutional
Image understanding: supervised learning: classification: ANN: backpropagation
Просмотров 73Год назад
Image understanding: supervised learning: classification: ANN: backpropagation
Image understanding: supervised learning: classification: ANN: universal approximation theorem
Просмотров 63Год назад
Image understanding: supervised learning: classification: ANN: universal approximation theorem
Image understanding: supervised learning: classification: artificial neural networks: xor + hidden
Просмотров 60Год назад
Image understanding: supervised learning: classification: artificial neural networks: xor hidden
Thank you, very clear and interesting explanation.
This was awesome. Thank you from a Computer Vision student in the UK!
1:54 -- what last section?
See here for the full syllabus: farid.berkeley.edu/learnComputerVision/
Fantastic, thank you!!!
Holy shit why doesnt this have more views
can anyone give me the correct step for the laplacian pyramid?? 1. Take the image fi from stage i. 2. filter the image fi with a low pass filter and thus create the image Li 3. Downsample the image li and thus create the image fi+1 4. Calculate the difference image hi=fi-li 5. cache hi 6. Consolidate all images hi 7. Repeat the above step n times.
Here is some Python code for computing a Laplacian pyramid: # Laplacian pyramid import matplotlib.pyplot as plt import numpy as np import cv2 from scipy.signal import sepfir2d im = plt.imread( 'mandrill.png' ) # load image h = [1/16,4/16,6/16,4/16,1/16]; # blur filter N = 4 # number of pyramid levels # Gaussian pyramid G = [] G.append(im) # first pyramid level for k in range(1,N): # pyramid levels im2 = np.zeros( im.shape ) for z in range(3): im2[:,:,z] = sepfir2d( im[:,:,z], h, h ) # blur each color channel im2 = im2[0:-1:2, 0:-1:2,:] # down-sample im = im2 G.append(im2) # Laplacian pyramid L = [] for k in range(0,N-1): # pyramid levels l1 = G[k] l2 = G[k+1] l2 = cv2.resize(l2, (0,0), fx=2, fy=2) # up-sample D = l1 - l2 D = D - np.min(D) # scale in [0,1] D = D / np.max(D) # for display purposes L.append(D) L.append(G[N-1]) # display pyramid fig, ax = plt.subplots(nrows=1, ncols=N, figsize=(15, 7), dpi=72, sharex=True, sharey=True) for k in range(N-1,-1,-1): ax[k].imshow(L[k])
@@hanyfarid5019 Thank you so much for the reply would you please give me the correct algorithm step for this one?
such a wonderful explanation! thank you!
Can I get a lecture on SIFT?
I don't have lecture on SIFT, but this is a lecture on the related HOG features: ruclips.net/video/RaaGoB8XnxM/видео.html
I love how easy this is to understand. I guess there are multiple ways to accomplish a CE. In my text, its explained using XOR with NOR circuits to accomplish the same thing. Or, am I misunderstanding?
You are correct. There are several different ways to create a CE circuit. The way I show is perhaps the most straight-forward, but definitely not the most efficient.
P=NP : ^ )
Much much better than the channels with millions of subscribers. I love it...❤❤
Why didnt I find this channel earlier...
Dear Professor Hany Farid, Thank you for sharing this fantastic course on image filtering! I find your explanations to be extremely clear and engaging. I have a quick question regarding linear time-invariant functions at around the 3:29 and 3:57 minute marks. The formulas shown use h[-3-k] and h[-4-k]. I was wondering if this might be a typo, as I would have expected h[-1-k] and h[0-k] to be used instead, or if I am missing something.
A viewer noticed that there is a bug at the 03:43 mark (nice catch) but I accidentally deleted their comment (sorry viewer). The code at this mark should read: M1z@M1z.T (not M1z@M2z.T)
Obvious typo on line 9 at 3:43: should be M1z@M1z.T + ...
Good catch. The code at this mark should read: M1z@M1z.T (not M1z@M2z.T)
Thanks Professor for your knowledge
Very clear explanation, thank you
This is the most comprehensive CV course I have ever seen. I was always looking for something like this but I always found general datascience courses. Thank you so much.
you is a legend
amazing point of view for explaining number of the features we want for a model won't change the cost function.
Wonderful lesson! Thank you
Amazing! Thank you
thank you for these lecture series, they are interesting
Can't be simpler. Best explanation
Thank you
Wow the legend that you are. Thank you!!!
Wow such a clear and concise video! And you make sure to review material that student should know! Amazing
Awesome explanation!! As on all the videos. Thanks for the uploads! 🙌🙌
Thank professor its really helpful
Thank you for this real world problem exercise ! I guess any rigid motion may be explained as a "combination" that ?
Why didn't anyone comment on a really good explanation?
how do you generalize this model to a dataset that "don't pass through the origin" ? Do we need to "center" the data before using it ?
Yes, the data can be centered (i.e., zero meaned) by subtracting the mean of each component.
@@hanyfarid5019 thanks, really appreciated. your video are really cool !!
Loved it!!
Great work sir. I never see any one in you tube covers in this much depth❤😊your students will be lucky to have you
Thank you professor for your effort this was really well explained, looking forward to learn more from this playlist
at 6:21, may be the summation of impulses representation is not right if the unit impulse signal is considered to be centered.
In this formulation, we assume the unit impulse falls on an integer sampling lattice.
You are great at teaching. ❤
VV Good
awesome video thanks!
how comes this channel has only 500 subs very valuable stuff here
Hi Prof. Farid, At 3:50, since the "*" notation stands for convolution, shouldn't the filter be [1, -1]? Assuming right is the positive x direction The following is some python output: a = [0, 1, 0, 0] b = scipy.signal.convolve(a, [1, -1], mode='same') print(a) print(b) [0, 1, 0, 0] [ 0 1 -1 0] Similarly in 13:27, I think the d filter should be reversed.
This depends on the specific implementation of convolution. Some implementations yield the results you see here in which the filter is flipped, while others don't flip the filter. Your code here is a good way to determine how to specify your filter values.
Your videos are great! Thank you for sharing!
What is the difference between grayscale intensity vs luminance from YCbCr?
Thank you@@hanyfarid5019 . I experimented both, turned out they have very similar variance, some of them are even exact match, so I kept only grayscale for easier explanation
This was a great video thank you
Thank you!
Thanks from Türkiye.
Hi Prof. Farid: I was having great trouble of understanding what sepfir2d do, since intuitively we are talking about convolving but here we are using a different function(sepfir2d). Since skimage API doesn't provide much details, I have to try and guess for a few hours to really understand the the linkage between the two concept. I felt it would be better to show the code using signal.convolve2d, then show the equivalent but more computational efficient form would really help future student. The following python code is not a bulletproof test but enough to show my understanding is correct : import numpy as np from scipy import signal import random PRE_FILTER = np.array( [0.030320, 0.249724, 0.439911, 0.249724, 0.030320], dtype=np.float32, ) FIRST_ORDER_DERIVATIVE_FILTER = np.array( [-0.104550, -0.292315, 0.000000, 0.292315, 0.104550], dtype=np.float32, ) KERNEL_FIRST_ORDER_DERIVATIVE_FILTER = np.zeros((5, 5), dtype=np.float32) KERNEL_FIRST_ORDER_DERIVATIVE_FILTER[2, :] = FIRST_ORDER_DERIVATIVE_FILTER KERNEL_PRE_FILTER = np.zeros((5, 5), dtype=np.float32) KERNEL_PRE_FILTER[:, 2] = PRE_FILTER def test_sepfir2d_convolve2d(test_count: int): for _ in range(test_count): random_image = np.array(random.sample(range(0, 255), 100)).reshape(10, 10) sep_gradient_x = signal.sepfir2d(random_image, FIRST_ORDER_DERIVATIVE_FILTER, PRE_FILTER) conv_gradient_x_row = signal.convolve2d(random_image, KERNEL_FIRST_ORDER_DERIVATIVE_FILTER, mode="same", boundary="symm") conv_gradient_x = signal.convolve2d(conv_gradient_x_row, KERNEL_PRE_FILTER, mode="same", boundary="symm") print(f'sepfir2d and convole2d are equal: {np.array_equal(sep_gradient_x, conv_gradient_x)}') test_sepfir2d_convolve2d(50) returns all true for 50 random tested image
Thanks for sharing this code.
These videos are so good and clear, how come no one leave a comment?
your videos are fantastic... I'm happy I found your channel ❤