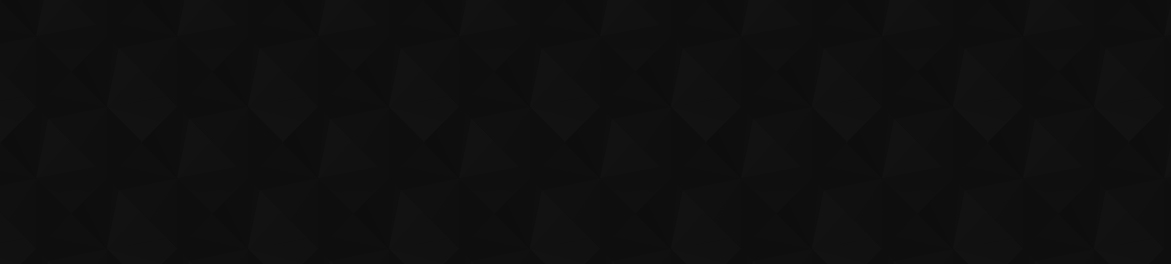
- Видео 98
- Просмотров 177 544
Statistics Made Easy by Stat-Ease
США
Добавлен 25 ноя 2015
Statistics Made Easy®...this channel is devoted to helping you use design of experiments (DOE) to succeed in making significant improvements to your products and processes. Software products referenced are Stat-Ease 360 and Design-Expert software published by Stat-Ease, Inc. For more information, go to www.statease.com.
Robustness Creating process flexibility from the start
This recorded webinar upgrades robustness considerations previously discussed for characterization via factorial design to the optimization phase of process or product development. It lays out response surface methods (RSM) that identify regions within the design space that not only meet all response specifications, but also minimizes variation caused by wandering factor settings and extraneous noise factors.
View the slides: cdn.statease.com/media/public/documents/Robustness_Creating_Process_Flexibility.pdf
Richard's other robustness webinar: ruclips.net/video/hADNyURhXPw/видео.html
More on robust design and POE: ruclips.net/video/jawFFzDxqmA/видео.html
RSM Saves the Circuit Board: ruclips.n...
View the slides: cdn.statease.com/media/public/documents/Robustness_Creating_Process_Flexibility.pdf
Richard's other robustness webinar: ruclips.net/video/hADNyURhXPw/видео.html
More on robust design and POE: ruclips.net/video/jawFFzDxqmA/видео.html
RSM Saves the Circuit Board: ruclips.n...
Просмотров: 97
Видео
DOE for on target results with minimal variation
Просмотров 127Месяц назад
This presentation makes the case for modeling both mean and standard deviation to achieve on target results with minimal variation. It demonstrates benefits via examples where experimenters took advantage of making multiple measurements for every run in their design. Newcomers to statistical design of experiments (DOE) often overlook this opportunity to achieve more robust operating conditions....
Robustness studies: If anything can go wrong, prevent it!
Просмотров 1212 месяца назад
The goal of robustness studies is to demonstrate that our processes will be successful upon implementation in the field when they are exposed to anticipated noise factors. Watch this webinar to learn about the assumptions and underlying concepts that need to be understood when setting out to conduct a robustness study, and what kind of design you need to fit each situation. View the slides: cdn...
Advanced Mixture DOE for Formulators
Просмотров 2352 месяца назад
Building up from the popular Mixture DOE Crash Course, this webinar explains how formulators can: - Create an experiment design that combines mixture components with process factors - Include categorical factors, such as various types of ingredients - Deal with hard to change variables via a split-plot design Stat-Ease software makes these advanced experiments easy to design and it provides exc...
Optimal experiment designs that combine mixture, process and categorical inputs
Просмотров 3544 месяца назад
This presentation provides practical aspects for combining mixture, process and categorical variables into one optimal experiment-design. It covers an innovative way to minimize runs via a specialized “KCV” model (named for the inventors: Kowalski, Cornell and Vining). Furthermore, the talk details options for structuring combined designs into a split plot, which makes them far more do-able. Al...
Strategies for Sequential Experimentation
Просмотров 3085 месяцев назад
DOE is often presented as a “one shot” approach. It may be more efficient to divide the experiment into smaller pieces, thus expending resources in a more adaptive manner. This sequential approach becomes especially suitable when beginning with very little information about the process, for example, when scaling up a new product. It allows for better definition of the design space, adaption to ...
Exploiting Statistical Experiment Design to Accelerate Pharmaceutical R&D
Просмотров 4676 месяцев назад
Learn how multicomponent and multifactor design-of-experiment (DOE) tools empower experimenters to quickly converge on the quality by design (QbD) “sweet” spot-ingredient and factor settings that meet all specifications at minimal cost. All examples come directly from pharmaceutical industries. Engineers, chemists and scientists working on drug development will do well by attending this briefin...
DOE Crash Course for Experimenters
Просмотров 4 тыс.8 месяцев назад
Learn how design of experiments (DOE) makes research efficient and effective. A quick factorial design demo illustrates how simple it can be to use DOE for accelerating R&D. Discover how to find your vital few factors and reveal breakthrough interactions. View the slides here: statease.com/documents/482/2024-02_Crash_Course_in_DOE_RSW_v2.pdf Disponible en español: ruclips.net/video/NxTPGgHKjWM/...
Layout Tool Mini-Demo
Просмотров 3728 месяцев назад
The layouts in Design-Expert and Stat-Ease 360 are customizable depending on your needs. This quick demo shows off how to arrange the windows in your analysis & optimization screens to meet your needs. Like, subscribe, and ring the bell to get notified when we post new videos! More quick tips: ruclips.net/p/PL2jZXHPuEgAQPP3PM-3HE_AMEblVtRJjh Subscribe to our newsletters to be notified about upc...
Know the SCOR for Multifactor Strategy of Experimentation
Просмотров 5129 месяцев назад
By way of example, this presentation lays out a strategy for design of experiments (DOE) that provides maximum efficiency and effectiveness for development of a robust process. It provides a sure path for converging on the ‘sweet spot’-the most desirable combination of process parameters and product attributes. Whether you are new or experienced at doing DOE, this talk is for you (and your orga...
Curso Intensivo en Diseño de Experimentos
Просмотров 24510 месяцев назад
Mejore su habilidad en diseños de experimentos (DOE) con esta herramienta de prueba multifactorial en esta sesión informativa. Una demostración breve demuestra porque DOE es tan efectivo en acelerar R&D (investigación y desarrollo) y optimización de procesos y productos. En este seminario descubrirá como DOE encuentra los factores vitales más importantes y revela interacciones innovadoras. Avai...
New User Intro to Design Expert® Software
Просмотров 10 тыс.10 месяцев назад
Learn how to use Design-Expert DOE software for factorial, response surface and mixture designs. This brief introduction demonstrates the basics you need to get started. Download the slides: cdn.statease.com/media/public/documents/New-User_Webinar_rev_1.5.pdf Ready to learn more? Try these videos: ruclips.net/video/y9TeN1kJdyk/видео.html ruclips.net/video/rqCpy_2SNKs/видео.html Learn about our ...
Keys to Analyzing a Response Surface Design
Просмотров 4,2 тыс.10 месяцев назад
Optimize your products and processes with accurate prediction models. In this webinar, learn how to get the most out of your response surface method (RSM) design by following a few key analysis steps. See how automated model-reduction tools build simpler models that predict more precisely. Then discover how diagnostics confirm your model’s validity. Finally, learn how key statistics like lack o...
Keys to Building the Perfect Response Surface Design
Просмотров 1,8 тыс.10 месяцев назад
Response surface methods (RSM) provide a quick path to the peak of process performance. This webinar presents an array of RSM designs to choose from - central composite, Box-Behnken and optimal (custom). Learn when each design excels. Also find out how to handle categoric factors, discrete numeric levels and complex constraints involving multiple factors. Discover how to set up the right RSM de...
The Latest & Greatest in Design-Expert® and Stat-Ease® 360
Просмотров 866Год назад
After decades of continuous development, Design-Expert® software (DX) leads the field for making design of experiments (DOE) easy for scientists and engineers. In response to many requests from loyal users to expand our statistical toolkit, we are proud to now produce Stat-Ease® 360 (SE360). With DX at its core, SE360 adds advanced features for statistics and engineering, as well as sophisticat...
DoE in the field of nuclear waste management
Просмотров 260Год назад
DoE in the field of nuclear waste management
What Teaching and the Practice of DOE Teaches Us About Deriving Meaning from Any Data
Просмотров 186Год назад
What Teaching and the Practice of DOE Teaches Us About Deriving Meaning from Any Data
Application of DOE in the Development of an Alcoholic Beverage
Просмотров 366Год назад
Application of DOE in the Development of an Alcoholic Beverage
Masterful experiment delivers delectable chocolate chip cookies
Просмотров 304Год назад
Masterful experiment delivers delectable chocolate chip cookies
Selecting a Most Useful Predictive Model
Просмотров 2,7 тыс.Год назад
Selecting a Most Useful Predictive Model
Dive into Diagnostics to Uncover Data Discrepancies
Просмотров 630Год назад
Dive into Diagnostics to Uncover Data Discrepancies
Taking Advantage of New DOE Tools for Random Block Effects
Просмотров 796Год назад
Taking Advantage of New DOE Tools for Random Block Effects
Design Evaluation: Statistical Tools for Assessing Your Design Quality
Просмотров 1,6 тыс.Год назад
Design Evaluation: Statistical Tools for Assessing Your Design Quality
Deploying DOE to Accelerate R&D for Biotech
Просмотров 414Год назад
Deploying DOE to Accelerate R&D for Biotech
Do’s & Don’ts for Screening Process Factors
Просмотров 939Год назад
Do’s & Don’ts for Screening Process Factors
Very good explanation
Will be nice to have the transfer equation for variation (POE) ? There are plans to have on the future versions ? Because if we have this equation we can predicted the variation in several regions of the process and predict quality levels
That's a great idea! We get lots of feature requests, so we'll add this to the list. Make sure to sign up for our software email newsletter to be informed of new features as they're released.
@@StatisticsMadeEasybyStatEase hope to see in the next release
Does the software works in arm based laptops, because I’m going to buy a Surface laptop 7 with arm chip.
It should! We haven't specifically tested it, but Windows says that our application (which is x64-based) would be able to run on the latest Surface.
Thanks, very helpful!
Very good
Can you share the ppt ?
It's linked in the description.
hai.. The **Predicted R²** of 0.3685 is not as close to the **Adjusted R²** of 0.7063 as one might normally expect; i.e. the difference is more than 0.2. This may indicate a large block effect or a possible problem with your model and/or data. Things to consider are model reduction, response transformation, outliers, etc. All empirical models should be tested by doing confirmation runs. The example above was from the software. the software stated if the difference is greater than 0.2, there is problem with data or etc. However, as u said, greater than 0.2 is better. I also found in one journal stated that greater is better. Can you explain regarding this matter? Thank you so much.
Hi! It looks like there's some confusion here. What the software is talking about is the *difference* between the Predicted and Adjusted R^2 values: you want them to agree with each other, so the difference of the values is less than 0.2. In other words, the Predicted R^2 in this example should be closer to the Adjusted R^2 value of 0.7063. This is not the same as looking at a single R^2 value, where you do want the value to simply be as high as possible.
Thank you a lot for your illustration, really, outliers are a big problem! But also, I think there are problems that can come from multicollinearity and need to be solved.
hai, if the means of my experimental values are all already in the PI interval, should I do the two sample t-test also to see either there is significant diff. between the experimental and predicted values? Thank you.
No, the prediction interval is the correct interval to use; that the observed mean falls within the prediction interval is the test.
Thanks very much
Is there any way to know if rsm is done right by looking at 3D graph
Yes, by seeing if the surface bisects the points and fits them within normal variation (does not exhibit a significant lack of fit). To see what I mean, open the tutorial data Chemical Conversion (Analyzed), go to the Model Graphs, 3D Surface and click through the Jump to run points. This is an example of RSM done right. : ) You can access the Stat-Ease tutorials at www.statease.com/docs/latest/tutorials
God Bless you, sir
Such an insightful presentation
How do I download design expert? I have sought for it for months, can’t find how to download it! 🥺
You can request a trial from www.statease.com/trial, and buy it if you think it will suit your needs.
Based on your experience do you think mixture designs can be effective in the development of concrete mixes. Just a random question? 58:00:01
Absolutely. Check out the Crash Course for Mixture Designs video: ruclips.net/video/yWDDCeQ0JVA/видео.html
@@StatisticsMadeEasybyStatEase I will definitely check it out.
Nice presentation on optimal designs. I especially like your concluding statement, "Always choose a design that fits the problem."
Thank you very much!
Hi, I am working on an experiment using Response Surface Methodology (Design of Experiment). I use StatEase software for the same. During analysis the software shows that CUBIC model for my data is Aliased. However, the cubic model is significant (p<0.05) and has insignificant lack of fit (p > 0.05). Moreover R2 value is also very good 0.985. Can I use this model for prediction of optimized conditions, although the model is aliased, but statistically significant? Please give me a quick response, Thanks
Hi! This is a great question for our experts. Please send it to them here: statease.com/about-us/contact/contact-support/
We did not see the presets in the simulation part of the video since pre-simulated files were used. Would you care to add that in your next video?
Going any deeper into this is beyond the scope of an introductory webinar, but you're welcome to explore the other videos here on RUclips or check out our eLearning on the Stat-Ease Academy: www.statease.com/training/academy
O problema todo é que o dual do dual é o primal.
Could you kindly add the sample files you use for the examples to the description in the video for easy access
We don't offer those files publicly, but you're welcome to download the slides to make it easier to do the data entry yourself.
What does it mean when a coefficient of a component in optimal design model equation is negative while the response only takes positive value? My understanding is that the coefficient is the response value of the component when it is pure (100%), so it can't be negative.
Sorry it took so long to see this! Please send this inquiry to our StatHelp group: www.statease.com/about-us/contact/contact-support/
when entering the responses, should i enter them as average with +- SD or as pure data? & thank you
Just enter the raw data for that specific experiment.
Please, can you explain how we can get the value of Response 1
We used some previously saved data for this tutorial. When you design your own experiments, you'll need to run the experiment and enter your observed responses.
Absolutely great content. Thank you for uploading this!
This is an excellent lecture on a very important concept in DOE. I have 2 questions. 1. Adding additional model points can increase the desired FDS. What if there are some practical constraints in not adding additional model points like time, or budget? What trade-offs we need to make to ensure we get the same precision? 2. What if I don't have an historical data of the std deviation? Let's say the process is a new one. Which std dev should I use? Should a separate ANOVA study be conducted and then use this std dev?
If you do not want to add more runs, then finding ways to decrease the noise in the system, or increasing the acceptable "d" will both change the signal to noise ratio. A larger S/N ratio will increase the FDS calculation.
Can 3D graphs be created for categorial data? Thank you
The 3D graph for categorical factors is a set of bar graphs, with the height of each bar representing the predicted response value for that combination.
Thank you
I'm going to have mixture component of A, B and C. A+B+C = 100% and the ratio of A/B must maintain at 0.5. How do I set the constrain since I can't enter both lower and upper limit with the same value.
This imposes an extra equality constraint. The level of A is dependent on the level of B. Now there are only 2 components that vary, but all 3 are involved in the total 100%. Short answer - there is not an easy way to get this done.
Do you have version to have Weibull Regression and DOE?
There will be a Python interface that allows Weibull regression in the 2023 release of Stat-Ease 360.
Great video!
Hi there, Can I use old data from somebody else and increase responses, and the design expert redesign another experiment for me so i do less experiment?
In theory yes, you can import the old data in the software and use Design Augmentation to add more runs to fit a higher order model. In practice, there are many questions starting with - is the process still running the same as it did previously? Are you measuring the same way? Was the previous data from a designed experiment or was it historical data from the system? This is really a practical experimentation question. You can try and it may be successful, or it may not work well and you would get better information from a new experiment.
Thnak you for this amazing video! How can I choose the number of samples I have to run for each trial?
Great question - this is a power/sample size calculation, based on the change in the response that you want to detect and the current standard deviation of the response. If you have a current license of Design-Expert or Stat-Ease 360, you are welcome to email more details to stathelp@statease.com. If you don't want to do the calculations, then often using a sample size of 4-5 per run, and then entering the average of those runs as the response, will give sufficient information.
28:30 I would suggest using 'Strength' as the metric, not Strength Reduction. Because the end customer is interested in strength. 34:10 Seems that component B is not adding value to the mixture from the response Strength perspective 37:04 Seems that component B is also not adding value with respect to the response Zeta Potential 57:00 Did you decide to leave the AB term in the model (despite p value > 0,10) because it felt not comfortable for you to tell the organization that component B can be removed (and that the company has been using it for no good reason)?
For strength reduction the BD interaction was highly significant. As mentioned, there were a number of other responses. B may have been involved in other interactions in those cases. Subject matter knowledge would always drive these decisions.
Thanks for sharing
Thank you for the great video. What is the significance, if any, of the standard mean error in design evaluation?
I'm not sure exactly which statistic you are referring to, but any standard error is an estimate of variation for that statistic.
@@StatisticsMadeEasybyStatEase I meant the one below FDS value at 51:34
@@tafadzwankhoma1533 That is the calculated standard error after the experimenter inputs their "d" and "s". Continuing forward to 52:21, Martin is demonstrating changing these values, thus changing the signal/noise ratio. The blue horizontal line is the calculated value (the standard error). The values should be your desired precision, relative to the normal process variation. Refer to this video for more discussion: ruclips.net/video/uY7OqM9awxE/видео.html
the triangle at 11:34, is it generated in DX or you plotted it in another App/Software separately.
The graphic originated in DX and was modified for the presentation.
👏
Thank you for the recording. What is the relationship between Design Expert and StatEase 360. Can one use Stat-Ease 360 to completely design and analyse experimental data without Design Expert or StatEase is a build up on Design Expert
Stat-Ease 360 includes Design-Expert software, and also has higher-level features such as linkage to Python and additional regression options.
Hello sir... Thank you for your great video.