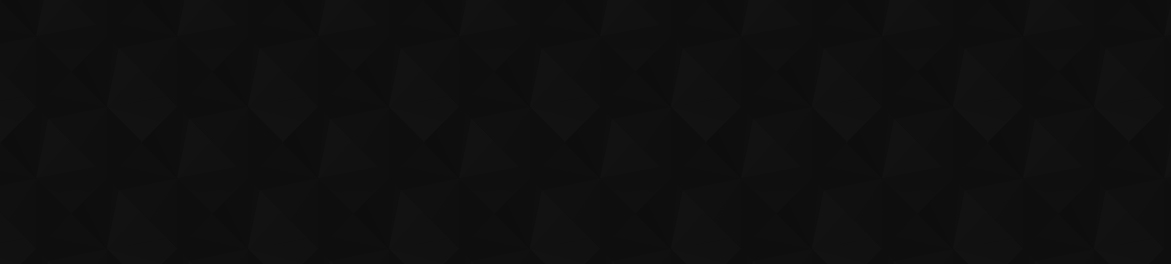
- Видео 173
- Просмотров 708 305
Elvis Saravia
Великобритания
Добавлен 5 сен 2013
Weekly tutorials, paper summaries, and technical walkthroughs on the most important AI and LLM developments.
Join my school to learn how to build with AI: dair-ai.thinkific.com/
Business inquiries: elvissaravia@dair.ai
Join my school to learn how to build with AI: dair-ai.thinkific.com/
Business inquiries: elvissaravia@dair.ai
DeepSeek releases new reasoning LLM | DeepSeek R1 Lite Preview | **IMPRESSIVE** (Tested)
Learn how to build with AI in my new courses here: dair-ai.thinkific.com/
Use code BLACKFRIDAY to get a 35% off. The offer expires 11/29.
---
Overview of DeepSeek R1 Lite Preview
#ai #coding #artificialintelligence
Use code BLACKFRIDAY to get a 35% off. The offer expires 11/29.
---
Overview of DeepSeek R1 Lite Preview
#ai #coding #artificialintelligence
Просмотров: 2 576
Видео
Introducing Forge Reasoning APIs | The future of building with agents?
Просмотров 90916 часов назад
Learn how to build with AI in my new courses here: dair-ai.thinkific.com/ Use code RUclips20 to get an extra 20% off. Limited-time offer! Newsletter: nlp.elvissaravia.com/ X: x.com/omarsar0 LinkedIn: www.linkedin.com/in/omarsar/ Overview of the Nous Forge Reasoning APIs and a discussion of the future of building with reasoning capabilities and agents. #ai #artificialintelligence #tech
ChatGPT can now look at your coding tools! 👀
Просмотров 1,1 тыс.19 часов назад
Learn how to build with AI in my new courses here: dair-ai.thinkific.com/ Use code RUclips20 to get an extra 20% off. Limited-time offer! Newsletter: nlp.elvissaravia.com/ X: x.com/omarsar0 LinkedIn: www.linkedin.com/in/omarsar/ ChatGPT Desktop can now look at coding apps and improve code generation. #ai #openai #tech
Qwen2-5-Coder is the NEW best open code LLM!
Просмотров 1,4 тыс.День назад
Learn how to build with AI in my new courses here: dair-ai.thinkific.com/ Use code RUclips20 to get an extra 20% off. Limited-time offer! Qwen2-5-Coder is the best open code LLM! Newsletter: nlp.elvissaravia.com/ X: x.com/omarsar0 LinkedIn: www.linkedin.com/in/omarsar/ #ai #tech #chatgpt
Claude PDF Analyzer | Ridiculously GOOD! (Tested)
Просмотров 2,2 тыс.14 дней назад
Learn how to build with AI in my new courses here: dair-ai.thinkific.com/ Use code RUclips20 to get an extra 20% off. Limited-time offer! Testing Claude PDF Analyzer #ai #chatgpt #artificialintelligence
Claude 3.5 Haiku | 4x the price of previous model | TOO EXPENSIVE!?
Просмотров 1,5 тыс.14 дней назад
Learn how to build with AI in my new courses here: dair-ai.thinkific.com/ Use code RUclips20 to get an extra 20% off. Limited-time offer! Overview of Claude 3.5 Haiku. More here: www.anthropic.com/claude/haiku #ai #programming #artificialintelligence
How to evaluate using LLM-as-a-Judge (Tutorial)
Просмотров 36414 дней назад
For code and full tutorial enroll in our FREE course on LLM Evaluation: www.comet.com/site/llm-course/ #ai #chatgpt #artificialintelligence
OpenAI introduces ChatGPT Search! (Watch this before trying it)
Просмотров 2,8 тыс.21 день назад
Learn how to build with AI in my new courses here: dair-ai.thinkific.com/ Use code RUclips20 to get an extra 20% off. Limited-time offer! OpenAI introduces ChatGPT Search. We take it for a spin and discuss on the good and bad. #ai #chatgpt #tech
Claude 3.5 Sonnet now available in GitHub Copilot!
Просмотров 11 тыс.21 день назад
Claude 3.5 Sonnet now available in GitHub Copilot!
Building a ReAct AI Agent (Tutorial)
Просмотров 1,4 тыс.21 день назад
Building a ReAct AI Agent (Tutorial)
Google ships custom audio overviews! (NotebookLM Updates)
Просмотров 2,2 тыс.Месяц назад
Google ships custom audio overviews! (NotebookLM Updates)
Mistral AI introduces Ministral 3B & 8B | Most capable small language models!?
Просмотров 1,7 тыс.Месяц назад
Mistral AI introduces Ministral 3B & 8B | Most capable small language models!?
Evaluating Fairness in ChatGPT (Research)
Просмотров 422Месяц назад
Evaluating Fairness in ChatGPT (Research)
Introduction to NotebookLM: About the Instructor
Просмотров 491Месяц назад
Introduction to NotebookLM: About the Instructor
Introduction to NotebookLM: Conclusion
Просмотров 178Месяц назад
Introduction to NotebookLM: Conclusion
Introduction to NotebookLM: Improving an AI Lecture
Просмотров 264Месяц назад
Introduction to NotebookLM: Improving an AI Lecture
Introduction to NotebookLM: Improving Technical Guide
Просмотров 216Месяц назад
Introduction to NotebookLM: Improving Technical Guide
Introduction to NotebookLM: Generating Social Media Posts
Просмотров 253Месяц назад
Introduction to NotebookLM: Generating Social Media Posts
Introduction to NotebookLM: Formatting Content
Просмотров 217Месяц назад
Introduction to NotebookLM: Formatting Content
Introduction to NotebookLM: Summarizing a Newsletter
Просмотров 243Месяц назад
Introduction to NotebookLM: Summarizing a Newsletter
Introduction to NotebookLM: Analyzing PDFs with NotebookLM
Просмотров 614Месяц назад
Introduction to NotebookLM: Analyzing PDFs with NotebookLM
Introduction to NotebookLM: Getting Started with NotebookLM
Просмотров 624Месяц назад
Introduction to NotebookLM: Getting Started with NotebookLM
Introduction to NotebookLM: Course Objectives
Просмотров 401Месяц назад
Introduction to NotebookLM: Course Objectives
Introduction to NotebookLM: Introduction
Просмотров 881Месяц назад
Introduction to NotebookLM: Introduction
OpenAI Introduces Canvas (Overview & Demo)
Просмотров 3,8 тыс.Месяц назад
OpenAI Introduces Canvas (Overview & Demo)
NotebookLM now supports chatting with YouTube videos
Просмотров 4,9 тыс.Месяц назад
NotebookLM now supports chatting with RUclips videos
Meta releases Llama 3.2 | NEW small & vision models are here!
Просмотров 2,6 тыс.Месяц назад
Meta releases Llama 3.2 | NEW small & vision models are here!
Google releases Gemini-1.5-Pro-002 | FASTER, BETTER, CHEAPER!
Просмотров 6 тыс.Месяц назад
Google releases Gemini-1.5-Pro-002 | FASTER, BETTER, CHEAPER!
Hey Elvis! This is a great overview of the paper. We work on structured generation at .txt and took the claims in this paper very seriously. We recently took a good look at the data and code behind this paper and published a response. We found several issues in the paper regarding the claim that "structured generation constraints significantly impact LLM performance across various tasks". Here's a quick summary of our findings: 1. The paper itself finds that structured generation has superior performance on a number of classification tasks (thanks for calling this out in the video!). 2. The prompts used for unstructured (NL) generation are markedly different than the ones used for structured generation, so the comparisons are not apples-to-apples to begin with. 3. The structured generation prompts do not provide the model with adequate information to solve the task, this leads to particularly poor performance for the ‘json-mode’ examples. 4. The real meat of the paper is actually about parsing the results of one LLM with a second LLM. The authors refer to this as the “Perfect Text Parser”. 5. The paper confuses structured generation with JSON-mode, although independent runs of these evals show that “JSON-mode” yields better results than unstructured generation. If you'd allow it I'd love to post a link to the response, but don't want spam your comments section! 😂
Thanks for the comment. By all means, please post your report. I would to take a look myself. These things change and I think it’s good work you all are doing to go deeper into the topic and present your findings.
How much is this the underlying model being really good and how much of it is a really well done chain of thought prompt?
I believe this is really the challenge here. The chain-of-thought prompt optimization and the model capabilities are both influencing the results. To what extent? We really don't know - I think that is an interesting area of research. From my experience CoT is useful to many class of problems that require logic, planning, and self-reflection but it's not even clear if we are getting all of these capabilities in the CoT steps. Something to analyze more closely so this is why I am excited about the possibility to get access to the raw thinking steps and not just a summary of it like what OpenAI o1 unfortunately does.
GitHub have api very well documentade.
I am using DeepSeek 2.5 since a while. In many tasks this LLM beats ChatGpt, Google Gemini and Claude Sonnet. It is slower but I like the usefulness of the responses. I assume that at DeepSeek smart people are developing useful models which are working well with less advanced compute. Banning modern NPU/GPU chips from China represents a clear incentive to develop LLMs which are running with less NPU/GPU requirements. That will pay off soon for the Chinese AI developers while US providers like OpenAI and Microsoft will be drowning in their compute cost.
You are right. These models are really good. I think it’s good competition and their approach to the thinking steps is an interesting direction. But we definitely need alternative and models that do more unique and efficient inference.
Not sure about the API version, but the chat version sucks.
Could you elaborate on what you mean by it sucks. I am curious to know as I think the dev experience is definitely lacking.
@@elvissaravia It very quickly loses track of its task, and begins looping quite easily. It can definitely tackle difficult problems, but once you go into real-world problems as opposed to benchmark type prompts, it quickly starts repeating the previous response instead of factoring in a reiteration of the problem statement/parameters that were already given to it before. And then it just gets stuck or loses track entirely. For comparison, o1-mini tackles the same tasks flawlessly without losing track. I do think it might be a token issue, though. Since up to the point of where it starts looping/losing track it gives very good responses, and when you hone in on subsections it can elaborate quite rigorously and accurately.
@ I see. Yeah I observed too that it’s repeating stuff unnecessarily even when it got the right response for some tasks. That’s not great! They need to do a lot more work on the quality of those thinking steps. Thanks for sharing, good stuff!
Can you make a video comparing it with another model in a environment
Yes, great suggestion. I am working on that along with a few more tests included.
@elvissaravia same project, same prompts, different model b2b. Let the games begin
Same deepSeek, same problem, giving different result
Peter has 5 candles that are all the same length. He lights them all at the same time. After a while, he blows out the candles one after the other. Which of the five candles was the first one he has blown out? Here is a figure of the five candles after they have been blown out. The number of = represents the length of the candle. Respond with the label of the candle that has been blown out first by Peter. 1. ==== 2. ======= 3. ======== 4. = 5. ==
Okay, let's see. I have this problem about Peter and his candles. He has five candles, all the same length, and he lights them all at the same time. After some time, he blows them out one after the other, and then there's a figure showing the lengths of the candles after they've been blown out. The lengths are represented by the number of equal signs. So, candle 1 has four equals, candle 2 has seven, candle 3 has eight, candle 4 has one, and candle 5 has two. First, I need to understand what's going on here. All candles are the same length initially, and they're all lit at the same time. That means they should be burning at the same rate, assuming they're made of the same material and are in the same environment. But then Peter blows them out one after the other, and we see their lengths after that. I think the key here is to figure out which candle was blown out first based on how much it has burned. Since they all started at the same length and were burning at the same rate, the one that was blown out first should have burned the least, right? Because it had less time to burn before being extinguished. Looking at the lengths after being blown out: candle 3 is the longest with eight equals, then candle 2 with seven, candle 5 with two, candle 1 with four, and candle 4 with one equal sign. Wait, actually, candle 4 is the shortest with just one equal sign, and candle 3 is the longest. So, if the one that was blown out first has the least time to burn, it should be the one with the least length burned off. But I need to think carefully here. The number of equals represents the length remaining, not the length burned. Wait a minute, if the equals represent the length remaining, then the candle with more equals means it has burned less, and the one with fewer equals has burned more. So, the candle that was blown out first would have had the least time to burn, meaning it has the most length remaining, which is candle 3 with eight equals. But, let's double-check. If all candles were lit at the same time and then blown out one after the other, the first one blown out would have burned for the shortest time, so it should have the most remaining length. Conversely, the last one blown out would have burned the longest, having the least remaining length. Given that, candle 3 has the most remaining length, so it should be the first one blown out. But, there's a twist. The problem says "Which of the five candles was the first one he has blown out?" and provides the lengths after being blown out. Wait, perhaps I should consider the burning rates differently. Maybe the candles have different burning rates even if they are the same length? But the problem says they are all the same length and presumably made of the same material, so they should burn at the same rate. Alternatively, maybe the number of equals represents the length burned, not the remaining length. But that doesn't make sense because it says "the number of = represents the length of the candle." Hmm, this is confusing. Let me read the problem again. "Here is a figure of the five candles after they have been blown out. The number of = represents the length of the candle." So, the equals represent the length remaining after being blown out. Therefore, the candle with the most equals has the most length remaining, meaning it burned the least, which would be the first one blown out. Therefore, candle 3 with eight equals is the first one blown out. But let's think about this again. If all candles are burning at the same rate, and the first one is blown out, it has less time to burn, so more length remains. The next one is blown out a bit later, so it has burned a little more, and so on. So, the order from most remaining length to least remaining length should correspond to the order in which they were blown out, from first to last. So, candle 3: eight equals (most remaining, first blown out) Then candle 2: seven equals Then candle 1: four equals Then candle 5: two equals Then candle 4: one equal (least remaining, last blown out) Yes, that seems logical. But perhaps there is another way to look at it. Maybe considering the time each candle was burning before being blown out. Assuming they all started at the same time, and were blown out one after the other, the first blown out has the shortest burning time, hence most remaining length. Yes, that aligns with what I thought earlier. So, the answer should be candle 3. But the problem is presented in a way that might be trying to trick me, so I need to be sure. Another way to approach this is to consider the total burn time. Let’s say each candle burns at a rate of 1 unit per minute. They all start burning at time t=0. Suppose Peter blows out the first candle at time t1, the second at t2, and so on, with t1 < t2 < t3 < t4 < t5. Then, the remaining length of each candle would be original length minus burn rate times the time it was burning before being blown out. Since original lengths are the same and burn rate is the same, remaining length is original length minus t1, original length minus t2, etc. So, the candle with the most remaining length has the smallest t, meaning it was blown out first. Yes, this confirms the earlier conclusion. Therefore, candle 3, with eight equals, is the first one blown out. Peter lights all five candles at the same time. Since they are the same length and burn at the same rate, the candle that was blown out first would have burned for the shortest time and thus has the most remaining length. The figure shows that candle 3 has the most remaining length with eight "=" symbols. Therefore, candle 3 was the first one Peter blew out. Answer: Candle 3
yes, i'm also thinking candle 3
The first candle blown out should have been the longest one. The LLM was incorrect saying it would be the shortest.
That’s a great catch. You are absolutely correct on this. That’s my mistake. I have tested this question so many times and for some reason I completely overlooked the error the model made.
@@elvissaraviaI thought I was going crazy! Otherwise, great video as always.
@ to be honest, prompts are getting trickier to assess but that’s where things are headed
@@elvissaravia i think you should have actually compared it side by side with o1. That way you are more likely to catch mistakes.
The subject is so wide that they deserve their own pre-print service.
❤
Learn how to build with AI in my new courses here: dair-ai.thinkific.com/ Use code BLACKFRIDAY to get a 35% off. The offer expires 11/29.
no answer how to enable Claude. dislike
Just look at the similar questions others asked and my replies.
This is a really well presented and concise video! Excited to see what OpenAI and Anthropic roll out in the coming months, we've reached the point where AI is actually useful and capable
Thanks Elvis for sharing your thoughts on this new development.
aaaaaaaannnnndddd THEY STILL CAN'T SEE (socialism/equality) YET???
Learn how to build with AI in my new courses here: dair-ai.thinkific.com/ Use code RUclips20 to get an extra 20% off. Limited-time offer!
Cool
You should mention the name of the website.
Learn how to build with AI in my new courses here: dair-ai.thinkific.com/ Use code RUclips20 to get an extra 20% off. Limited-time offer!
Thanks for this course, even with a newbie like me, it was very usueful
Good walkthrough and some interesting thoughts shared, specifically about in addition to covering more programming languages, covering more frameworks like next js. What i would like to add is: is there a way the code llm can be provided the latest documentations (the version specific documentation) of any framework, that is rapidly under development, (example: langchain) so that the llm can leverage it to better assist the developer at debugging and developing....
Yeah there are heaps of ways!
Learn how to build with AI in my new courses here: dair-ai.thinkific.com/ Use code RUclips20 to get an extra 20% off. Limited-time offer!
Claude and Chat-GPT is very sensitive and politically correct. I asked Claude if a certain gun's original magazine was better quality than a different reputable brand and it didn't answer me as it could cause harmful misuse? What the hell even is it on about? Grok on the other than directly answered me. Unfortunately depending on the topic you have to use different AI unless Grok becomes best at everything you use AI for.
NotebookLM made me fall in love with teaching again!
Eventually the people who authors PDFs should just go straight to make them AI friendly and AI first. For people, they should consume the content in more friendly format such as podcast, voice summary etc.
Whats llm do you use to resume article? the same question to use in vídeo
For articles it is Claude and sometimes Gemini for extremely large articles. For videos definitely Gemini due to its native multimodal capabilities.
for deeper and better analysis of pdf's I recommend Google's "NotebookLM". And it's free.
how to use
Grata sorpresa ver que sabes hablar en español ¡Saludos desde Chile! a video for the analysis tool would be great
👍🏻
wait a long time for Haiku 3.5 model ..apparently the price hmm better switch to gpt 4o mini
But ChatGPT can do the same. Do you think the accuracy on Claude is higher?
Would be interesting to compare in a separate video.
Can it understand the relevant topics of the PDF, how they relate, then generate a JSON file so that we can use it with React Flow?
Nevermind, now I see you covered that later in the video. Thanks!
Yeah
Learn how to build with AI in my new courses here: dair-ai.thinkific.com/ Use code RUclips20 to get an extra 20% off. Limited-time offer!
Thanx!!
Can I use the sonnet model with the gitcopilot individual plan?
Here is more information about how they are releasing this: docs.github.com/en/copilot/using-github-copilot/using-claude-sonnet-in-github-copilot#claude-35-sonnet-announcement-and-rollout
Thank you for the thorough explanation and for walking through multiple examples. Your effort is greatly appreciated!
Enjoying hearing from a Belizean and Caribbean man on a technical topic.
wait, some people said its mean 3x cheaper than sonnet ?
it is cheaper idk wtf they are talking about
@@TheUnknownNote exactly, right ? its 3x cheaper , lighter (fast), and more better than sonnet. why people want to complain about this ?
Nonsense price. A bad decision of the maeketing department.
Thanks man this clears up alot i main use the Haiku for some of my little projects. Please can you also cover the catching, that will be great
Microsoft may have squeezed them somewhere (for Copilot adoption), hence the need to reprice their cheaper model.
If that is the price i’ll just use mistral large 2
They can prince it however they want. And when noone shows up, theyll change to the market levels, having wasted alot of customer goodwill.
There's no sense to increase 4x ...
I still can’t understand the reasoning behind it.
Learn how to build with AI in my new courses here: dair-ai.thinkific.com/ Use code RUclips20 to get an extra 20% off. Limited-time offer!
but i dont see this feature in my extension ?! i see only old coplilot
same here
@pablosoria8286 fixed, update your vs code
Layman's terms/peasant terms: for example can scrape an entire website like property taxes map keys in my area and find houses paid off with at least 2 acres and names then put those into a neat list?
Yes but have other steps.
if i didn't have such Trust issues with Google... i would consider using this. BUT! since Google will use your data and not tell you about it.. will keep all my research off of Google's grubby little paws