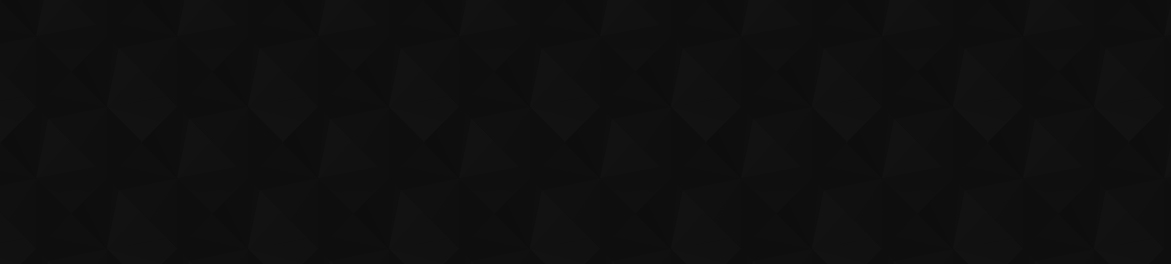
- Видео 85
- Просмотров 38 488
Stanford ILIAD
США
Добавлен 17 мар 2018
Stanford Intelligent and Interactive Autonomous Systems Group (ILIAD) develops algorithms for autonomous systems that safely and reliably interact with people. Using the tools from artificial intelligence, control theory, robotics, machine learning, and optimization, we develop practical algorithms and the theoretical foundations for interactive robots working with people in uncertain, and safety-critical environments.
Lab Website: iliad.stanford.edu/
Lab Website: iliad.stanford.edu/
Bidipta Sarkar's talk on "Diverse Conventions for Human-AI Collaboration"
Supplementary Video for the NeurIPS 2023 paper:
Bidipta Sarkar, Andy Shih, and Dorsa Sadigh. Diverse conventions for Human-AI collaboration. In Advances in Neural Information Processing Systems 36 (NeurIPS), 2023
Paper: arxiv.org/abs/2310.15414
Website: iliad.stanford.edu/Diverse-Conventions/
Code: github.com/Stanford-ILIAD/Diverse-Conventions
Abstract: Conventions are crucial for strong performance in cooperative multi-agent games, because they allow players to coordinate on a shared strategy without explicit communication. Unfortunately, standard multi-agent reinforcement learning techniques, such as self-play, converge to conventions that are arbitrary and non-diverse, leading to poor gene...
Bidipta Sarkar, Andy Shih, and Dorsa Sadigh. Diverse conventions for Human-AI collaboration. In Advances in Neural Information Processing Systems 36 (NeurIPS), 2023
Paper: arxiv.org/abs/2310.15414
Website: iliad.stanford.edu/Diverse-Conventions/
Code: github.com/Stanford-ILIAD/Diverse-Conventions
Abstract: Conventions are crucial for strong performance in cooperative multi-agent games, because they allow players to coordinate on a shared strategy without explicit communication. Unfortunately, standard multi-agent reinforcement learning techniques, such as self-play, converge to conventions that are arbitrary and non-diverse, leading to poor gene...
Просмотров: 295
Видео
Megha Srivastava's talk on "Generating Language Corrections for Teaching Physical Control Tasks"
Просмотров 282Год назад
"Generating Language Corrections for Teaching Physical Control Tasks" Megha Srivastava, Noah Goodman, Dorsa Sadigh International Conference of Machine Learning (ICML) 2023, Honolulu, Hawai'i We design and build CORGI, a model trained to generate language corrections for physical control tasks, such as learning to ride a bike. CORGI takes in as input a pair of student and expert trajectories, an...
Supplemental video for "In-Mouth Robotic Bite Transfer With Visual and Haptic Sensing"
Просмотров 359Год назад
Supplemental video for "In-Mouth Robotic Bite Transfer With Visual and Haptic Sensing", ICRA 2023. View more information and the paper on our website: tinyurl.com/btICRA Music: Music from #Uppbeat (free for Creators!): uppbeat.io/t/sky-toes/the-long-ride-home License code: XHROZYRPSAI2DR4B
Minae Kwon's talk on "Reward Design with Language Models"
Просмотров 646Год назад
"Reward Design with Language Models" Minae Kwon, Sang Michael Xie, Kalesha Bullard, Dorsa Sadigh International Conference on Learning Representations (ICLR), May 2023
Siddharth Karamcheti's talk on "Online Language Corrections for Robotics via Shared Autonomy"
Просмотров 300Год назад
Paper: arxiv.org/abs/2301.02555 "No, to the Right" - Online Language Corrections for Robotic Manipulation via Shared Autonomy Yuchen Cui*, Siddharth Karamcheti*, Raj Palleti, Nidhya Shivakumar, Percy Liang, Dorsa Sadigh 18th ACM/IEEE International Conference on Human-Robot Interaction (HRI), March 2023
Joey Hejna's Talk on "Few-Shot Preference-based RL"
Просмотров 741Год назад
Joey Hejna's Talk on "Few-Shot Preference-based RL"
Priya Sundaresan's talk on "Learning Visuo-Haptic Skewering Strategies for Robot-Assisted Feeding"
Просмотров 276Год назад
Paper Website: sites.google.com/view/hapticvisualnet-corl22/home PDF: openreview.net/pdf?id=lLq09gVoaTE Priya Sundaresan, Suneel Belkhale, Dorsa Sadigh
Megha Srivastava's talk on "Assistive Teaching of Motor Control Tasks to Humans"
Просмотров 218Год назад
Paper: iliad.stanford.edu/pdfs/publications/srivastava2022assistive.pdf "Assistive Teaching of Motor Control Tasks to Humans" by Megha Srivastava, Erdem Biyik, Suvir Mirchandani, Noah Goodman, Dorsa Sadigh
Jennifer Grannen's talk on "Learning Bimanual Scooping Policies for Food Acquisition"
Просмотров 355Год назад
Paper: arxiv.org/abs/2211.14652 Learning Bimanual Scooping Policies for Food Acquisition Jennifer Grannen*, Yilin Wu*, Suneel Belkhale, Dorsa Sadigh
PLATO: Predicting Latent Affordances through Object Centric Play
Просмотров 2562 года назад
Paper: arxiv.org/abs/2203.05630 PLATO: Predicting Latent Affordances through Object Centric Play Suneel Belkhale, Dorsa Sadigh Conference on Robot Learning, December 2022
Erik Brockbank's talk on Incorporate Advice from Artificial Agents when Making Physical Judgments
Просмотров 852 года назад
Paper: arxiv.org/abs/2205.11613 How do People Incorporate Advice from Artificial Agents when Making Physical Judgments? Erik Brockbank*, Haoliang Wang*, Justin Yang, Suvir Mirchandani, Erdem Bıyık, Dorsa Sadigh, Judith Fan Cognitive Science Society Conference (CogSci), July 2022
Andy Shih's talk on "Conditional Imitation Learning for Multi Agent Games"
Просмотров 702 года назад
Paper: arxiv.org/abs/2201.01448 Conditional Imitation Learning for Multi-Agent Games Andy Shih, Stefano Ermon, Dorsa Sadigh 17th ACM/IEEE International Conference on Human-Robot Interaction (HRI), March 2022
Mark Beliaev's talk at ICML 2022 on "Imitation Learning by Estimating Expertise of Demonstrators"
Просмотров 5092 года назад
Paper: arxiv.org/abs/2202.01288 Imitation Learning by Estimating Expertise of Demonstrators Mark Beliaev*, Andy Shih*, Stefano Ermon, Dorsa Sadigh, Ramtin Pedarsani 39th International Conference on Machine Learning (ICML), July 2022
ICRA22 talk for 'Weakly Supervised Correspondence Learning'
Просмотров 1332 года назад
The talk for ''Weakly Supervised Correspondence Learning', which is accepted by ICRA22.
ICRA talk for 'Leveraging Smooth Attention Prior for Multi-Agent Trajectory Prediction'
Просмотров 2392 года назад
ICRA talk for 'Leveraging Smooth Attention Prior for Multi-Agent Trajectory Prediction'
Zihan Wang's Talk on "Learning from Imperfect Demonstrationsvia Adversarial Confidence Transfer"
Просмотров 1602 года назад
Zihan Wang's Talk on "Learning from Imperfect Demonstrationsvia Adversarial Confidence Transfer"
Erdem Bıyık's Talk on "Partner-Aware Algorithms in Decentralized Cooperative Bandit Teams" at AI-HRI
Просмотров 3243 года назад
Erdem Bıyık's Talk on "Partner-Aware Algorithms in Decentralized Cooperative Bandit Teams" at AI-HRI
Erdem Bıyık's Talk on "APReL: A Library for Active Preference-based Reward Learning Algorithms"
Просмотров 4233 года назад
Erdem Bıyık's Talk on "APReL: A Library for Active Preference-based Reward Learning Algorithms"
Andy Shih's Talk on "HyperSPNs: Compact and Expressive Probabilistic Circuits"
Просмотров 3823 года назад
Andy Shih's Talk on "HyperSPNs: Compact and Expressive Probabilistic Circuits"
Songyuan's Talk on "Confidence-Aware Imitation Learning from Demonstrations with Varying Optimality"
Просмотров 3513 года назад
Songyuan's Talk on "Confidence-Aware Imitation Learning from Demonstrations with Varying Optimality"
Suvir Mirchandani's Talk on "ELLA: Exploration through Learned Language Abstraction" at NeurIPS 2021
Просмотров 3493 года назад
Suvir Mirchandani's Talk on "ELLA: Exploration through Learned Language Abstraction" at NeurIPS 2021
Learning Reward Functions from Scale Feedback
Просмотров 2033 года назад
Learning Reward Functions from Scale Feedback
Partner-Aware Algorithms in Decentralized Cooperative Bandit Teams
Просмотров 1833 года назад
Partner-Aware Algorithms in Decentralized Cooperative Bandit Teams
Learning Multimodal Rewards from Rankings
Просмотров 2183 года назад
Learning Multimodal Rewards from Rankings
PantheonRL: A MARL Library for Dynamic Training Interactions
Просмотров 1,1 тыс.3 года назад
PantheonRL: A MARL Library for Dynamic Training Interactions
APReL: A Library for Active Preference-based Reward Learning Algorithms
Просмотров 3383 года назад
APReL: A Library for Active Preference-based Reward Learning Algorithms
Woodrow Z. Wang's Talk at IJCAI 2021 on "Emergent Prosociality in Multi-Agent Games Through Gifting"
Просмотров 1193 года назад
Woodrow Z. Wang's Talk at IJCAI 2021 on "Emergent Prosociality in Multi-Agent Games Through Gifting"
Learning How to Dynamically Route Autonomous Vehicles on Shared Roads
Просмотров 1843 года назад
Learning How to Dynamically Route Autonomous Vehicles on Shared Roads
Minae Kwon's Talk at ICML 2021 on "Targeted Data Acquisition for Evolving Negotiation Agents"
Просмотров 2183 года назад
Minae Kwon's Talk at ICML 2021 on "Targeted Data Acquisition for Evolving Negotiation Agents"
Kejun Li's Talk on Region of Interest Active Learning for Characterizing Exoskeleton Gait Pref's
Просмотров 1233 года назад
Kejun Li's Talk on Region of Interest Active Learning for Characterizing Exoskeleton Gait Pref's
good job
This is excellent. The passive data presentation was very helpful.
Thanks.
Seriously? Are you guys out of your minds? That's a robot without any restriction on it's motion with a sharp EOA attachment (your fork) and you're not even using safety glasses to protect your eyes?
❗ 𝓹𝓻𝓸𝓶𝓸𝓼𝓶
I'm confused by how you get h to transition between s to s' before training the aligning model. How exactly does the data collection of getting the human input work? so to go form s to s' using h, is h being passed through the untrained transformation function in the paper, or is h being initially handled by some other teleoperation control scheme which is then mapped to the transformation map?
Wait, is the idea that. The user sees the transition from s -> s' and then you ask them "how would this look to you on the joystick?" if that's the case, I think I answer my own question. Thank you!
@@michaelprzystupa520 Thanks for the question. Yes exactly, your understanding is correct. We don't assume a default control scheme. In the data collection process, we would show to the user the transition s -> s', and then ask them to give their preferred h for realizing the transition. Please let us know if you have further questions :)
First!
the main issue taht limits the suit is constant misalignemnet of the joints and the lack of a few major axis of movement (leg rotation and abduction for example). this creates a frame that forces the wearer to move unnaturaly and output that can not match human movement by not being able to move like a human. a more anatomicaly correct design (better ankle and hip joint alignment, double joint knee, leg abductor behiind the back and rotator beside it, lengt adjustable upper and lower leg) would greatly improve movement fluidity and accuracy, allowing for more useful software output. a system of bungee cords to simulate the elastic properties of muscle wihout having it rely on beyond peak motor function might help too.
Hi. Where did you publish your codes related to the study?
So in the paper it says that only 2DOF joystick control is used, is that per task? It seems that the user still presses with their right hand sometimes. Is that for switching tasks or different tasks have different controls?
Hello! Within some of the baseline methods the user directly controls the robot's end-effector. This requires 6 DoF, but the joystick only allows 2 DoF at a time. So the user presses a button with their right hand to toggle between different modes.
@@dylanlosey2476 Thanks for the reply! Great work! Just to clarify, for the proposed approach LA + SA, is it correct that when switching from say reaching the tofu to cutting it, the user needs to press a button with their right hand to switch between latent spaces?
@@liyangku1253 With the proposed LA + SA approach, the user never needs to "manually" switch latent spaces. The meaning of the latent space automatically changes as a function of the robot's state and belief. If you see the user pressing a button with our approach, this could just be because they forgot / got confused by the different modes.
great talk, zhangjie !