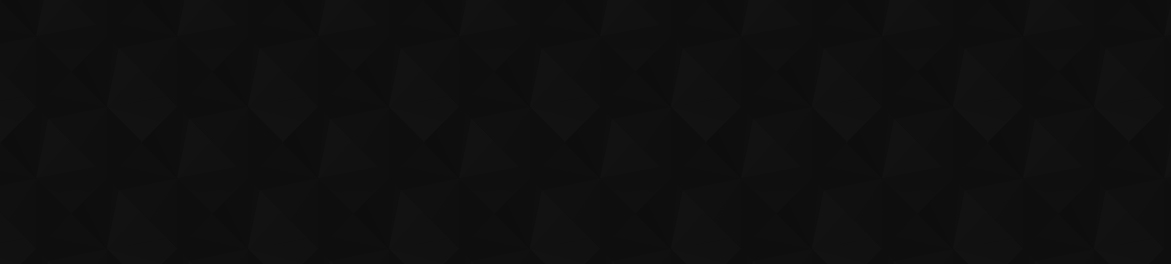
- Видео 196
- Просмотров 50 650
DaCCoTA Biostats Core
США
Добавлен 5 июн 2020
Electronic Health Records for Research
Core Training Event by the Professional Development Core and Research Design, Compliance and Data Management Core of TRANSCEND.
Просмотров: 12
Видео
Python in 10 Lunch Break Session 5
Просмотров 7910 месяцев назад
Recording of the Python in 10 Lunch Break Session, part 5 of 5. Lesson 9: Library Work Lesson 10: Stepping Out Beyond Hello World Bonus: Python in Jupyter Links: www.geeksforgeeks.org/what-is-the-difference-between-pythons-module-package-and-library/ thecleverprogrammer.com/2021/01/23/libraries-packages-and-modules-in-python/ www.makeuseof.com/tag/install-pip-for-python/ med.und.edu/daccota/_fi...
Python in 10 Lunch Break Session 4
Просмотров 1310 месяцев назад
Recording of the Python in 10 Lunch Break Session, part 4 of 5. Lesson 7: Linking Data Lesson 8: Transforming Data Links: med.und.edu/research/transcend/_files/docs/berdc_docs/python_in_10_7_data.zip med.und.edu/research/transcend/_files/docs/berdc_docs/loblolly_wide.csv med.und.edu/research/transcend/_files/docs/berdc_docs/seer_cancer_1.csv med.und.edu/research/transcend/_files/docs/berdc_docs...
Python in 10 Lunch Break Session 3
Просмотров 1910 месяцев назад
Recording of the Python in 10 Lunch Break Session, part 3 of 5. Lesson 5: Extracting Data Lesson 6: Editing Data Links: med.und.edu/research/transcend/_files/docs/berdc_docs/county_level_brain_cancer_incidence.csv www.arb-silva.de/ med.und.edu/research/transcend/_files/docs/berdc_docs/haladaptatus_16s.txt
Python in 10 Lunch Break Session 2
Просмотров 910 месяцев назад
Recording of the Python in 10 Lunch Break Session, part 2 of 5. Lesson 3: Exploring a Large Dataset Lesson 4: Splitting Data Links: www.nber.org/research/data/county-distance-database www.nrcs.usda.gov/wps/portal/nrcs/detail/national/home/?cid=nrcs143_013697 www.tutorialspoint.com/python/python_for_loop.htm www.tutorialspoint.com/python/python_if_else.htm med.und.edu/research/transcend/_files/d...
Python in 10 Lunch Break Session 1
Просмотров 15110 месяцев назад
Recording of the Python in 10 Lunch Break Session, part 1 of 5. Lesson 1: Getting Python Lesson 2: Obligatory Fundamentals Links: www.python.org/downloads/ wiki.python.org/moin/BeginnersGuide/Download www.pythoncheatsheet.org/ data36.com/python-built-in-functions-methods-python-data-science-basics-3/
A Whirlwind Tour of Taxonomy
Просмотров 811 месяцев назад
Hello and welcome to A Whirlwind Tour of Taxonomy, where I will attempt to cover the scope of all living things in fifteen minutes or less.
Exploring Private Research Grants
Просмотров 17Год назад
2023 DaCCoTA Symposium Concurrent Session Khosrow Rezvani, PhD, MD; Jill Weimer, PhD; Michelle Baack, MD
Federal Data Archiving Requirements: Considerations when Working with Sovereign Nation Partners
Просмотров 7Год назад
2023 DaCCoTA Symposium Concurrent Session Bridget Diamond-Welch; Anna Kosloski; Katie Edwards
2023 DaCCoTA Symposium Awardee Presentations Round 1
Просмотров 7Год назад
Awardee Updates: Jonathan Bleeker, MD Kevin Francis, PhD Soojung Kim, PhD Xusheng Wang, PhD Victor Huber, PhD & Khosrow Rezvani, PhD, MD
Sanford PLEDGE: Integrating Screening for Type 1 Diabetes Risk into Routine Pediatric Cares
Просмотров 51Год назад
2023 DaCCoTA Symposium Concurrent Session Kurt Griffin, MD, PhD
2023 DaCCoTA Symposium Awardee Presentations Round 2
Просмотров 9Год назад
Awardee Updates Cheryl Hysjulien, PsyD Dinesh Katti, PhD Marijo Roiko, PhD Melanie Nadeau, PhD Dali Sun, PhD
Until Health Equity Do Us Part: Ways to Initiate & Sustain Partnerships
Просмотров 7Год назад
2023 DaCCoTA Symposium Concurrent Session Keyonna King, DrPH, MA; Emily Frankel, MPH
Handy Research Tools for Immediate Use
Просмотров 27Год назад
2023 DaCCoTA Symposium Concurrent Session Mark Williamson, PhD; Kent Ripplinger, MS; Nick Bittner
2023 DaCCoTA Symposium Poster Presentation: Paola Vermeer
Просмотров 24Год назад
Title: Tumor innervation in high-grade serous ovarian carcinoma.
2023 DaCCoTA Symposium Poster Presentation: Md Saimon Mia
Просмотров 3Год назад
2023 DaCCoTA Symposium Poster Presentation: Md Saimon Mia
2023 DaCCoTA Symposium Poster Presentation: Sabha Ganai
Просмотров 23Год назад
2023 DaCCoTA Symposium Poster Presentation: Sabha Ganai
2023 DaCCoTA Symposium Poster Presentation: Madison Kovar
Просмотров 4Год назад
2023 DaCCoTA Symposium Poster Presentation: Madison Kovar
2023 DaCCoTA Symposium Poster Presentation: Kennedy Forest
Просмотров 6Год назад
2023 DaCCoTA Symposium Poster Presentation: Kennedy Forest
2023 DaCCoTA Symposium Poster Presentation: Steven Wu
Просмотров 8Год назад
2023 DaCCoTA Symposium Poster Presentation: Steven Wu
2023 DaCCoTA Symposium Poster Presentation: Ramkumar Mathur
Просмотров 9Год назад
2023 DaCCoTA Symposium Poster Presentation: Ramkumar Mathur
2023 DaCCoTA Symposium Poster Presentation: Soonhee Roh
Просмотров 7Год назад
2023 DaCCoTA Symposium Poster Presentation: Soonhee Roh
2023 DaCCoTA Symposium Poster Presentation: Andrew Guymon
Просмотров 15Год назад
2023 DaCCoTA Symposium Poster Presentation: Andrew Guymon
2023 DaCCoTA Symposium Poster Presentation: Jadyn Gasper
Просмотров 14Год назад
2023 DaCCoTA Symposium Poster Presentation: Jadyn Gasper
2023 DaCCoTA Symposium Poster Presentation: Valentina Catalan
Просмотров 10Год назад
2023 DaCCoTA Symposium Poster Presentation: Valentina Catalan
2023 DaCCoTA Symposium Poster Presentation: James Foster
Просмотров 4Год назад
2023 DaCCoTA Symposium Poster Presentation: James Foster
2023 DaCCoTA Symposium Poster Presentation: Bailey Pickering
Просмотров 3Год назад
2023 DaCCoTA Symposium Poster Presentation: Bailey Pickering
2023 DaCCoTA Symposium Poster Presentation: Pilar De la Puente
Просмотров 15Год назад
2023 DaCCoTA Symposium Poster Presentation: Pilar De la Puente
2023 DaCCoTA Symposium Poster Presentation: Ying Kang
Просмотров 3Год назад
2023 DaCCoTA Symposium Poster Presentation: Ying Kang
2023 DaCCoTA Symposium Poster Presentation: Komila Rasuleva
Просмотров 11Год назад
2023 DaCCoTA Symposium Poster Presentation: Komila Rasuleva
You did a GREAT JOB with these videos. Many thanks for sharing your knowledge
Greetings from Brazil. You did a very good job in this playlist about sample size. Thank you for posting. Meanwhile I was wondering if you can share the reference for "add 15%" for non-parametric data. Thank you in advance
This was the reference I used. www.graphpad.com/guides/prism/latest/statistics/stat_sample_size_for_nonparametric_.htm
Hi sir, great video! But may I ask in your example of the binomial analysis, why change the observed larger fixed effect of 0.11 to a smaller one, i.e., 0.05? Does that mean even if we have observed a larger effect size, we still have to change it to the number that we hypothesized? Why not stick to 0.11?
The effect size you use depends on the effect size you expect your future experiment (the one you want to run and this estimate sample size for). The main point of changing the effect size in the example is to show that in the SIMR framework, you can manually change the effect size. So maybe you think the effect size of 0.11 from the dataset is an accurate estimate of what the future experiment would produce. Then, use the 0.11. But perhaps you think that the real effect size is smaller than that, say about 1/2 that size. Then, adding the effect size of 0.05 manually would let you generate the sample size for such an effect.
@@daccotabiostatistics Clear explanation! Thank you very much!😀
This is what I was looking for. Absolutely brilliant. Thanks a ton !!!
Hello. I am sure I am asking a dumb question, but I can't seem to find answers anywhere and I don't know how to take an r package apart to understand the code yet. It seems to me that pwr.chisq.test (if df==2) and pwr.2p.test are the same thing, but yield different answers. May I ask, why that is?
Thank you! Extremely useful summary
Thank you so so so much, Professor. Very helpful and well-paced presentation with helpful images and adequate narrative.
Hi thanks alot for this, how would I then incorporate the replication
I am not sure what you mean.
What a great video, thank you very much. I have a question regarding Example 5. So let's pretend that extending the number of sites to 24 let us achieve 90% power. Great, so in our real study, we know we'll have sufficient power... but for what, exactly? The effects, or also each of their levels? For example, our fixed effect of modification has three levels, each with their own effect sizes. Would we have achieved enough power to make comparisons such as "mod1 and mod2 lead to statistically equivalent levels of the DV "abundance", but mod3 is statistically different (and higher) from both of them, therefore mod1 and mod2 have identical efficacy but mod3 is more effective than both"? Or are we only powered for modification in general, such as "there is a significant effect of modification"?
That is a good question. I think the sufficient power is for the overall model. Then, if the overall model is significant, you'd look at the F-values for specific variables and then compare mean values across different levels for what the significant difference was.
thank you very much sir!!❤❤❤💯!
1:11:48
Great intro thanks - is there any way you could repost this without the music? I'm finding it really difficult to follow with the background noise
I don't have plans to repost without music in the short term. You can access the content in slide format using the link below: med.und.edu/research/daccota/_files/pdfs/berdc_resource_pdfs/sample_size_r_module_glmm2.pdf
Is there any way that Can I get the pdf for the presentation.
med.und.edu/research/daccota/_files/pdfs/berdc_resource_pdfs/sample_size_r_module_glmm2.pdf
Professor! The previous values of two groups used in the example of T-test sample size. These values are from a pilot experiment or where I can get this?
The mean and standard deviations for the t-test (m1=20, m2=25, std1=10, std2=10) were just used for an example. There is no underlying data. However, if you wanted to, you could use the rnorm function in R to generate sample data. rnorm(n=10, mean=20, sd=10) rnorm(n=10, mean=25, sd=10)
Very helpful! Given that you are running the power curve off data, would this yield post-hoc power estimates rather than a priori estimates?
My understanding would be that if the data is what you actually want to analyze, yes, it would be post-hoc. But if the data is preliminary data to get an estimate for a full study, I'd consider it a priori.
your resources are really helpful. i am using the poisson, with one random effect. What if you do not have a set/fixed number of doctors, but you still want to control for this variable to avoid violation of independence of observations - how many would you need for a 80% power? Will it still be 14 days for me to get the minimum sample size?
Thanks for great explanation! Are you giving a private lessons? I would need them
Thank you for your videos and this channel, it brings me back the hope that I will understand what I am learning on the Biostatistics studies! Please don't stop this work as it is really helpful!
Is there a similar video looking at just GLMs? :3
Not that I've made. I haven't found a good approach to GLMs in R for power analysis.
@@daccotabiostatistics thank you. I think SIMR works on glm() objects as well.
you can estimate power in GLMs using the pwr function-very simple
thanks for sharing/organizing these -omics!
I love that this has 2013 video aura ❤️
Following from NYC!
Very systemic series for Power analysis. Clearly explained. Thank you. The only question is for the two examples of Multilevel Modeling. To me, they are identical research questions. Why are they calculated by difference codes?
Looking in WebPower manual (cran.r-project.org/web/packages/WebPower/WebPower.pdf), Cluster randomized trials and multisite randomized trials are described the same way, as shown below. "[CRT/MRT] are a type of multilevel design for the situation when the entire cluster is randomly assigned to either a treatment arm or a control arm (Liu, 2013). The data from [CRT/MRT] can be analyzed in a two-level hierachical linear model, where the indicator variable for treatment assignment is included in second level. If a study contains multiple treatments, then multiple indicators will be used." In the variable inputs between the two functions, CRT is much simpler, just needing the icc in addition to the number of clusters, alpha, power, and effect size. MRT needs three variances (tau00, tau11, sg2). I think this is because the MRT can look at three types of tests (main, site, and variance).
@@daccotabiostatistics Thank you so much for clarification.
Thank you for you videos. But I am sorry if I do not anderstand why do I have to simulate data. If I have been asked to perform statistical analysis on a retrospective study and I want to check the power of the study , why can I base my estimation of power on the real data ? And how do I do this in LMM (the code is not working....)
From what I understand, linear mixed models (and other similar models) are more complex, so simulation is better than replying on specific formulas (see: stats.stackexchange.com/questions/373597/how-can-you-compute-sample-size-for-a-linear-mixed-model-gpower-only-does-repe). My default for power analysis is G*power, but it doesn't have anything for more complex linear models. Therefore, I found SIMR in R for such things. I suppose you could do something like run power analysis for linear multiple regression in G*power and just ignore the random effects. As for code not working, if you could provide more details, I might be able to help. SIMR doesn't always work smoothly for me either.
Great series of videos, many thanks! Do you know an easy way to calculate Bayes Factors in SAS?
Hi. Thanks for comprehensively discussed the logistic regression Gpower. May I know if there is publication of this so that I can cite you in my paper. Thanks.
I do not have a publication on this. These were put together as video/pdf source. You could cite the PDF document. med.und.edu/daccota/_files/pdfs/berdc_resource_pdfs/sample_size_gpower_module.pdf
Can I cite this? Do you have published journal on this? Thanks.
THANKS
Hello sir, can you share the script?
Thank you very much for the informative presentation!
Thanks for uploading, well explained !!
Fantastic examples and explanation! Thank you!
Thank you so much for such a clear explanation of all of this! I love the classical music in the background, I think it is fantastic!
Thank you very much sir
Thank you for this video. It is very helpful. I am doing a study in which I am using PLS (using SmartPLS software) analysis. I need to do a power analysis. Can you suggest how I can do that? Can I use G*Power software or I should use R?
I am not very familiar with partial least squares (PLS) methods. However, there does appear to be a paper that goes through a tutorial on power analysis in R: www.researchgate.net/publication/281690984_Sample_Size_Determination_and_Statistical_Power_Analysis_in_PLS_Using_R_An_Annotated_Tutorial
For those looking for the next videos: ruclips.net/video/ryhn5o8RIrE/видео.html ruclips.net/video/lhoEllpKBkA/видео.html and the data can be found here: med.und.edu/daccota/berdc-resources.html
GREAT 👍 thanks
sir, may i ask why power curve result turn to be like this "Error in arrows(c("11", "13", "15", "17", "19", "2", "3", "5", "7", "9" : invalid first argument".. i have already run multiple times act..
It is hard to tell without seeing the full code. But I don't see how the arrows function and a list of numbers as characters would be used in running a power curve.
@@daccotabiostatistics if you don't mind, can I email it to you sir?.. so you could have a look and give an opinion.. its important to me
Thank you so much for explaining everything in such a nice way! I really appreciate it. In the model of minute 8:18, lmer(Reaction ~ Days + (Days | Subject) if my understanding was correct, we can interpret the parenthesis part as "subject as a random effect within day." On the other hand, in the two models of minute 12:13, the parenthesis part is (1 | herd). I was wondering: 1) how should be interpreted this part (what is the meaning of 1)? and 2) why we put a number instead of the name of a variable? Thank you in advance for your time and consideration :)
The format (1|group) represents a random group intercept. The number 1 is sort of a placeholder. So for (1|herd), herd is the random effect with random intercept. The format (X|group) represents a random slope and random intercept, that is a random slope of X within group with correlated intercept. This is a good resource I found that explains random effects models in R: bbolker.github.io/mixedmodels-misc/glmmFAQ.html
@@daccotabiostatistics Thank you so much for your kind reply and for your time. I really appreciate it 🙏🏼 And the link that you shared is sooo good. Many thanks. Wishing you a good day :)
The music really does not help to concentrate...
Hello and thank you for the video I would like to use GLMM multinomial logistic regression mixed model for repeated data with R software, response ~ trt + period + seqTrt + (1|id) do you know a package or a function for this model thank you in advance
I have not run a multinomial logistic regression for repeated data, so I can't say for sure. I would start with trying out a simple case of multinomial logistic regression. Here is a good source from UCLA for trying that out (stats.idre.ucla.edu/r/dae/multinomial-logistic-regression/). From there, you could test if the multinom function can handle repeated data. Otherwise, I did find this post that may be useful (hlplab.wordpress.com/2009/05/07/multinomial-random-effects-models-in-r/). Hopefully those sources help.
@@daccotabiostatistics thank you :-)
Hi Will, thanks for taking the time to make these videos! they're so good! I have a question: how would you run a powerSim() for a Gamma model? I have been trying to follow your approach but I keep getting all zero values :(
You'll want to make sure your model has both a fixed and a random effect, glmer(y~x+(1|z)). I tried just having a fixed effect and got the same problem. Below is R code that I got to work for a simple simulated dataset drawn from a gamma distribution. library(lme4) library(simr) #create simulated dataset mygamma1 <- rgamma(n=20, shape=2, rate=2.0) mygamma2 <- rgamma(n=20, shape=2, rate=2.5) hist(mygamma1) hist(mygamma2) gamma_df <-data.frame('treatment'=c(rep(1,20),rep(2,20)), 'block'=rep(seq(1,10,1),4), 'rate'=c(mygamma1,mygamma2)) View(gamma_df) boxplot(rate~treatment, data=gamma_df) #create model gamma_glm <-glmer(rate~treatment+(1|block), family="Gamma",data=gamma_df) summary(gamma_glm) fixef(gamma_glm)["treatment"] powerSim(gamma_glm, nsim=50) #extend number of blocks gamma_glm_ext <- extend(gamma_glm, along="block", n=60) gamma_PC <-powerCurve(gamma_glm_ext, along="block", breaks=c(20,30,40,50,60)) print(gamma_PC)
@@daccotabiostatistics Thanks very much! I will work through the code provided in the morning (it's way past bedtime here in Australia). But just as a reference, my model looks somewhat similar to the model you created: glmer(gamma_distributed_score ~ time + age + sex + score.1 + (1 | patient.id), family = Gamma(link="identity"), data = mydata) ### time has only two levels. Thanks again for your help will let you know how I go!
Best video on the internet! Thank you so much
Thanks
Great! Keep the good work
Power Analysis at 13:02
Can you explain more why you use fcompare in the treatment time model ?
The 'test' option specifies the test to perform. Otherwise, the first fixed effect will be tested by default. So, in the example, I wanted to look at 'time' specifically and then 'treat' specifically, so I set it that way.
The content is organized and helpful. The violin bgm and your sudden loud voice are awful however.
Thanks for the feedback. I've been working to make sure the videos don't have sudden shifts in volume.