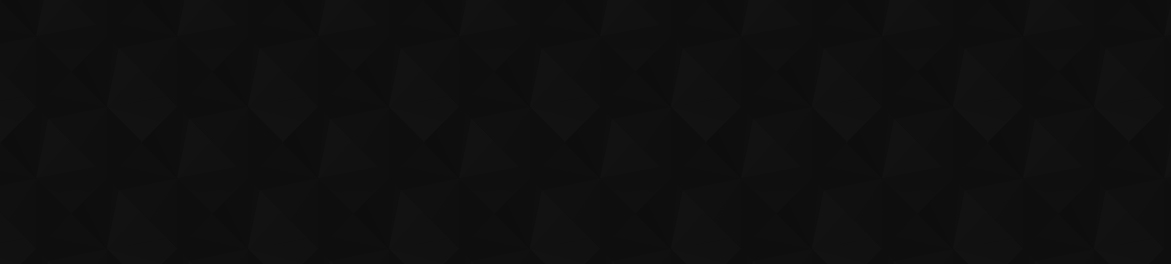
- Видео 300
- Просмотров 150 827
Statistical Learning and Data Science
Германия
Добавлен 26 фев 2019
I2ML - Supervised Classification - Linear Classifiers
This video is part of the open source online lecture "Introduction to Machine Learning". URL: slds-lmu.github.io/i2ml/
Просмотров: 61
Видео
I2ML - Supervised Classification - Naive Bayes
Просмотров 452 месяца назад
This video is part of the open source online lecture "Introduction to Machine Learning". URL: slds-lmu.github.io/i2ml/
I2ML - Supervised Classification - Discriminant Analysis
Просмотров 722 месяца назад
This video is part of the open source online lecture "Introduction to Machine Learning". URL: slds-lmu.github.io/i2ml/
I2ML - Supervised Classification - Basic Definitions
Просмотров 902 месяца назад
This video is part of the open source online lecture "Introduction to Machine Learning". URL: slds-lmu.github.io/i2ml/
I2ML - Supervised Classification - Logistic Regression
Просмотров 722 месяца назад
This video is part of the open source online lecture "Introduction to Machine Learning". URL: slds-lmu.github.io/i2ml/
I2ML - Supervised Classification - Tasks
Просмотров 452 месяца назад
This video is part of the open source online lecture "Introduction to Machine Learning". URL: slds-lmu.github.io/i2ml/
I2ML - Random Forest - Bagging Ensembles
Просмотров 1207 месяцев назад
This video is part of the open source online lecture "Introduction to Machine Learning". URL: slds-lmu.github.io/i2ml/
I2ML - Random Forests - Out-of-Bag Error Estimate
Просмотров 2997 месяцев назад
This video is part of the open source online lecture "Introduction to Machine Learning". URL: slds-lmu.github.io/i2ml/
I2ML - Random Forest - Proximities
Просмотров 1067 месяцев назад
This video is part of the open source online lecture "Introduction to Machine Learning". URL: slds-lmu.github.io/i2ml/
I2ML - Random Forest - Feature Importance
Просмотров 5627 месяцев назад
This video is part of the open source online lecture "Introduction to Machine Learning". URL: slds-lmu.github.io/i2ml/
I2ML - Random Forest - Basics
Просмотров 2147 месяцев назад
This video is part of the open source online lecture "Introduction to Machine Learning". URL: slds-lmu.github.io/i2ml/
I2ML - Tuning - In a Nutshell
Просмотров 797 месяцев назад
This video is part of the open source online lecture "Introduction to Machine Learning". URL: slds-lmu.github.io/i2ml/
SL - Regularization - Non-Linear Models and Structural Risk Minimization
Просмотров 1287 месяцев назад
This video is part of the open source online lecture "Supervised Learning". URL: slds-lmu.github.io/i2ml/
SL - Regularization - Geometry of L2 Regularization
Просмотров 517 месяцев назад
This video is part of the open source online lecture "Supervised Learning". URL: slds-lmu.github.io/i2ml/
SL - Regularization - Weight Decay and L2
Просмотров 707 месяцев назад
SL - Regularization - Weight Decay and L2
SL - Regularization - Geometry of L1 Regularization
Просмотров 437 месяцев назад
SL - Regularization - Geometry of L1 Regularization
SL - Regularization - Bayesian Priors
Просмотров 797 месяцев назад
SL - Regularization - Bayesian Priors
SL - Regularization - Early Stopping
Просмотров 297 месяцев назад
This video is part of the open source online lecture "Supervised Learning". URL: slds-lmu.github.io/i2ml/
SL - Regularization - Other Regularizers
Просмотров 317 месяцев назад
SL - Regularization - Other Regularizers
SL - Regularization - Lasso Regression
Просмотров 387 месяцев назад
SL - Regularization - Lasso Regression
SL - Regularization - Lasso vs. Ridge
Просмотров 377 месяцев назад
SL - Regularization - Lasso vs. Ridge
SL - Regularization - Ridge Regression
Просмотров 597 месяцев назад
SL - Regularization - Ridge Regression
SL - Regularization - Elastic Net and regularized GLMs
Просмотров 347 месяцев назад
SL - Regularization - Elastic Net and regularized GLMs
SL - Information Theory - Information Theory for Machine Learning
Просмотров 676Год назад
SL - Information Theory - Information Theory for Machine Learning
SL - Information Theory - Joint Entropy and Mutual Information II
Просмотров 131Год назад
SL - Information Theory - Joint Entropy and Mutual Information II
SL - Information Theory - Joint Entropy and Mutual Information I
Просмотров 216Год назад
SL - Information Theory - Joint Entropy and Mutual Information I
SL - Information Theory - KL Divergence
Просмотров 194Год назад
SL - Information Theory - KL Divergence
SL - Information Theory - Cross Entropy and KL
Просмотров 137Год назад
SL - Information Theory - Cross Entropy and KL
SL - Information Theory - Differential Entropy
Просмотров 629Год назад
SL - Information Theory - Differential Entropy
Super🎉
danke
gern geschehen.
Hii i can help u for reaching monitization for ur channel
Thanks, that was a great video!
Good explanation! Thank you so much!
There is an error in the 1st slide. Entropy of the fair coin equals 1 bit, not 0.7. It's probably natural log was used in this graph.
Really enjoyed the guitar tuning analogy. Can’t stand playing out of tune guitars or when the intonation is slightly off.
Is Stock price prediction an suitable real world use case for this Online learning ?
YES
Appreciate it 👍
Hi,professor ! I have a question: I remember in previous video I learned that random forest can deal with missing data by surrogate splitting, but now in this video professor said proximities can be used for imputation. I'm confused. If random forest can deal with missing data then why do we need imputation? And in this video in imputation part, step one is using median to impute the data, why we need to impute if random forest already can use surrogate splitting to get the prediction result? why dont we use the result generated by surrogate splitting to compute proximity to impute the data and keep doing step 2 and 3? Besides, I feel I dont totally understand how does surrogate splitting work. I searched on the internet, but I still dont know the details. I just know it is about finding another variable that can generate the good result as variable[the primary split] with missing values. But how does the calculaton happen to make us know that the variable with missing values can be the best split at specific point[and if we already know that the variable with missing values can be a good split at specific point then why do we need surrogate splitting]?
The question is a bit long, but wish for your replies! Thank you!
Absolute gold
great video thanks, very clear
thank you
how do we get phi values in step 4?
LET'S GOOOOOOOO 💫💫 THANK YOU FOR TAKING YOUR TIME TO MAKE THESE VIDEOS💯💯💯💯❤❤
Is this algorithm inspired by k-means clustering
Thank you! You explained thing very clearly.
Very helpful video. The visualization in the OOB is very easy to understand. Thank you!
great lecture! what if some of the variables to be optimized are limited in a certain range? using multivariate normaldistribution to generate offspring might exceed the range limit?
Excuse me, but wouldn't z1+z2+z3+....zT be (-1)^T/2 instead of (-1/2)^T? Anyway, you did a great job. Congratulations!!
first :)
It's so hard to hear what you're saying, please amplify the audio post processing on your future uploads. Excellent presentation nonetheless, you explained it so simply and clearly. <3
Thank you. We are still not "pros" with regards to all technical aspects of recording. Will try to be better in the future.
at 4:00 isn't the square supposed to be inside the square brackets?
HI, great course, however a small note, at 12:20 I think that the function on the left might not be convex, as the tangent plane in the "light blue area" is on top on the function, and not below the function, thus violates the definition of convexity (afaik they are called Quasiconvex function that sort of functions)...
finally found out clear logic behind the weights. Thank you so much🎉
Nice video. I have a question. How to calculate shapley value for classification problem?
to not violate axiom, do it in logit space
Thank you for doing this video in Numerator layout. It seems many videos on machine learning use Denominator layout but I definitely prefer Numerator layout! Is it possible you could do a follow-up video where you talk about partial derivative of scalar function with respect to MATRIX ? Most documents I've looked at seem to use Denominator layout for this type of derivative (some even use Numerator layout with respect to VECTOR, and then switch to Denominator layout with respect to MATRIX). I assume it's because Denominator layout preserves the dimension of the matrix, making it more convenient for gradient descent etc. What would you recommend I should do?
💐 Promo'SM
So, the permutation order was only to define which feature will get the random value? Not creating a whole new instance with feature order same as the permutation order? (The algorithm shows S, j, S- , but your example shows S, S-, j)
Thank you!
Nice lecture. I also recommend Dr. Ahmad Bazzis convex optimization series.
Great work
Hi, sadly your github link doesnt work for me. Thanks for the video.
Can you explain more about the ommitted variable bias in M-plots? My teacher told me that you can use a linear transformation to explain the green graph by transofmring x1 and x2 to two independent random variables x1 and U. Is that true?
Thank you so much for this video. Consider example on 7:20 min. -- but doesn't it look like feature permutation? Shouldn't I use expected values for other variables (x2,x3) ? Thanks in advance.
Thanks
the material is not accessible right now, can someone reupload it ?
what do you mean with "pessimistic bias" ?
If i understand correctly, it is pessimistic, because you use lets say 90% of your available data as training set and 10% as test set. So the model you test is only trained on 90% of your data, but your final model that you use/publish will use 100% of the data. This means it will probably perform better than your training model that used 90% but you cant validate it because you have no test data left. In the end you evaluate the model on 90% of the data which is probably slightly worse than the model that is trained on 100% of the data.
good explanation madam
Good Explanation Madam
Hallo, I'm a student in TU dortmund, our lecture also use your resources, but the link in the description is not working now, how can we get access to the resources?
Great Explanation!! Thank You
Excellent. Thanks
Finally some serious content
Thanks Sir, but excuse myself (zero statistics & mathematics background), but what does this video suggest about using Brier Score for measuring forecast?
Nice explanation! You are using the same set of HP configurations λi, with i=1, ...,N through fourfold CV (in the innner loop). But, what happend if I would like to use a Bayesian hyperparameter to sample the values of the paramters? For example, for each outer cv with its corresponding inner cv, could I use a Bayesian hyperparameter search? Then the set of HP configuration wouldn't be the same in each inner cv, and then the question is: Can be the set of HP configurations different in each inner cv and is it still valid this nested cross valitadion method?
awesome!! thanks so much!
thank you, well explained.
Heres a free resource on one of the most important academic concepts of the modern age: 800 views. GG humanity. GG
thank you for the explanation