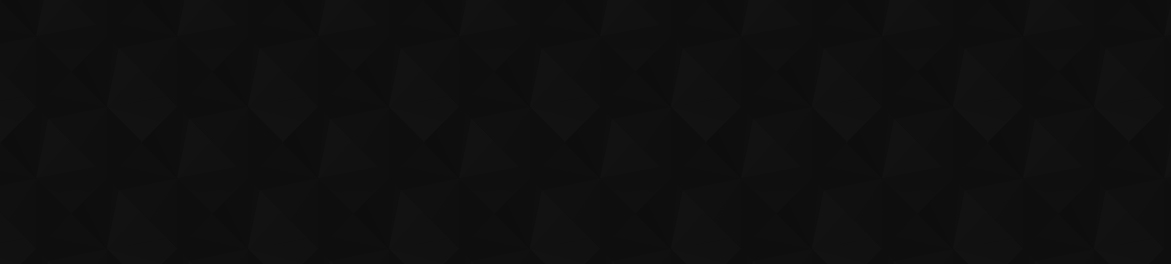
- Видео 18
- Просмотров 78 297
Jonathan Stallrich
Добавлен 28 июл 2014
Видео
8 Analyzing Repeated Treatment Designs in SAS
Просмотров 9 тыс.7 лет назад
8 Analyzing Repeated Treatment Designs in SAS
8 Constructing IBDs and Confounded Block Designs in JMP
Просмотров 7847 лет назад
8 Constructing IBDs and Confounded Block Designs in JMP
7 Analysis of Unreplicated Factorial Experiments in JMP
Просмотров 3828 лет назад
7 Analysis of Unreplicated Factorial Experiments in JMP
Hi, I conducted a three-factorial [ pH (2 levels), salt(3 levels), and iron (3 levels) in a completely randomized design conducted with 3 replications. I want to do an orthogonal test on the second and third levels of iron at the first levels of pH and salt in SAS. However, I get the message NOTE: CONTRAST one is not estimable. Could you please tell me where I am making mistake? Data factorial; input ph salt iron rep yield; cards; 1 1 1 1 3.5 1 1 1 2 3.8 1 1 1 3 3.4 1 1 2 1 4.1 1 1 2 2 4.3 1 1 2 3 4.5 1 1 3 1 4.6 1 1 3 2 4.8 1 1 3 3 4.9 1 2 1 1 3.1 1 2 1 2 3.2 1 2 1 3 3 1 2 2 1 3.4 1 2 2 2 3.5 1 2 2 3 3.3 1 2 3 1 3.5 1 2 3 2 3.8 1 2 3 3 4 1 3 1 1 3 1 3 1 2 2.98 1 3 1 3 2.8 1 3 2 1 3.1 1 3 2 2 3.5 1 3 2 3 3.2 1 3 3 1 3.6 1 3 3 2 4.1 1 3 3 3 3.4 2 1 1 1 3.2 2 1 1 2 3.4 2 1 1 3 3.1 2 1 2 1 4.1 2 1 2 2 4 2 1 2 3 4.2 2 1 3 1 4.3 2 1 3 2 4.5 2 1 3 3 4.3 2 2 1 1 2.98 2 2 1 2 2.8 2 2 1 3 2.7 2 2 2 1 3.2 2 2 2 2 3.1 2 2 2 3 3.3 2 2 3 1 3 2 2 3 2 3.4 2 2 3 3 3.6 2 3 1 1 2.65 2 3 1 2 2.75 2 3 1 3 2.5 2 3 2 1 3 2 3 2 2 2.98 2 3 2 3 2.95 2 3 3 1 3.2 2 3 3 2 3.5 2 3 3 3 3.1 ; proc glm; class ph salt iron rep; model yield=ph salt iron ph*salt ph*iron salt*iron ph*salt*iron/ss3; contrast 'one' iron 0 1 -1 ph*salt*iron 0 1 -1 0 0 0 0 0 0 0 0 0 0 0 0 0 0 0/e; run;
Extremely helpful. Thanks!
Thanks a lot Jonathan. This was very helpful
great tutorial, thanks
thanks for the video. Is it possible to add letters (A, B, C, etc) to indicate significance of the least square means or an additional table showing the treatment means and the letters of significance and what would be the syntax to show this? Thanks!
al parecer eso solo se puede usando unos MACROS que no he podido conseguir. Utilicé proc glimmix y con ese si pude obtener las letras en las medias. proc glimmix data=PARDCD; class REP GRAN PEQ; model REND= GRAN PEQ GRAN*PEQ; random REP GRAN*REP; lsmeans GRAN PEQ GRAN*PEQ / pdiff=all adjust=tukey lines plot=mean(sliceby=PEQ join);
Can we perform/ demonstrate superiority/non-inferiority using this confidence intervals if I want. If yes then let suppose superiority margin is 2.5 for comparison one then what confidence interval we should get so that we can claim that treatment 1 is superior than treatment 2.
Hi Jonathan, Hope you are doing well
What is the difference of a covariate model compared to simply using the (End - Initial weight) as the dependent variable?
Good question and one I just encountered when I taught this class last semester! Let's say the true model is Y=b0 + bx + tau + E where x is the initial weight and b is the slope for the covariate. If we subtract x from both sides, as you suggest, we get (Y-x) = b0 + (b-1)x + tau + E. So the only way this removes the covariate effect is if b=1, which seems unlikely. The only reason way I can recommend doing an analysis on Y-x is if it is a meaningful quantity. Otherwise, it doesn't "remove" the covariate effect. It's better, in my opinion, to just work with the original Y value.
@@jonathanstallrich2143 OK, thank you so much for your reply, now I understand it better!
Hi, how do you interpret the coefficients of mixed effects model?
Hey, How can I contact with you? Do you have any Email address?
so blurry!
Hi Jonathan, very interesting video. What if I need to include also blocks within my factorial analysis? A database with data on wheat production (tons per hectar) treated with two doses of fertilizer and irrigated or not but on a slope (so I have to consider the gradient and add blocks)
All you need to do is include the blocking factor as a model effect (make sure it is a Nominal factor)! What is sometimes confusing about performing a block analysis in software is that it is done exactly the same as if that blocking factor were a treatment factor. It is up to the user to know what parts of the output are relevant. In particular, the p-value associated with the test for block effects is not valid. But including the block effect will hopefully reduce your experimental error.
You nailed it! I was including blocks that were just numbers (1, 2, 3...). Now I simply changed them into a, b, c and it works. Thank you very much!
You're welcome! It is a very common mistake. This is why it is important to know how many degrees of freedom to expect an effect to take up. If the block is a continuous factor, it is fitting a regression relationship which only has 1 degree of freedom. It should have b-1 degrees of freedom where b = # blocks.
Great. Since you have been so kind I would like to ask you another question. What if I want to reapeat these measurements over time? Is it also possibile to perfom a repeated measure ANOVA with JMP?
Very helpful and nice video, Thanks a lot
Dear : Mr Jon S. According to your comment on. I concern that you are the best instructor to teach me about SAS. My name is Rayudika who is doctoral student of SUT in Thailand. Indeed, recently time of this month is conducting of my synthesis from data meta analysis. To be honest, I never use SAS program, consequently, I know nothing about that. My pleasure to accept your considerable is coming true when you give me it. My data : Factor (A) effect for parameter (X) with using separately methods : Method 1 and method 2. Would you like to suggest me to input the data (SAS language code) on PROC MIX ? Yours sincerely, Rayudika Aprilia Patindra Purba Animal Production, SUT, Thailand rayudikaapp.007@gmail.com 14 December 2016
what about setting up random intercepts
Whenever you put a classification variable in a RANDOM statement you are creating random intercepts for each category. This is essentially what is done with the subsampling model, where random intercepts are included for each experimental unit.
I'm trying to make sure I am including the random var appropriately. I know it appears in the class statement because its categorical, however should it also be in the model statement? ex. variance across sites and across doctors given patient response of satisfaction.
What is the difference between stating random variable versus random intercept variable
The MODEL statement only includes fixed effects. If you put a categorical effect in the MODEL statement and RANDOM statement then you will be introducing identifiability issues. A random intercept variable is introduced when you have a random categorical variable in the random statement. Each category is given a random effect in the model, hence a random intercept is assigned to all observations having that corresponding category.