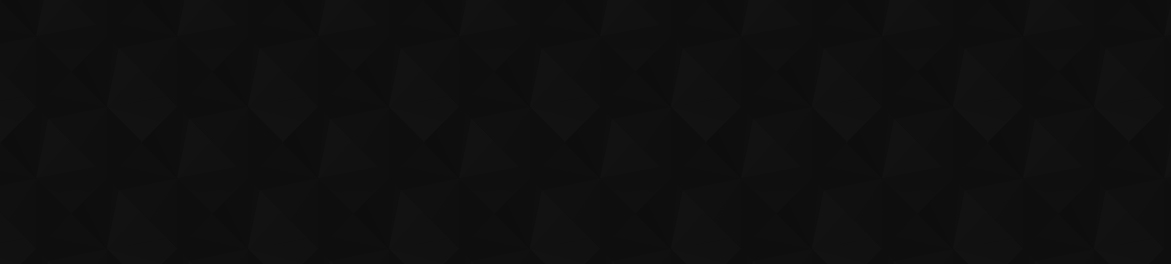
- Видео 113
- Просмотров 59 554
Ketan Rajawat
Добавлен 13 июн 2007
Optimization for Big Data
Non-convex Optimization via Convex Optimization Algorithms
Non-convex problems can sometimes be solved exactly (by recognizing hidden convexity, proving strong duality, or using bisection) or inexactly (relaxing, or via algorithms such as BCD, MM, SCA).
Просмотров: 935
Видео
Transformations that preserve Convexity
Просмотров 698Год назад
Transformations that preserve Convexity
Supporting and Separating Hyperplanes
Просмотров 2 тыс.Год назад
Supporting and Separating Hyperplanes
Change of Variables
Просмотров 545Год назад
Equivalent optimization variables via change of variables using a one-to-one function
Operations on Convex Sets
Просмотров 817Год назад
Intersection, Affine Transformation, Product of Sets, Minkowski sum.
Addition and Elimination of Variables
Просмотров 453Год назад
Equivalent problems via adding slack variables and the epigraph trick
Singular Value Decomposition
Просмотров 634Год назад
The four fundamental spaces, SVD, and the link between SVD and EVD.
Relating Convex Sets and Functions
Просмотров 745Год назад
Definitions of epigraph and of quasi-convex functions
First and Second Order Conditions for Convexity
Просмотров 2,4 тыс.Год назад
These conditions allow us to determine if a function is convex or not using first and second order derivatives of the function.
PSD Cone
Просмотров 862Год назад
Here we see how sets can also be expressed in terms of matrices. We look at the set of symmetric matrices as well as the set of PSD matrices.
Positive Definite Matrices
Просмотров 726Год назад
We provide several definitions of positive definite and positive semidefinite matrices.
L1 and L-Infinity Norm Minimization (Approximation Problems)
Просмотров 1,9 тыс.Год назад
We see how minimizing the l1 norm of the residuals makes the fit robust to outliers. In contrast, minimizing the l-infinity norm of the residuals makes the fit sensitive to outliers.
LP in Communications Systems
Просмотров 473Год назад
A communication example, showing how power control is possible via LP
Motivation for Convex Optimization
Просмотров 1,2 тыс.Год назад
This video motivates the need to study convex optimization.
Examples of Convex Sets
Просмотров 1,2 тыс.Год назад
Convex Cone, Convex Conic Hull, Hyperplane, Half-space, Polyhedron
Convex Problems
Просмотров 672Год назад
Convex optimization problems, manipulating problems and equivalent problems
Ax+b use this is wrong
thanks
great videos
show me by optimization problem
absolutely awesome. I like that you try to give visualized examples which helps with better understanding
ధన్యవాదములు धन्यवाद:
Hello Sir, Thank you for providing this wonderful explanation. The process of feature selection through seems pretty similar to PCA. Am I correct? Can you please provide an explanation about the similarities and dissimilarities between LASSO and PCA? Which technique should be used in which scenario? As I understand, PCA helps us decompose the variance of factors and if required we can drop some of those factors that do not significantly contribute to the variance. In case my understanding is incorrect, please do point out the flaw in my understanding. Thanks.
how to do optimisation for a product of convex functions ?
Thank you, very nice! 🙂
Great explanation sir ❤
This was extremely clear, thank you very much. I was struggling with presentations and textbooks which tend to be heavy on the proof side but light on the explanation and example side. This video was exactly what I needed.
Thank you very much.
Thank you very much.
Thank you very much for this great tutorial.
which book should I follow for this topic
Thanks sir, Is the pdf available?
Nice explaination❤
69
Best explanation 👌👌 Thanks a lot sir 🙏🙏
hello professor can you share with me your email address
great video
Thank you so much for the video At 14:25 - how did you get 0 and 0 in the first matrix?
🤘 *promo sm*
You made it look easy 😱 wow!!
In which topic we studied the formulation of lambda(max). I am not able to recall!