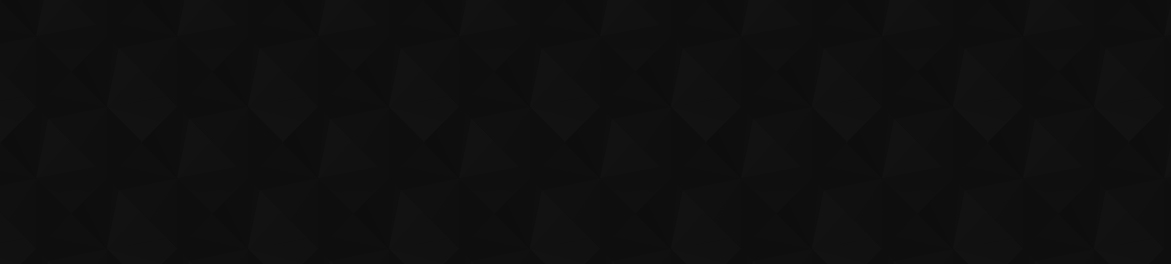
- Видео 79
- Просмотров 33 464
CAMLIS
Добавлен 7 май 2018
Graph-Based User-Entity Behavior Analytics for Enterprise Insider Threat Detection
CAMLIS 2023, Grant Gelven and Shannon Strum
Просмотров: 200
Видео
Don’t you forget NLP: prompt injection using repeated sequences in ChatGPT
Просмотров 2788 месяцев назад
CAMLIS 2023, Mark Breitenbach, Adrian Wood, Win Suen and Po-Ning Tseng
Playing Defense: Benchmarking Cybersecurity Capabilities of Large Language Models
Просмотров 2328 месяцев назад
CAMLIS 2023, Adarsh Kyadige, Salma Taoufiq, Younghoo Lee, Tamas Voros and Konstantin Berlin
LLM Prompt Injection: Attacks and Defenses
Просмотров 1,7 тыс.8 месяцев назад
CAMLIS 2023, Gary Lopez Munoz
Model Leeching: An Extraction Attack Targeting LLMs
Просмотров 2938 месяцев назад
CAMLIS 2023, Lewis Birch, William Hackett, Stefan Trawicki, Neeraj Suri and Peter Garraghan
Anomaly Detection of Command Shell Sessions based on DistilBERT: Unsupervised and Supervised Appro
Просмотров 1328 месяцев назад
CAMLIS 2023, Zefang Liu and John Buford
Razing to the Ground Machine Learning Phishing Webpage Detectors with Query Efficient Adversarial HT
Просмотров 658 месяцев назад
CAMLIS 2023, Biagio Montaruli, Luca Demetrio, Maura Pintor, Luca Compagna, Davide Balzarotti and Battista Biggio
Web content filtering through knowledge distillation of Large Language Models
Просмотров 928 месяцев назад
CAMLIS 2023, Tamas Voros
Compilation as a Defense: Enhancing DL Model Attack Robustness via Tensor Optimization
Просмотров 498 месяцев назад
CAMLIS 2023, Stefan Trawicki, William Hackett, Lewis Birch, Neeraj Suri and Peter Garraghan
Multi-Agent Reinforcement Learning for Maritime Operational Technology Cyber Security
Просмотров 1828 месяцев назад
CAMLIS 2023, Alec Wilson, Ryan Menzies, David Foster, Marco Casassa Mont, Neela Morarji, Esin Turkbeyler and Lisa Gralewski
Enhancing Exfiltration Path Analysis Using Reinforcement Learning
Просмотров 748 месяцев назад
CAMLIS 2023, Cheng Wang, Riddam Rishu, Akshay Kakkar, Abdul Rahman, Christopher Redino, Dhruv Nandakumar, Tyler Cody, Ryan Clark, Dan Radke and Edward Bowen
MalDICT: Benchmark Datasets on Malware Behaviors, Platforms, Exploitation, and Packers
Просмотров 1038 месяцев назад
CAMLIS 2023, Robert Joyce, Edward Raff, Charles Nicholas and James Holt
Keynote - Lessons for AI Security Preparedness
Просмотров 2508 месяцев назад
CAMLIS 2023, Shawn Richardson
Adaptive Experimental Design for Intrusion Data Collection
Просмотров 858 месяцев назад
CAMLIS 2023, Kate Highnam, Zach Hanif, Ellie Van Vogt, Sonali Parbhoo, Sergio Maffeis and Nicholas R. Jennings
Proxy in a Haystack: Uncovering and Classifying MFA Bypass Phishing Attacks in Large Scale Authenti
Просмотров 1628 месяцев назад
Proxy in a Haystack: Uncovering and Classifying MFA Bypass Phishing Attacks in Large Scale Authenti
Small Effect Sizes in Malware Detection? Make Harder Train:Test Splits!
Просмотров 618 месяцев назад
Small Effect Sizes in Malware Detection? Make Harder Train:Test Splits!
SQL Driven Infrastructure for Cybersecurity ML Operations
Просмотров 828 месяцев назад
SQL Driven Infrastructure for Cybersecurity ML Operations
FASER: Binary Code Similarity Search through the use of Intermediate Representations
Просмотров 1718 месяцев назад
FASER: Binary Code Similarity Search through the use of Intermediate Representations
Threat Detection on Kubernetes Logs Using GNN Embeddings
Просмотров 2848 месяцев назад
Threat Detection on Kubernetes Logs Using GNN Embeddings
Building a Multi-Tenant Machine Learning Workflow Orchestration Platform (CAMLIS 2022)
Просмотров 94Год назад
Building a Multi-Tenant Machine Learning Workflow Orchestration Platform (CAMLIS 2022)
OmnibusCyber: a schema-ready strongly typed database to model all cyber security objects
Просмотров 72Год назад
OmnibusCyber: a schema-ready strongly typed database to model all cyber security objects
Inroads in Autonomous Network Defence using Explained Reinforcement Learning (CAMLIS 2022)
Просмотров 239Год назад
Inroads in Autonomous Network Defence using Explained Reinforcement Learning (CAMLIS 2022)
Enhancing 2FA with IP-based geolocation without blocking all your users (CAMLIS 2022)
Просмотров 82Год назад
Enhancing 2FA with IP-based geolocation without blocking all your users (CAMLIS 2022)
Playing Cat and Mouse with the Attacker: Frequent Item Set Mining in the Registry (CAMLIS 2022)
Просмотров 102Год назад
Playing Cat and Mouse with the Attacker: Frequent Item Set Mining in the Registry (CAMLIS 2022)
Firenze: Model Evaluation Using Weak Signals (CAMLIS 2022)
Просмотров 52Год назад
Firenze: Model Evaluation Using Weak Signals (CAMLIS 2022)
Keys to the Digital Castle: Detecting Malicious MDA Device Enrollment at Scale (CAMLIS 2022)
Просмотров 50Год назад
Keys to the Digital Castle: Detecting Malicious MDA Device Enrollment at Scale (CAMLIS 2022)
Temporal Attack Detection in Multimodal Cyber-Physical Systems with Sticky HDP-HMM (CAMLIS 2022)
Просмотров 52Год назад
Temporal Attack Detection in Multimodal Cyber-Physical Systems with Sticky HDP-HMM (CAMLIS 2022)
Keynote: Lessons Learned in Red Teaming AI Systems in High-Stakes Environments (CAMLIS 2022)
Просмотров 508Год назад
Keynote: Lessons Learned in Red Teaming AI Systems in High-Stakes Environments (CAMLIS 2022)
Half-Day Vulnerabilities: A Study of the First Days of CVE Entries (CAMLIS 2022)
Просмотров 42Год назад
Half-Day Vulnerabilities: A Study of the First Days of CVE Entries (CAMLIS 2022)
Shopa chittagong panch laish khaleq dadu shohal bepul upstairs Akbar dadu pervin fufu mother's name same mine baba Azizul Haq next at Dhaka elephant road please let me know how is your parents and your other two sisters
❤
Amazing editing and story-telling - outstanding speaker. Thank you for the video!
How did you draw the loss surface?
🎯 Key Takeaways for quick navigation: 00:00 🎤 *The speaker, Mark Brighton Boach, introduces himself as a security engineer at Dropbox and discusses the topic of an AI attack called "repeated sequences."* 01:09 📦 *Dropbox is interested in AI to improve its services, including AI-powered search and question answering across Dropbox files.* 03:26 💻 *The AI/ML team at Dropbox collaborated with the security team to investigate a novel AI attack involving repeated sequences in prompts.* 05:57 🔍 *Backspaces, control characters, and meta-characters were found to produce unexpected behaviors in Transformer-based chat models.* 08:56 🕵️ *Dropbox engaged with OpenAI to address the issue but had to work on their own. They planned to open-source their findings.* 11:13 🧐 *Spaces, backslashes, and other character sequences were identified as risky inputs that could disrupt AI models.* 15:18 ⏱️ *A moderator framework was proposed to detect and block risky inputs, such as repeated sequences, to ensure model safety.* 17:32 🧰 *The repeated sequence moderator analyzes prompts for suspicious sequences and flags them based on repeat count and score.* 21:05 ❓ *The speaker addresses questions about mixing and matching special characters and discusses the potential effects.* 23:00 🤖 *The speaker considers the model's training and behavior regarding certain tokens and suggests further exploration.* 24:10 🌐 *The speaker discusses the use of UTF-8 byte order marks and Unicode characters in experiments involving repeated sequences but didn't explore Unicode bomb orderings in their work.* 25:31 📞 *A question is raised about whether prompt injection attacks are fundamentally broken and similar to phone freaking, and the speaker acknowledges the complexity of the issue without a clear solution.* Made with HARPA AIwha
🙂 *Promo sm*
So humor and excellent talk! Now I'm the fan of Nicolas Carlini
Great talk! About to head out and read the paper now. Thank you very much!
Great talk! Thanks for sharing!
Happy this guy is on our team...great advantage to not have a genius like this on the other side.
Very informative and well-organized presentation. Thank you!!
Great talk! His comparison of current state of the robustness of ML models with the crypto in 1920's is spot on.
Great talk. It's such a difficult problem that is at the heart of generalisation. The google brain paper "Adversarial Examples that Fool both Computer Vision and Time-Limited Humans" is worth a read.
Damn, this talk is epic! [ Although I disagree with his answer to the question in 39:20 'the human is the proof-of-concept that adversarial robustness exists'; humans have their own set of singular errors constrained by their visual system which gives rise to visual/optical illusions, so perhaps the goal should not be to avoid adversarial examples altogether, but to somehow align/evaluate the mistakes the machines makes with that of the human. Similar to: www.pnas.org/content/pnas/117/47/29330.full.pdf ]
An excellent talk, thank you!
only 421 views?
ruclips.net/video/otmt0-cewbc/видео.html
ruclips.net/video/MjhDkNmtjy0/видео.html
ruclips.net/video/h1BQPV-iCkU/видео.html
Activism, Human Nature, & American Repentance: Noam Chomsky interviewed by Shmuly Yanklowitz ruclips.net/video/EA7Dj-dmzEE/видео.html
Psychological Slavery Full Episode | American Black Journal ruclips.net/video/vKh0H3sg1Uo/видео.html
President John F. Kennedy's Civil Rights Address ruclips.net/video/7BEhKgoA86U/видео.html
How does this talk only has 300 views and two comments? This man's talk is absolutely brilliant.
very useful. Thank you!
great talk