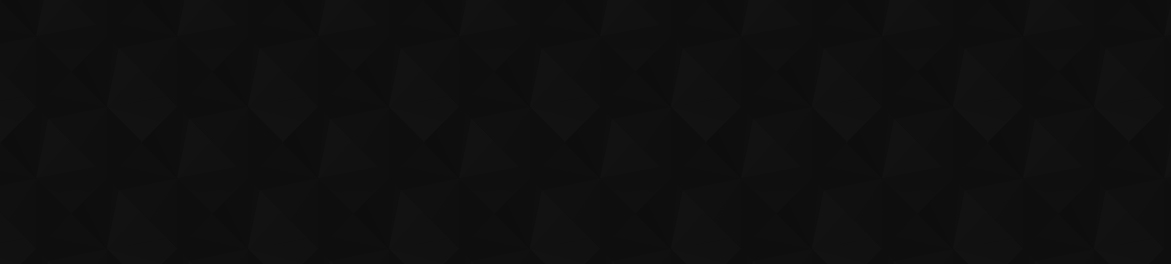
- Видео 24
- Просмотров 39 893
MLBoost
Канада
Добавлен 22 янв 2023
Join our Slack workspace for more detailed discussions: join.slack.com/t/mlboostworkspace/shared_invite/zt-2fwe33yoz-h3L8t~JynUO2pq3eXNA~qA
Our Mission: Accelerating ML Excellence through Insightful Lessons
At MLBoost, we are a platform dedicated to provoking minds with concise and insightful content about Machine Learning. Our primary objective is to empower our audience with a deeper understanding of the fundamental concepts, enabling them to tackle big problems with confidence and creativity. Through engaging videos and expert analysis, we strive to demystify the complexities of Machine Learning and make it accessible to learners at all levels. Join us on our journey of exploration, as we unravel the intricacies of this transformative field and inspire our viewers to push the boundaries of innovation.
Our Mission: Accelerating ML Excellence through Insightful Lessons
At MLBoost, we are a platform dedicated to provoking minds with concise and insightful content about Machine Learning. Our primary objective is to empower our audience with a deeper understanding of the fundamental concepts, enabling them to tackle big problems with confidence and creativity. Through engaging videos and expert analysis, we strive to demystify the complexities of Machine Learning and make it accessible to learners at all levels. Join us on our journey of exploration, as we unravel the intricacies of this transformative field and inspire our viewers to push the boundaries of innovation.
MLBoost Seminars (9): Robust Yet Efficient Conformal Prediction Sets
#ai #machinelearning #uncertainty #quantification
Imagine boosting your model's reliability even when faced with noise and adversarial attacks! Join us for another talk on conformal prediction, this time by Soroush H. Zargarbashi from CISPA Helmholtz Center for Information Security, entitled 'Robust Yet Efficient Conformal Prediction Sets' and uncover how his innovative approach extends conformal coverage guarantees to worst-case noisy scenarios.
02:56 - Will conformal coverage guarantee break when noise is iid?
14:51 - Are calibration instances clean or poisoned?
Imagine boosting your model's reliability even when faced with noise and adversarial attacks! Join us for another talk on conformal prediction, this time by Soroush H. Zargarbashi from CISPA Helmholtz Center for Information Security, entitled 'Robust Yet Efficient Conformal Prediction Sets' and uncover how his innovative approach extends conformal coverage guarantees to worst-case noisy scenarios.
02:56 - Will conformal coverage guarantee break when noise is iid?
14:51 - Are calibration instances clean or poisoned?
Просмотров: 132
Видео
MLBoost Seminars (8): Conformal Inverse Optimization
Просмотров 202Месяц назад
In this seminar, we hosted Bo Lin from The University of Toronto who presented his work on Conformal Inverse Optimization. Link to Paper: arxiv.org/abs/2402.01489 Inverse optimization has been increasingly used to estimate unknown parameters in an optimization model based on decision data. We show that such a point estimation is insufficient in a prescriptive setting where the estimated paramet...
MLBoost Seminars (7): Sequential Conformal Prediction for Time Series
Просмотров 3442 месяца назад
#ai #machinelearning #uncertainty #quantification In this MLBoost seminar, we hosted Chen Xu who presented his work on conformal prediction for time series. Here are the links to his papers: arxiv.org/abs/2403.03850 arxiv.org/abs/2212.03463 arxiv.org/abs/2010.09107 Conformal prediction for multi-dimensional time series by ellipsoidal sets Conformal prediction (CP) has been a popular method for ...
MLBoost Seminars (6): Kolmogorov-Arnold Neural Networks
Просмотров 1,1 тыс.2 месяца назад
In this seminar, we had the pleasure of hosting Ziming Liu from MIT, who presented an insightful talk on Kolmogorov Arnold Neural Networks. The seminar covered key concepts, innovative methodologies, and potential applications of this advanced neural network framework. During and following the presentation, there was a vibrant Q&A session where participants engaged with Ziming on various topics...
Uncertainty Quantification (5): Avoid these Missteps in Validating Your Conformal Codes!
Просмотров 3043 месяца назад
Link to Jupyter Notebook: github.com/mtorabirad/MLBoost/blob/main/Episode15/Episode15Main.ipynb If you find the notebook/video useful, please consider giving a star to the repository. Keywords: #machinelearning #datascience #conformalprediction #uncertainty #quantification See mapie.readthedocs.io/en/stable/examples_regression/2-advanced-analysis/plot_coverage_validity.html for how the MAPIE te...
MLBoost Seminars (5): Selection by Prediction with Conformal p-values
Просмотров 1,1 тыс.8 месяцев назад
#datascience #conformalprediction #machinelearning 🚀 Join me for another exciting presentation on Conformal Prediction! 🌟 I am hosting Ying Jin from Stanford for a presentation on the channel 🎥: “Selecting Trusted Decisions from AI Black Boxes: Correcting 🔥🔥Conformal Prediction 🔥🔥 for Selective Guarantees”. Link to the paper in the comments section below. The work studies screening procedures, ...
MLBoost Seminars (4): Uncertainty Quantification over Graph with Conformalized Graph Neural Networks
Просмотров 2,2 тыс.9 месяцев назад
Welcome to the MLBoost channel, where you always get new insights! 🌟 I am hosting Kexin Huang from Stanford University for a presentation on the channel 🎥. Kexin will present his work “Uncertainty Quantification over Graph with Conformalized Graph Neural Networks”. The work proposes conformalized GNN (CF-GNN), extending 🔥🔥 conformal prediction (CP)🔥🔥 to graph-based models for guaranteed uncerta...
MLBoost Seminars (3): Conformal Prediction for Time Series with Modern Hopfield Networks
Просмотров 1,4 тыс.9 месяцев назад
#datascience #conformalprediction #timeseries Abstract: To quantify uncertainty, conformal prediction methods are gaining continuously more interest and have already been successfully applied to various domains. However, they are difficult to apply to time series as the autocorrelative structure of time series violates basic assumptions required by conformal prediction. We propose HopCPT, a nov...
MLBoost Seminars (2): Trustworthy Retrieval Augmented Chatbots [Utilizing Conformal Predictors]
Просмотров 22310 месяцев назад
#datascience #largelanguagemodels #RAG #conformalprediction #machinelearning The recording of the talk by Shuo Li from University of Pennsylvania about his paper “TRAC: Trustworthy Retrieval Augmented Chatbot”. The work proposes a framework that can provide statistical guarantees for retrieval augmented question answering systems by combining 🔥🔥conformal prediction🔥🔥 and global testing. Link to...
MLBoost Seminars (1): Uncertainty Alignment for Large Language Model Planners
Просмотров 41311 месяцев назад
A talk by Allen the lead author of paper "Robots That Ask For Help: Uncertainty Alignment for Large Language Model Planners" Paper Abstract Large language models (LLMs) exhibit a wide range of promising capabilities from step-by-step planning to commonsense reasoning that may provide utility for robots, but remain prone to confidently hallucinated predictions. In this work, we present KnowNo, w...
Uncertainty Quantification (4B): Mastering and Implementing Split Conformal Methods with NumPy Only
Просмотров 80511 месяцев назад
#datascience #statistics #predictioninterval #conformalprediction If you find the video or its Jupyter Notebook github.com/mtorabirad/MLBoost/tree/main/Episode14 useful, please consider giving a star to the repository. Thank you! Keywords: Python-Numpy Implementation, Split Conformal Methods, Uncertainty Quantification, Coverage Validity Welcome to another MLBoost episode! In this comprehensive...
Applied Conformal Predictors: Why Large Language Models (LLMs) Need Conformal Predictors
Просмотров 1,7 тыс.Год назад
#datascience #statistics #predictioninterval #conformalprediction #largelanguagemodels "Conformal Prediction with Large Language Models for Multi-Choice Question Answering" Welcome to the first episode of our new playlist dedicated to the fascinating world of conformal predictors and their critical role in enhancing the reliability of Language Model Models (LLMs), particularly in the context of...
Uncertainty Quantification (4A): Implementing Split Conformal - Relation for Prediction Intervals
Просмотров 4,8 тыс.Год назад
#uncertainty #datascience #statistics #predictioninterval #conformalprediction #conformal In the last two episodes, we explored the concept of measuring the non-conformity of a point with a bag of points using methods like absolute errors and the conformity ladder. In this video, we delve deeper into split conformal methods, aiming to demonstrate how to add uncertainty quantification to any mod...
Uncertainty Quantification (3): From Full to Split Conformal Methods
Просмотров 4,9 тыс.Год назад
Uncertainty Quantification (3): From Full to Split Conformal Methods
Uncertainty Quantification (2): Full Conformal Predictors
Просмотров 10 тыс.Год назад
Uncertainty Quantification (2): Full Conformal Predictors
Uncertainty Quantification (1): Enter Conformal Predictors
Просмотров 7 тыс.Год назад
Uncertainty Quantification (1): Enter Conformal Predictors
(8) Training and Evaluating Point Forecasting Models: What Does and Doesn’t Make Sense!
Просмотров 193Год назад
(8) Training and Evaluating Point Forecasting Models: What Does and Doesn’t Make Sense!
(7) Under Absolute Percentage Error loss, a Non-conventional Median is Optimal!
Просмотров 238Год назад
(7) Under Absolute Percentage Error loss, a Non-conventional Median is Optimal!
(6) In ML Competitions, when the Error is MAE, Submit the Median of Inferred Distribution.
Просмотров 198Год назад
(6) In ML Competitions, when the Error is MAE, Submit the Median of Inferred Distribution.
(5) In ML Competitions, when the Error is MSE, Submit the Expected Value of Inferred Distribution.
Просмотров 509Год назад
(5) In ML Competitions, when the Error is MSE, Submit the Expected Value of Inferred Distribution.
(4) Best Possible Model May Lose to a Naive One if Evaluation Metric is Not Consistent with ...
Просмотров 248Год назад
(4) Best Possible Model May Lose to a Naive One if Evaluation Metric is Not Consistent with ...
Would you be able to provide any guidance on videos, papers, blogs etc. that are using conformal prediction for unsupervised algorithms? Thanks!
1:00
really interesting 👏👏👏👏
Thank you for the explanation, this is of high quality
Glad it was helpful!
Amazing!
Thanks!
This is great!! Thanks!
You're welcome!
Thank you for the video. However I didn’t understand the ladder about the conformity scores. You say that it “Shows the ranking of the points in the sorted non-conformity array and not the non-conformity values themselves.” But how do you sort them if not according to their non-conformity value?
You are welcome and glad to see that attention is being paid to every detail. What I mean by that sentence is that the vertical axis does not show the raw non-conformity scores - it shows the rank of a point in the sorted non-conformity array. You are correct. We need to first sort that array. For example, imagine we have only two calibration points: the first one with non-conformity = 0.5 and the second with non-conformity 0.7. Then the vertical-axis value associated with the first point will b 2 (because the rank of that point in the sorted non-conformity array is 2) and the one associated with the second point will be 1.
Amazing videos! I am wondering when the next one is coming out? How do I properly check for coverage when applying conformal predictors?
Thanks for you comment. The next video should be up early Feb. it will discuss exactly the question you asked: how to properly check for coverage!
@@MLBoost Looking forward to it! Also when you get the chance, can you also make a video about how to get prediction intervals for test case that has unknown true labels for regression problems? (i.e: Let's say I have random x values, I want to predict the y label for based on my pretrained model, how do I get prediction intervals for these predictions instead of just point predictions?)
This is already discussed in the videos. Both for Full conformal and split conformal.
I was curious if heteroskedasticity would be a problem. I looked it up and someone had the same question and found a solution. See "Distribution-Free Predictive Inference For Regression" by Lei, G'Sell, Rinaldo, Tibshirani and Wasserman (2017).
After watching this series a few times, the thing that (still) confuses me is the fact that for (100 % of training data + each new point) we have to fit a new model each time for each plausible label, but for (percentage of training data or calibration data + each new point) we don't. It looks to me that in both cases test point could be out of bag, be it full training data or e.g. 20% of training data (calibration dataset). I see that everything is the same in terms of implementing CP, but the difference is only in the number of points (training vs calibration).
I am glad to see that you have watched all the videos. In full conformal, test point is assigned a plausible label, which allows it to be considered as part of the bag; we have to refit the model so that all plausible values are considered. With split conformal however the bag consists of calibration points only.
Very interesting, thank you for the video!
Glad you enjoyed it!
Awesome video series. Thanks!
Glad you enjoyed it! Thanks for your comment!
Absolutely brilliant presentation!
if the square is a test point why the model need to be fit accounting for it? Thanks for the video
well it was answered in the next video
exactly! Thanks for your comments!
God I have sinned, of the 70th like. Pls forgive me. 🛐 Amen.
thank you!
How is this different to a quantile approach with X% confidence intervals? I guess the quantile approach would only meet some but not all of the requirements mentioned😅. Interesting stuff.
Using that approach requires one to make an assumption on the underlying distribution where as the conformal method does not. Great question! Thanks for watching!
Very good video. Thanks for making this!
My pleasure!
Excellent 👌 explanation
Thank you 🙂
also hope to cover this ??? one thing its important is that its probably is not dynamic for point, for i.e. if the loss of a point in the dataset is .098 is in 90% interval, so the other points with the same loss are also in 90% interval, but in more dynamic quantifier, a point with this loss may have 60% interval or 93% interval, I mean `conformal predictor` doesnt take to account the Uncertainty Quantification of input space, so model agnostic and distribution free are not good criteria, instead `model and distro adaptive` are better
thanks again for the question. But I am not really sure I understand what it is. Could you rephrase?
@ 8:40 ??? why these sub intervals have equal probabilities of 20%? isnt it better to assign prob with their MAE range which they take(ofc I know for last subInterval we would have a problem that it would get infinite)? what if we had another point with .16 MAE loss(note we have .15 MAE also), so it would have created another subInterval with same prob as others?
because of exchangeability as discussed @8:42
@@MLBoost first of all I thought `exchangeability of data` means that the orders of (x,y) pairs doesnt matter, I dont know where but it was in the video and I think it needs to be explained (intuitive if possible) more why exchangeability is correct, complement the explanation with my question of if there is 5 points and they have [.15, .16, .31, .46, .67] losses, why exchangeability still makes sense to assume all intervals equal probable. ofc I know in practice they might be more points and this may or may not happen but if this exchangeability and taking intervals equal probable, is a principle, so it should make sense in this case also
Great questions and I am really glad to see videos are being watched in detail. You are correct that exchangeability means order does not matter and yes that was mentioned in one of the videos. Number of points does not really matter. As long as exchangeability is satisfied intervals are equi-probable. The theoretical proof of why that is the case is in the original conformal papers or the book by original developers of the method but I may prepare a video addressing that.
@@MLBoost without any doubt these videos are top notch content so they worth to be watched carefully
so I hope in next vids we would get review on how practically the `conformal predictors` respect these criteria like 1. `coverage validity` and `efficency` are respected in conformal because the data itself is used in making intervals? 2. how we know its model agnostic? is not involving any model params, enough. also the same thing for distribution free?
Thanks for the questions. They will be covered in the next videos of the playlist.
thanks for the explanation!
You bet!
Great lecture. Thank you very much. I subscribed this channel. 🙏
Thanks and welcome!
Good work
Thank you! Cheers!
This video deserves more views and likes!
thank you!
At [8:42] I'm trying to see the bigger picture. I get that there are such edge cases where MAPE could be misleading as a function for minimizing. But, if minimizing the MAPE involves minimizing the absolute percentage error, which accounts for both under-forecasting and over-forecasting, then why it doesn't make sense to use it in business case like forecasting inventory items you've explained? It does not necessarily imply that there will be a bias towards under-forecasting or over-forecasting. That is, minimizing the MAPE does not necessarily imply that there will be a lot of under-forecasting or a lot of over-forecasting. Maybe we should get a step further and whilst minimizing MAPE we explicitly state a bias towards over-forecasting using some kind of "weighted MAPE", and thus reducing the number of under-forecasting values. Something like that?
Thanks for the question. I will get back to you asap.
@@MLBoost In your time!
amazing!
Thanks!
My reply to a question raised in an earlier comment: Question: “3) Why are you talking about how to 'cheat' such metrics in this (in my opinion) unrealistic situation (where you have access to the underlying distribution). What if an average of all 3 metrics is used? Do these assumptions still hold if the interval on which the evaluation is done increases? What if the evaluation is done on a test set twice as big as the known train set?” The discussion is important even when one does not have access to the underlying distribution because, even then, one typically needs to evaluate and rank different and competing forecasting methods (ex. tree-based vs. neural networks), which requires deciding on which evaluation metric to use (ex. MSE vs. MAE). For example, imagine a scenario where we have two competing models A and B. The MSE of model A is lower than that of model B but the MAE of model A is higher. So if we use MSE as an evaluation metric, then model A is the better model, but if we use MAE then model B will be the better one. So, the question is which metric should we use for evaluation? It is important to base our evaluation on a metric that properly targets the point from the underlying and unknown distribution that is more important for us. For example, if, for the business case at hand, predicting the mean is more important than the median, then, model A is a better one for that business case. Different evaluation metrics can be combined but one should be aware that by doing so a non-conventional point from the underlying distribution is then being targeted. In the example above if predicting the median and mean are both important, then it would make sense to use some combination of MSE and MAE as the evaluation metric. And yes the arguments stated in the video (ex. mean is optimal under SE) will hold even if you increase the evaluation interval. But if you significantly shorten the interval they may not hold.
My reply to a question raised in an earlier comment: Question: “2) Once such an expert has access to the underlying data distribution, why aren't all these error metrics set to zero since they can perfectly predict the future data point? -> I assume that this is because it's an random process and you don't have a y(t+1) = f(y(t)) deterministic relationship, with 'f' being the distribution.” Yes, and let’s also keep in mind that these metrics are point ones, meaning that to evaluate each one has to pick a point from the distribution.
Reply to the question raised in an earlier comment: “1) How would an 'expert' given only the data point correctly infer the data distribution?” That will require the expert to build an error-free model. For complicated cases such as the one discussed in the episode, I am not sure that would be possible in practice. However, the point of this series of episodes is to highlight that different evaluation metrics (MAE, MSE, MAPE, etc.) get their minimum values with different points from the distribution. When one performs point forecasting for a phenomenon that is inherently probabilistic, which is the most common real-life forecasting setting, even after one builds the model, so much care still needs to be given to select the right point. I believe the former (building model) typically receives enough attention but the latter does not. One may reasonably argue that, ok if the task is point forecasting, why should we build a probabilistic model? And the answer is that when you build a point-forecasting model, the point from the underlying, unknown distribution you are training for (i.e., the training loss) should be consistent with the evaluation metric. I will discuss this point in more detail in the upcoming episodes. Hope this answers your first question. Looking forward to follow-up comments if any. Thanks for reading!
I'm trying to follow these videos, but they're a bit out of my depth. Could you explain a few things? 1) How would an 'expert' given only the data point correctly infer the data distribution? 2) Once such an expert has access to the underlying data distribution, why aren't all these error metrics set to zero since they can perfectly predict the future data point? -> I assume that this is because it's an random process and you don't have a y(t+1) = f(y(t)) deterministic relationship, with 'f' being the distribution. 3) Why are you talking about how to 'cheat' such metrics in this (in my opinion) unrealistic situation (where you have access to the underlying distribution). What if an average of all 3 metrics is used? Do these assumptions still hold if the interval on which the evaluation is done increases? What if the evaluation is done on a test set twice as big as the known train set?
Thanks for your great questions. Below are my answers to each in a separate comment and I look forward to follow-up questions if any.
How fortunate we are that center of mass exists in a Hookean universe.
Yes, indeed!
very informative! I like the questions presented around 3:43.
Thank you!
Hi Mahdi, I like your videos very much! They are very well structured and well referenced by academic papers. What I would like to discuss here, is related to the material presented after 8:39 in your video. Two tables are presented on when to use MAPE vs. adjusted MAPE. Can you please elaborate on the "Outlier Impact on Cost" case? I would like to know more about cases where that impact is limited vs. unlimited. What I am trying to understand deeper is how one should decipher whether the impact of an outlier on cost is limited or unlimited in his/her case. Thank you in advance!
Hi Farzad, I am glad you like the videos, thanks for your nice words, and so sorry for such a delay in my reply. Let's first note that the entity that will use forecasts to make business decisions will incur an economic cost (penalty measured in $) because our forecasts will have an error. Imagine we have multiple forecasts, where one (i.e., the outlier) is very bad but the others are decent or good. The question is how the error of that single outlier (e_out) increases the penalty that the entity will incur. One can think of two scenarios. Scenario A: the higher e_out the higher the penalty; here, the economic cost of the single bad forecast can overshadow the benefits of the other good forecasts. Scenario B: as e_out increases, the rate by which e_out increases the penalty diminishes. Therefore, a single bad forecast will decrease the benefits of the other good forecasts but it cannot totally diminish them. Let me give two examples: Suppose you have a financial investment firm that uses forecasts to guide their investment decisions. They make seven forecasts for different stocks, and six of them turn out to be accurate or close to the real values. However, one forecast for a high-risk stock turns out to be significantly bad, resulting in a substantial loss. Here, the higher the latter loss, the higher the penalty for the firm. In other words, this single bad forecast could lead to a large financial loss that far exceeds the gains made from the accurate forecasts for the other stocks. In this case, the economic cost of the bad forecast overshadows the benefits of the other good forecasts. Consider a retail store that uses forecasts to predict customer demand for different products. They make seven forecasts for the upcoming week, and six of them are accurate or close to the real values. However, one forecast for a seasonal product turns out to be significantly bad, resulting in excess inventory. While there might be a cost associated with holding excess inventory, it is highly unlikely that it will overshadow the benefits gained from the accurate forecasts for the other products. The store can still sell the excess inventory over time or offer discounts to clear it, minimizing the impact on its overall profitability. In this case, the economic cost of the bad forecast does not overshadow the benefits of the other good forecasts. I hope this has clarified your question! If you have further questions, please let me know. Thank you!
Great and clear explanation! Helped me further, thanks! 👏🏻
Glad it helped!
This is a great video, keep it up, please! I have a question: Around 7:54 in the video while you are explaining under-forecasting and over-forecasting examples in the table, why are we swapping the values of actuals and forecasts? I was thinking why are not we considering this scenario in which under-forecasting is (Actual 150, Forecast 100) and over-forecasting is (Actual 150, Forecast 200). What I am trying to say is, if we go with the scenario mentioned here, MAPE doesn't change. So, does the definition of a counterpart in this context mean the actual and forecasted values should be swapped in order to compare under and over-forecasting in a given time point?
Thank you Farzad for such a great question and for your interest watching the video. You are absolutely right! If we swap the values the way you mentioned, MAPE will not change. Great observation! And you are right about the definition of counterpart. I have discussed this issue in this second video titled adjustedMAPE. Cheers!
@@MLBoost Thanks again! Just realized you posted the adjusted MAPE video right after writing the previous question.
I hope you cover distributional prediction
Thanks for watching the video and leaving a comment. That topic is on the agenda.
very helpful content. keep the good work!
Thanks, will do!
Great video! I found you through LinkedIn and found the video really informative. Although I knew MAPE is weighted based on actual value, I hadn’t ever considered the fact that this leads to a heavier penalty on positive errors. As far as feedback, I think your graphics and the sound are all really good. I would say the structure of the video could use improvement. For example, I would prefer you start the video with an explanation of when MAPE is useful and discuss its use cases. I felt the video jumped into the limitations before we fully got to see why it’s useful. It sort of reminded me of a linear algebra proof where the professor only discusses the preconditions required for the proof that by then end of the class It’s hard to remember what the actual proof was for. All in all, a really quality video and I’ve subscribed. Also a small other point, but when first looking at the thumbnail it gave me the impression that this is one of those ads stating that MLBoost is a package (pretty cool name for a package though imo) that you can calculate time series with. I typically avoid anything like that and since this video is informative I would consider changing the title to something more explanatory.
Thank you for such a detailed comment. I am still in the process of figuring out if what I am doing here adds any value to the community and comments like this are certainly very encouraging. I will certainly keep them in mind for the next videos. Thank you again!
Question 2: How much of the content presented in the video was new to you, and how much did you already know?
Question 1: Did the video provide you with new insights on MAPE, or did you already know about it? If you did gain new insights, what were they?