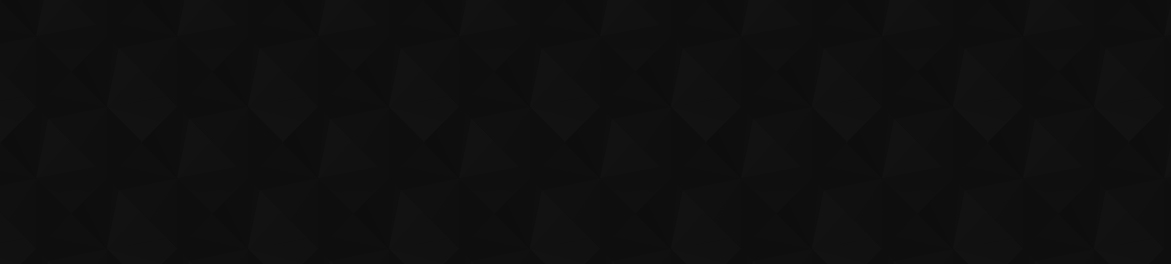
- Видео 19
- Просмотров 93 712
Generable
США
Добавлен 7 июл 2016
The latest advances in Bayesian computing allow users to estimate models that were previously out of reach for most practitioners in industry and academia. Today, we are able to fit models with full Bayesian inference that jointly estimate hundreds of thousands and sometimes millions of parameters. As model complexity grows, we need tools to make sense of these models so we can better understand their strengths and more importantly their weaknesses. By analogy, if we are building a plane, it is our responsibility to test under which conditions it can and cannot fly. We owe this much to our p̶a̶s̶s̶e̶n̶g̶e̶r̶s̶ users.
In this channel, we are focusing on understanding and explaining uncertainty in the broadest sense of the word including interesting model structures, model inferences, predictions, causal inference, decision analysis, and communicating models and uncertainty.
In this channel, we are focusing on understanding and explaining uncertainty in the broadest sense of the word including interesting model structures, model inferences, predictions, causal inference, decision analysis, and communicating models and uncertainty.
Duco Veen: On using expert information in Bayesian statistics
Duco Veen is an Assistant Professor at the Department of Global Health situated at the Julius Center for Health Sciences and Primary Care of the University Medical Center Utrecht. In that capacity, he is involved in the COVID-RED, AI for Health, and Trials@Home projects. In addition, he is appointed as Extraordinary Professor at the Optentia Research Programme of North-West University, South Africa. Duco works on the development of ShinyStan and has been elected as a member of the Stan Governing Body.
In this talk, Duco will discuss how expert knowledge can be captured and formulated as prior information for Bayesian analyses. This process is also called expert elicitation. He will highlig...
In this talk, Duco will discuss how expert knowledge can be captured and formulated as prior information for Bayesian analyses. This process is also called expert elicitation. He will highlig...
Просмотров: 376
Видео
Juho Timonen: Design of Statistical Modeling Software
Просмотров 2142 года назад
Abstract Juho presents what he thinks is an ideal modular design for statistical modeling software, based on his experiences as a user, developer, and researcher. He looks at the structure of existing software, focusing on high-level user interfaces that use Stan as their inference engine. Juho uses the longitudinal Gaussian process modeling package lgpr and its future improvements to demonstra...
Jonathan Auerbach: Could voting restrictions be increasing election fraud?
Просмотров 2522 года назад
In this talk, Jonathan will present some research he conducted as the 2020-2021 Science Policy Fellow at the American Statistical Association. The talk will review several projects before focusing on the title question, which Science Policy Director Steve Pierson and Jonathan write about in a recent publication for Statistics and Public Policy. The abstract is summarized below. Jonathan will co...
Lizzie Wolkovivh: Predicting future forest tree communities and winegrowing regions with Stan
Просмотров 2832 года назад
Climate change is having large impacts on natural and agricultural systems around the globe. Mitigating the worst consequences requires models that mechanistically predict changes. Towards that goal, the Temporal Ecology Lab works on models to better predict the most reported biological impact - shifts in phenology, the timing of recurring life history events such as leafout, and flowering. Her...
Rok Češnovar: The Current State and Evolution of Stan
Просмотров 6692 года назад
We will present the current state of the Stan ecosystem, highlight some of the advances that improved the performance of Stan in the past few years, and discuss what is still to come in the not-so-distant future. You will hear about the core modules of Stan, how they all fit together, and how the various interfaces bring that core to life in different ways to make your Stan models run. If your ...
Lester Mackey: Kernel Thinning and Stein Thinning
Просмотров 5213 года назад
Abstract This talk will introduce two new tools for summarizing a probability distribution more effectively than independent sampling or standard Markov chain Monte Carlo thinning: Given an initial n point summary (for example, from independent sampling or a Markov chain), kernel thinning finds a subset of only square-root n points with comparable worst-case integration error across a reproduci...
Uri Shalit: Towards responsible patient-level causal inference: taking uncertainty seriously
Просмотров 7933 года назад
Topics: Calibrated Webcast, Bayesian Inference, Causal Inference, Machine Learning, Healthcare About the speaker Uri Shalit is an Assistant Professor in the Faculty of Industrial Engineering and Management at Technion University. He received his Ph.D. in Machine Learning and Neural Computation from the Hebrew University in 2015. Prior to joining Technion, Uri was a postdoctoral researcher at NY...
Elea Feit: A Gaussian Process Model for Response Time in Conjoint Surveys
Просмотров 3633 года назад
Choice-based conjoint analysis is a widely-used technique for assessing consumer preferences. By observing how customers choose between alternatives with varying attributes, consumers' preferences for the attributes can be inferred. When one alternative is chosen over the others, we know that the decision-maker perceived this option to have higher utility compared to the unchosen options. In ad...
Jessica Hullman: Theories of Inference for Data Interactions
Просмотров 9403 года назад
Research and development in computer science and statistics have produced increasingly sophisticated software interfaces for interactive and exploratory analysis, optimized for easy pattern finding and data exposure. But design philosophies that emphasize exploration over other phases of analysis risk confusing a need for flexibility with a conclusion that exploratory visual analysis is inheren...
Tamara Broderick: Fast Discovery of Pairwise Interactions in High Dimensions using Bayes
Просмотров 9583 года назад
Discovering interaction effects on a response of interest is a fundamental problem in medicine, economics, and many other disciplines. In theory, Bayesian methods for discovering pairwise interactions enjoy many benefits such as coherent uncertainty quantification, the ability to incorporate background knowledge, and desirable shrinkage properties. In practice, however, Bayesian methods are oft...
Paul Bürkner: An introduction to Bayesian multilevel modeling with brms
Просмотров 16 тыс.3 года назад
The talk is about Bayesian multilevel models and their implementation in R using the package brms. It starts with a short introduction to multilevel modeling and to Bayesian statistics in general followed by an introduction to Stan, which is a flexible language for fitting open-ended Bayesian models. We then explain how to access Stan using the standard R formula syntax via the brms package. Th...
Aki Vehtari: On Bayesian Workflow
Просмотров 4,8 тыс.3 года назад
We discuss some parts of the Bayesian workflow with a focus on the need and justification for an iterative process. The talk is partly based on a review paper by Gelman, Vehtari, Simpson, Margossian, Carpenter, Yao, Kennedy, Gabry, Bürkner, and Modrák with the following abstract: "The Bayesian approach to data analysis provides a powerful way to handle uncertainty in all observations, model par...
Charles Margossian: Some Outstanding Challenges when Solving ODEs in a Bayesian context
Просмотров 7543 года назад
Many scientific models rely on differential equation-based likelihoods. Some unique challenges arise when fitting such models with full Bayesian inference. Indeed as our algorithm (e.g. Markov chain Monte Carlo) explores the parameter space, we must solve, not one, but a range of ODEs whose behaviors can change dramatically with different parameter values. We will present two examples where thi...
Kristian Brock: Functional uniform priors for dose-response models
Просмотров 2473 года назад
Dose-response modeling frequently employs non-linear regression. Functional uniform priors are distributions that can be derived for parameters that convey approximate uniformity over the range of function shapes generated by the model. They provide a stark alternative to regular uniform priors, which in the non-linear setting can provide potentially undue influence on the estimated functional ...
Arman Oganisian: Introduction to Nonparametric Bayes
Просмотров 1,4 тыс.4 года назад
Bayesian nonparametrics combines the flexibility often associated with machine learning with principled uncertainty quantification required for inference. Popular priors in this class include Gaussian Processes, Bayesian Additive Regression Trees, Chinese Restaurant Processes, and more. But what exactly are “nonparametric” priors? How can we compute posteriors under such priors? And how can we ...
Matthew Kay: Uncertainty visualization and Bayes
Просмотров 2,1 тыс.4 года назад
Matthew Kay: Uncertainty visualization and Bayes
Jacqueline Buros: Predicting survival from early tumor data in oncology clinical trials
Просмотров 7324 года назад
Jacqueline Buros: Predicting survival from early tumor data in oncology clinical trials
Benjamin Goodrich: Introduction to Bayesian Computation Using the rstanarm R Package
Просмотров 12 тыс.8 лет назад
Benjamin Goodrich: Introduction to Bayesian Computation Using the rstanarm R Package
Andrew Gelman: Introduction to Bayesian Data Analysis and Stan with Andrew Gelman
Просмотров 50 тыс.8 лет назад
Andrew Gelman: Introduction to Bayesian Data Analysis and Stan with Andrew Gelman
Wondered why we have '-1' in "2 * Phi(asin((R - r) / sigma) - 1" in the golf example model. (Min 51:38)
Anyone know why loo_predict(blm) and predict(blm[-obs.,],data[obs,]) are giving me a predicted odds of 0.28 and 0.8 respectively? These estimates are so far apart. “0.8” seems more accurate to me but the events true outcome was “0” so loo_predict did a better job. Does loo_predict just not work with high Pareto values, is that why??
I came across this after I was explaining square root and exponents equating to 2d and 3D structures. As I understand it a 4d anything would appear as a 3D object to us. However, though we cannot see it, are we able to interact? If so how would we measure those interactions. Further more, the interest in tools that seemingly fit this standard to measure that in which we can not perceive.
How to consider priors in Bayesian regression with some data
Dont know
She’s awesome
Lmfao this guys so funny
Just beginning to learn about Bayesian analysis ... thanks for the great video and everyone for links in comments ... Question: Is it correct to say that, in the world cup example 1, the only variables that are calculated by Stan are: b real, sigma_a >=0, and sigma_y >=0? In other words, Stan figures out (simultaneously/jointly): (1) the best b and sigma_a for the equation a = b*prior_scores + sigma_a*[eta_a ~ N(0,1)] (2) the best sigma_y so that student_t(df = 7, a[team_1] - a[team_2], sigma_y) best predicts ~ sqrt_adjusted[score(team_1) - score(team_2)] That seems kind of weird to me that after we figure out the formula for a, it kind of boils down to just one parameter = sigma_y
Thanks for this! Most interesting and useful
Wow! Brilliant - this really helped me a lot. Thank you.
Contain information would been shared ,👍🏻
Is it possible to include a factor variable in the model? If yes any examples please.
Most excellent!!!
Yeah, he Roks
Great
What's with the blurred box in the bottom of the slides?
There was a pop-up on Lizzie's screen that we had to edit out.
As a clinician treating patients CATE (conditional average treatment effect) is not adequate. What is needed is conditional treatment effect distribution (C-TED). We need to know what the risk of bad outcomes is, for each treatment option, including varying the timing, dose and protocol. We need to know if the tails of the C-TED can be anticipated, detected, and mitigated. For this reason we don't want to compute average outcomes, we need to propagate distributions through the model, from the observed variables, through the hidden (latent) variables to the outcomes. Knowing the shape of the distribution is critically important. In pathophysiology and therapeutics the causal effects may often be non-linear.
Very good explanation, Thanks!
That was beautiful - Thank you for the wonderful package, Paul!
Gelman is quite nice to listen to. His RL voice sounds different from his blog voice somehow
(1:35) "A lot of us...a lot of us are." The melancholy of that statement was so tangible :)
Thank you so much for building bmrs. You saved my master thesis, got me into Bayesian statistics and made me learn R, which is now a staple tool of my professional career.
Very valuable package indeed! I'm wondering how to model the covariance structure in a bayesian longitudinal setting, similar to covariance patterns such as compound symmetry, autoregressive, Topelitz etc. in the frequentist world. In the frequentist world, taking serial correlation into consideration narrows the confidence intervals of the parameters. How to model the covariance structure in a bayesian longitudinal setting? I'm wondering if a bayesian intercept always introduces compound symmetry, similar to a random intercept in a frequentist linear mixed effects model? I suspect taking serial correlation would narrow the posterior distributions of the model parameters, strengthening the bayesian inference. However, I'm not at all sure if my thoughts are anywhere near correct. The brms package is a very valuable resource. However, the parts about covariance structures seem to be still in progress. If anyone has good theoretical (and why not practical) bayesian references regarding these covariance modeling issues (serial correlation etc.), I would appreciate them very much.
What was the bug he fixed? I want to know how he solved the problem.
There was a factor of 2 missing. Full story is here: statmodeling.stat.columbia.edu/2014/07/15/stan-world-cup-update/
I know this is a rather old video but it is still highly relevant and useful. At 47:02 I don't think a standard EV calculation really does that situation justice. With high payout/low loss situations like that I think it is better to weight the payouts by their utility. For example losing $10 may have basically no subjective utility loss when compared to the subjective utility gained from having $100k. Lets say that to me having $100k has 20k times as much ultility as losing $10 does. When you switch from an ev calculation based on the win/loss to a subjective ultility of the payouts there is a drastic increase in the EV(although still negative in this case). e.g: win=10000 dollars lose = 10 dollars (win*5.4e-06)-((1-5.4e-06)*lose)= ~-$9.46 dollars win_util = 20000 utility points or "utils" lose_util = 1 utils (win_util*5.4e-06)-((1-5.4e-06)*lose_util)=-0.89 utils This is a simple example and we could for sure argue about the subjective utility values but I think overall it shows that the normal EV calculation doesn't really do the situation justice when you think about the utility of the win versus the utility of the loss. One could also flip this around and talk about the subjective utility of losing samples versus winning samples. Like say this was overall +ev but that the subjective value in winning so rarely was less than the subjective value loss from losing so often. I got this concept from game theory. There are plenty of examples, especially in poker, where doing something that is -ev right now could lead to a +ev situation later on. Poker players call that implied ev and an example could be calling with some sort of draw when the current raw pot odds don't justify it but you know that when you do make your hand that the profits gained will make up for the marginal loss now. So for example lets say I have some idea for a product or service that would earn $50k a year off a $100k investment. With using a fairly standard 10x income for valuation estimation I could say the subjective utility of winning that 100k is actually worth 50k utility points versus the 10k utility points implied by an even weighting. This specific situation would still be -ev though. All of that leads me down the path of seriously doubting most of rational economics.
and even just going with constant valuation of money, you get _multiple entries_. So, sure, the probability of 1 contribution winning is whatever he got, but you can submit multiple submissions whose combination could definitely lead to a win. especially, when you consider that you can make about 10k submissions. made a little sad to see that reductive analysis (and with all the floating) when I was excited to see a really interesting decision problem at hand :/
At 16:27 you talk about checking predictive posterior distributions for games against their actual results to check if they are within their respective 95% CIs. Are these games training data or unseen data?
He didn't say they were held out samples. So probably they were in the training data. Ideally you shouldn't do that. Because it would hide overfitting. However bayesian methods are much less prone to overfitting. In his example he found some completely different problem.
@@Houshalter thanks
@@Houshalter I've heard people argue that bayesian methods don't overfit but the developers sometimes do have incorrect assumptions about priors and their distributions which can lead to situations that may look similar to overfitting in the classic sense of the word. For example say we naively look at some time series data and we think we have a solid basic understanding of the distributions of the processes that formed that data. We fit a model and it seems to do well on the trainging set but fails pretty horribly when testing on unseen data. There are many reasons this could happen and almost all of them are based on the fact that our training sample didn't include enough data to really estimate the distributions and their parameters, we picked the wrong distributions/priors for those processes or that the processes that generate our data vary over time.
@@crypticnomad bayesian can absolutely suffer from a bad model. But it's a different problem than normal overfitting. And a validation test would not necessarily show any difference from the training set
"Soccer games are random, it all depends how good the acting is"
Also really liked the golf putt example.
Thanks for this. Any link to the slides?
www.dropbox.com/s/sfi0pcf7hais91r/Gelman_Stan_talk.pdf?dl=0 This (for given slides) talk was given in Aalto university, October 2016.
Prof. Gelman: At 19:00 you talk about checking how the model fits the data; are there any tools in Stan to avoid overfitting?
There are no "tools", but this may help: andrewgelman.com/2017/07/15/what-is-overfitting-exactly/
Thanks Dr. Gelman
That golf putting model is just about the coolest thing ever.
Thanks a lot for making this code available for download. That was really helpful for getting started in Stan.
where is the code??
Thanks for the great presentation and explanations on real models. This made me laugh: "working with live posterior"
The data set from (bit.ly/lc-loans) was modified for this video illustration. Could you provide the modified one that i can follow? Thanks
There is CSV file in this directory: bit.ly/rstanarm-share
Hi, using the code in your Google drive folder I only get 2,964 observations. When I drop the ,fileEncoding = "latin1" portion of the get_data.R script and then run the on slide 12 of the presentation I get 38,607.
Nice fix, I get 41,279 but maybe dataset has since increased.