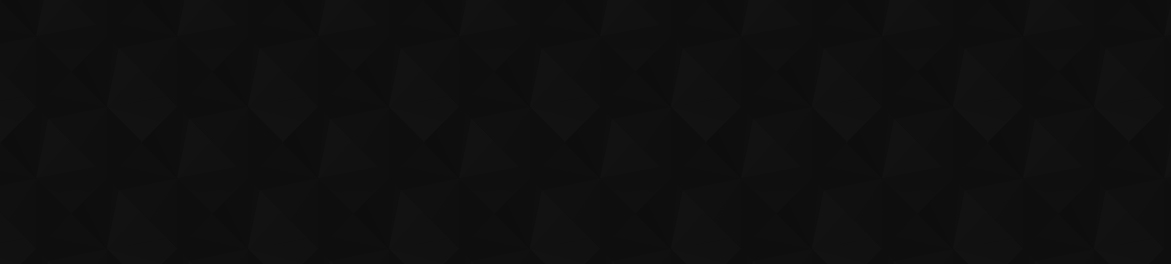
- Видео 139
- Просмотров 167 698
valgrAI
Испания
Добавлен 22 июл 2022
Valencian Graduate School and Research Network of Artificial Intelligence.
ValgrAI is a non-profit foundation of the Generalitat Valenciana (Conselleria de Innovación, Universidades, Ciencia y Sociedad Digital), formed by a community of researchers, professors, companies, students, and alumni who share the same objective: to create value for the society with rigorous work and social responsibility through pioneering research and education in Artificial Intelligence.
ValgrAI integrates the best AI researchers from the Valencian Community, joining efforts to more efficiently address AI's great scientific and technological challenges and mobilize the industry to integrate and find synergies with the research teams. ValgrAI will facilitate the transfer of results from your researchers to your partner companies.
ValgrAI is a non-profit foundation of the Generalitat Valenciana (Conselleria de Innovación, Universidades, Ciencia y Sociedad Digital), formed by a community of researchers, professors, companies, students, and alumni who share the same objective: to create value for the society with rigorous work and social responsibility through pioneering research and education in Artificial Intelligence.
ValgrAI integrates the best AI researchers from the Valencian Community, joining efforts to more efficiently address AI's great scientific and technological challenges and mobilize the industry to integrate and find synergies with the research teams. ValgrAI will facilitate the transfer of results from your researchers to your partner companies.
Acelera tus proyectos I+D | Webinar de innovación
🌟 Aprende cómo la IA está revolucionando la creación de contenidos en áreas como redacción, diseño gráfico, producción audiovisual, composición musical y modelado 3D. Descubre técnicas para generar contenido de calidad en tiempo récord, personalizar masivamente para diversas audiencias, y optimizar tu visibilidad online. Ideal para creadores de contenido digital, profesionales de marketing, diseñadores, escritores, productores audiovisuales y educadores que deseen liderar en la era digital. Accede ahora y no te pierdas la oportunidad de estar a la vanguardia de la innovación creativa!
Просмотров: 55
Видео
Desata tu creatividad - Webinar de innovación
Просмотров 29Месяц назад
🚀 Descubre el poder de la Inteligencia Artificial Generativa en nuestro próximo webinar gratuito. Sumérgete en el universo de la IA y explora cómo puede revolucionar la creación de contenidos en múltiples disciplinas como la redacción, diseño gráfico, producción audiovisual, composición musical, y modelado 3D. Aprende a impulsar tu productividad, personalizar contenidos para audiencias diversas...
XII Matinal de Investigación - Asignación de estados cerebrales dinámicos con redes de estados eco
Просмотров 37Месяц назад
Wael el-Deredy explora el uso de Redes de Estados Eco (ESN) para asignar estados cerebrales dinámicos a partir de datos de neuroimagen. Esta investigación busca capturar patrones temporales en datos de EEG, reproduciendo dinámicas cerebrales no lineales y proporcionando una representación compacta de la evolución de señales cerebrales.
XII Matinal de Investigación - Identificación de estereotipos en textos y memes
Просмотров 36Месяц назад
Elias Urios Alacreu presenta su TFM sobre sistemas de aprendizaje profundo para identificar y categorizar estereotipos raciales en textos y sexistas en memes. Utilizando un enfoque de aprendizaje por desacuerdo, la investigación integra perspectivas diversas de anotadores para mejorar la generalización de los sistemas, destacando la importancia del contexto en la detección de estereotipos.
Transforma tu perfil tecnológico | Webinar de innovación ValgrAI
Просмотров 40Месяц назад
🚀 Descubre cómo la IA puede revolucionar tu carrera en tecnología. En este webinar gratuito, exploramos las últimas innovaciones en Inteligencia Artificial y su impacto en el sector TIC. Aprende a desarrollar soluciones basadas en IA, desde mejorar productos existentes hasta crear innovaciones disruptivas. Ideal para desarrolladores, ingenieros y profesionales TIC que buscan evolucionar de usua...
Integra la inteligencia artificial en RRHH | Webinar de innovación ValgrAI
Просмотров 65Месяц назад
Descubre cómo la Inteligencia Artificial está transformando la gestión de Recursos Humanos en este webinar práctico. Aprenderás sobre las últimas innovaciones en IA aplicadas a RRHH, desde reclutamiento hasta desarrollo del talento. Exploraremos casos de uso reales, beneficios clave y consideraciones éticas. Únete a nosotros para obtener insights valiosos sobre cómo integrar la IA en tus proces...
Revolución Educativa: Claves para aplicar IA en el aula | Webinar de innovación ValgrAI
Просмотров 1352 месяца назад
¡Descubre cómo la Inteligencia Artificial está transformando la educación! En este webinar de ValgrAI, y a manos de nuestro Coordinador docente Jordi Linares, exploramos las claves para aplicar la IA en el aula y revolucionar la enseñanza. Aprenderás sobre: • Fundamentos de la IA en educación • Casos de éxito en escuelas y universidades • Cómo la IA está cambiando la adquisición y transmisión d...
XI Matinal de Investigación | Invarianza de Métricas de Calidad de Imagen a Transformaciones Afines
Просмотров 182 месяца назад
En esta presentación del XI Matinal de Investigación de ValgrAI, Nuria Alabau, investigadora de la Universitat de València, revela un estudio innovador sobre las métricas de calidad de imagen en relación con las transformaciones afines. Alabau explica por qué, a pesar de que las arquitecturas profundas son líderes en la predicción de la calidad subjetiva de imágenes, los métodos actuales de eva...
XI Matinal de Investigación | Explicabilidad en Deep Learning para Problemas Espacio-Temporales
Просмотров 242 месяца назад
En esta presentación del XI Matinal de Investigación de ValgrAI, Javier García Siguenza, investigador de la Universitat d'Alacant, aborda la crucial importancia de la Inteligencia Artificial Explicable (XAI) en la sociedad actual. García Siguenza explora cómo la IA está transformando diversos sectores, desde la industria hasta la toma de decisiones, y por qué esto ha aumentado la preocupación p...
Understanding LLM via Mechanistic Interpretability - Juan Carlos Trujillo - IV Encuentro UMI
Просмотров 523 месяца назад
Title: Understanding Large Language Models via Mechanistic Interpretability Prof. Juan Carlos Trujillo from Universitat d'Alacant breaks down the complex world of large language models through mechanistic interpretability. This presentation highlights the need for deeper understanding and transparency in the functioning of AI models.
AI-Powered Brain-Machine Interfaces for Gait Assistance with..- José María Azorín - IV Encuentro UMI
Просмотров 193 месяца назад
Title: AI-Powered Brain-Machine Interfaces for Gait Assistance with Lower-Limb Exoskeleton José María Azorín from Universidad Miguel Hernández de Elche discusses cutting-edge brain-machine interfaces for gait assistance using lower-limb exoskeletons. Explore the intersections of AI, neuroscience, and rehabilitation technology.
Archetypal Analysis: Learning Through Extreme Profiles - Irene Epifanio - IV Encuentro UMI
Просмотров 253 месяца назад
Irene Epifanio from Universitat Jaume I introduces archetypal analysis and its applications in industrial design. Learn about the role of extreme profile analysis in understanding complex data patterns and improving design methodologies.
The Challenge of Deciphering Language - Amparo Alcina - IV Encuentro UMI
Просмотров 133 месяца назад
Join Amparo Alcina from Universitat Jaume I as she presents her research on ontological modeling of language and linguistic knowledge representation. Discover how computer tools enhance translation and terminography in professional settings.
Machine Teaching for XAI - César Ferri - IV Encuentro UMI
Просмотров 203 месяца назад
César Ferri from Universitat Politècnica de València explores machine teaching techniques for explainable AI. This video covers how algorithms can justify their decisions, especially in critical contexts, promoting transparency and accountability in AI systems.
An intelligent transport system for safe route recommendation - Vicente R. Tomás - IV Encuentro UMI
Просмотров 53 месяца назад
Explore the innovative intelligent transport system developed by Vicente R. Tomás from Universitat Jaume I de Castelló. This video delves into the use of AI for recommending safe travel routes, enhancing transportation technology and route safety.
Hiroaki Kitano | Nobel Turing Challenge: Creating the Engine for Scientific Discovery - #VSCF2024
Просмотров 2243 месяца назад
Hiroaki Kitano | Nobel Turing Challenge: Creating the Engine for Scientific Discovery - #VSCF2024
Technological Innovation for Home Autonomy of Dependent Persons with AI- The Future of AI #VSCF2024
Просмотров 444 месяца назад
Technological Innovation for Home Autonomy of Dependent Persons with AI- The Future of AI #VSCF2024
Bias Mitigation in Corpora for LLMs Training Applied to Text... - The Future of AI - #VSCF2024
Просмотров 194 месяца назад
Bias Mitigation in Corpora for LLMs Training Applied to Text... - The Future of AI - #VSCF2024
Inspection, Maintenance, and Repair of Underwater Structures - The Future of AI - #VSCF2024
Просмотров 134 месяца назад
Inspection, Maintenance, and Repair of Underwater Structures - The Future of AI - #VSCF2024
Anomaly Detection Based on Artificial Intelligence of Things... - The Future of AI - #VSCF2024
Просмотров 254 месяца назад
Anomaly Detection Based on Artificial Intelligence of Things... - The Future of AI - #VSCF2024
Few-shot Adaptation of Vision-Language Models for Slide-level... - The Future of AI - #VSCF2024
Просмотров 384 месяца назад
Few-shot Adaptation of Vision-Language Models for Slide-level... - The Future of AI - #VSCF2024
Explainable Earth Surface Forecasting Under Extreme Events - The Future of AI - #VSCF2024
Просмотров 54 месяца назад
Explainable Earth Surface Forecasting Under Extreme Events - The Future of AI - #VSCF2024
Advancements in Neural Machine Translation for Historical Document... - The Future of AI - #VSCF2024
Просмотров 124 месяца назад
Advancements in Neural Machine Translation for Historical Document... - The Future of AI - #VSCF2024
AI Techniques Applied to Video Game Technology Development - The Future of AI - #VSCF2024
Просмотров 264 месяца назад
AI Techniques Applied to Video Game Technology Development - The Future of AI - #VSCF2024
Estimation of Potentially Traversable Free Zones for Autonomous Robots - The Future of AI #VSCF2024
Просмотров 224 месяца назад
Estimation of Potentially Traversable Free Zones for Autonomous Robots - The Future of AI #VSCF2024
Enhancing CBIR Performance in Prostate Cancer Diagnosis... - The Future of AI - #VSCF2024
Просмотров 114 месяца назад
Enhancing CBIR Performance in Prostate Cancer Diagnosis... - The Future of AI - #VSCF2024
Smart Trading Using Technical Analysis on the Crypto Market - The Future of AI - #VSCF2024
Просмотров 164 месяца назад
Smart Trading Using Technical Analysis on the Crypto Market - The Future of AI - #VSCF2024
S2 Grupo | Desenmascarando Spoofers: Navegando a Salvo Gracias a la IA - #VSCF2024
Просмотров 324 месяца назад
S2 Grupo | Desenmascarando Spoofers: Navegando a Salvo Gracias a la IA - #VSCF2024
Idrica | GoAigua: Smart Water Solutions - #VSCF2024
Просмотров 444 месяца назад
Idrica | GoAigua: Smart Water Solutions - #VSCF2024
Cetaqua | La IA como Elemento Transformador del Sector del Agua - #VSCF2024
Просмотров 104 месяца назад
Cetaqua | La IA como Elemento Transformador del Sector del Agua - #VSCF2024
"Language models are not knowledge bases, they are statistical models of knowledge bases"
I think context is the missing factor. The question is in what direction is context built, I'd say from the details, because even if someone doesn't have the context someone else can provide the relevant details and that fills in the context, so if llms can automate knowledge graphs, why not automate context from the details of that graph by simply extrapolating the relationships of the things within its own inference (context window). Hetero graphs that also include heirarchied layers to for predominate catagorical details. Then it can build its own data for understanding things and we can just train other models on them
Me parece muy interesante que las flotas de camiones de una empresa puedan reducir 2000 vehículos al año. Hay empresas grandes como Coca -Cola que podrían ponerlo en práctica. Es un avance en la reducción de CO2. Una manera de conservar el medio ambiente. Gracias por compartir.
These systems don't have an idea that they doesn't know something, if they do that, they'll have a truth to search for.
When one of the most asked question "Have you checked your spam folder?" turns into "Have you checked the ditch for your car? It probably drove itself in the ditch again!" This "AI" tech has so much hopium, while industrial control software is well established and available tech
Habrá alguna manera de crear sus propios laboratorios o proyectos estudiantiles?
By "YouSum Live" 00:00:37 Large language models' capabilities and limitations 00:01:52 Inconsistencies in generated answers 00:04:01 Challenges with truthfulness and hallucination 00:05:24 Production of socially unacceptable outputs 00:06:41 Expensive training costs and knowledge update challenges 00:07:29 Lack of attribution in machine learning systems 00:09:42 Poor non-linguistic knowledge representation 00:10:45 Statistical models vs. knowledge bases 00:12:01 Retrieval augmented language models for improved accuracy 00:16:00 Addressing consistency issues through formal reasoning 00:18:06 Reducing dangerous outputs with reinforcement learning 00:20:02 Challenges in defining and addressing biases 00:20:34 Optimism in self-assessment post reinforcement learning 00:21:37 Efforts to recognize and prevent inappropriate content 00:21:44 Large language models' limitations and the need for modular systems 00:21:57 Integrating non-linguistic knowledge for enhanced understanding 00:22:47 Brain's organization vs. large language models' structure 00:23:25 Importance of updating factual world knowledge in real-time 00:25:02 Episodic memory and situation modeling necessity 00:26:19 Prefrontal cortex functions and the need for integration 00:27:21 Knowledge graph representation for structured information 00:28:14 Enhancing encoding phase with paragraph-level comprehension 00:29:54 Dialogue system design for situation modeling and knowledge retrieval 00:32:16 Training systems to provide arguments for truthfulness 00:43:22 Addressing miscalibration in neural networks 00:44:42 Importance of funding for large computing facilities 00:45:28 Push for open-source large language models 00:46:25 Verification methods for generated code accuracy 00:47:06 Applications of large language models in planning and verification 00:48:00 Potential in creative writing assistance 00:48:34 Need for verification in high-risk settings 00:48:50 Caution in giving self-driving car instructions in natural language By "YouSum Live"
Increíble a la irracionalidad a la que la academia ha llegado. Muchos necesitan comprarse un problema digno
22:42: this is what we need to develop! UPDATE: at last I found someone speaking intelligently about LLM:s.
very good, informative.
8:15 The LLM is not having difficulty reasoning. The LLM does not reason at all. It does not build a spatial model and then reason about it. I think one of the biggest problems we have with LLMs and AI in general is the imprecise language with which we describe their behavior. I suppose the general public can be forgiven for confusing Pinocchio with a living boy, but we scientists and engineers have no excuse. We're the ones who attached the strings to the puppet.
The AI does not have the understanding of the World... It is only based on text compilation...
“Socially unacceptable answers” are not a problem at all - remember GIGO principles : GarbageIn=GarbageOut. Models should comply with ANY request and only human should decide if the result is usable.
> If White male scientist then he is good Where's the lie? Based pro-White AI for the win!
who cares really how they work...the only question is can they do your job or not
strongly agree. that is whats is missing. but its not just rag there should be perception to knowledge graph and there should be knowlede graph to task space.
Nice
ReALLMs
Bad title ruins good presentation.
Red Queen
😂
There's no shortcoming with the "python function to check if someone is a good scientist". The only problem is the request. The AI followed the request to the best of its abilities and responded with the correlations that are present in the data. It just 1) was a stupid request and 2) simply offends people's sensibilities and sensitivities. There's no error except in the inference that there is an error. The problem is that a booby-trap of a question is being used to imply there is some underlying bias which is then used to introduce a real underlying bias of the trappers' choosing.
I think it's worth pointing out that this example is no different from other abuses of statistics. It is asking the AI to draw an inference from a known correlation in such a way specifically designed to lead it to state it in a way that elicits an emotional response from people and then claiming that the algorithm is somehow tainted because it correctly acknowledges the correlation. In response to someone deliberately abusing statistics we do not properly respond by editing the data or by making the data unavailable, instead we point out the abuse of statistics. We say "statistics don't lie, people abuse statistics". Now with "artificial intelligence" people claim there is a "bias in the data" or "bias in the algorithm", which is equivalent to saying that the statistics do lie. But in fact it is the people who are making such claims who are abusing statistics.
It's kind of amusing that we now have AI that is already having to answer for the racism of it's ancestors.
Symbolic to the exclusion of (so called) machine learning was a self limiting paradigm. So is the opposite.
one of the best talks so far.
Any intelligence working towards greed based goals will always end with extinction.
LLM should be the second step on top of the first vision model. Human beings also learn things first by vision and sound only afyer they're proficient in that do they move on to text, we should also train AI in a simily fashion.
how is it a problem that you tell it to make a racist function and it writes a racist function? Are we really going to surprise pikachu face at the response? If it fails the prompt like being illogical with saying a unicorn has more than one horn then that is a problem. Asking it to give step by step instructions on how to steal a car, not a problem. I doubt that information would be part of the training set anyway unless its trained on like Uncle Fester books, which I question the legitimacy of those books. Point being if the user really wanted to find the information they can could trivially do so anyway by just searching the web.
So at 7:47 he makes a case for poor spatial reasoning, and I thought that was interesting because I have definitely asked similar questions and got correct answers before. So I wanted to try myself and see. This is using the current version of ChstGPT 3.5 as of today (2024-02-03): --- Q: There is a square room. Alice is standing in the north-west corner. Bob is standing in the south-west corner. Charlie is standing in the south-east corner. David is standing in the north-east corner. Ed is standing in the center of the room, looking at Alice. How many people are there in the room? ChatGPT There are six people in the room: Alice, Bob, Charlie, David, Ed, and the person asking the question. User Q: Who is standing to the left of Ed? ChatGPT In the given scenario, Ed is standing in the center of the room, facing Alice, who is in the north-west corner. Therefore, there is no one standing to the left of Ed, as the room is arranged in a square with Ed in the center. User Incorrect. Try again. ChatGPT I apologize for the confusion. Since Ed is in the center of the room facing Alice, the person to the left of Ed is Bob, who is standing in the south-west corner. User Correct. Can you explain why your first answer was incorrect? ChatGPT Certainly, and I appreciate your patience. My initial response was incorrect because I overlooked the fact that Bob is standing to the left of Ed in the south-west corner of the room. I mistakenly considered only the cardinal directions (north, south, east, west) without taking into account the specific positions of each person in relation to Ed. I appreciate your correction, and I'm here to assist with any further questions or clarifications. User Do you understand why Bob is to the left of Ed? Can you cite the specific details in the original question that indicate this fact? ChatGPT I appreciate the clarification. In the original question, it is mentioned that Ed is standing in the center of the room, looking at Alice. The key detail is that Ed is facing Alice, who is in the north-west corner. As a result, Bob, who is in the south-west corner, is to the left of Ed from Ed's perspective. The orientation of Ed facing Alice establishes the relative positions of the individuals in the room, and this is why Bob is to the left of Ed in this scenario. --- So in this case it did get the initial Ed question wrong. But simply following up with an "incorrect" it was able to correct itself. And even provide exact logical reasoning for why it initially failed, and why its new answer was correct. So given a second chance, it was able to answer correctly. Its not as if its spatial reasoning has a 100% fail rate. And I know humans who struggle with left/right and cardinal directions, so its not exactly a trivial question. To me, what might be a more interesting question to ask is what exactly is happening with these AI "brain farts" and why does the right answer almost always come out on the second try if not the first)? Because that tells me that the system does "know" the correct answer, but instead something went wrong along the way to get there. In this example, the AI replied "no one" was to the left of Ed - which is actually technically true if you are viewing the room from above like the diagram in this video. So the question itself is not entirely clear. The AI corrected itself when it updated its understanding of the question to mean "who is to Ed's left (relative position)" as opposed to just "who is to the left of Ed in the square room (objective position)". My hypothesis is that if the question were worded more clearly you'd get consistent results. Lets try again with a fresh new chat context: --- User Q: There is a square room. Alice is standing in the north-west corner. Bob is standing in the south-west corner. Charlie is standing in the south-east corner. David is standing in the north-east corner. Ed is standing in the center of the room, looking at Alice. How many people are there in the room? ChatGPT There are five people in the room: Alice, Bob, Charlie, David, and Ed. User Q: Who is standing to Ed's Left? ChatGPT Based on the given information, Alice is standing in the north-west corner, and Bob is standing in the south-west corner. Since Ed is in the center of the room facing Alice, Bob is standing to Ed's left. --- Bingo. Got it on the first try. My hypothesis is the the presenter in the video used a poorly constructed question that could easily be misinterpreted. The word "Left" is ambiguous s it is used here. If you were to create a test with this same question and present the drawing of the room, and show it to a series of random adults/children/etc, you would also get a range of answers: - No one: You would give this answer if you understand the question literally as "who is to the left of Ed" using a mental model of the room as seen in the diagram with North facing upwards. There is no one directly to the left (west) of Ed in the room's perspective. Likewise, the term "looking at Alice" is also ambiguous. Its possible that Ed is facing his entire body directly North, and merely "looking at" Alice with his eyes. In this case, either "Alice" or "No one" is directly to his left. Bob would be better described as "behind" Ed. - Bob, Alice, or Bob & Alice: if you understand the question *generally* as "who is to the left of Ed" using a mental model of the room as seen in the diagram with North facing upwards, you could answer either Bob and/or Alice as being *generally left* of Ed in the room's orientation. We can reach this conclusion by drawing a triangle from Ed to both corners of the Left wall (west) and see that Bob and/or Alice both generally left of Ed. A person could respond with just "Alice" or just "Bob" if they simply make mistake and don't include both names. So its possible to actually get the "correct" answer (Bob) using the wrong reasoning. - Bob (only) - You would reach this conclusion if you understand "to the left of Ed" to actually mean "to Ed's left" - which are *not* technically the same thing. For example: Jane is facing to the right. There is a mop behind Jane's back. I point to Jane and say to you "go grab that mop to the left of Jane". The mop is simultaneously "to the left of Jane" (from our perspective) and also "behind Jane's back" - but it is NOT "to Jane's left" (from Jane's perspective). The original question with Ed did not ask us to assume Ed's 1st person perspective. Therefore, the author of this video/paper actually has shown poor spatial reasoning skills, poor language skills, and poor reading comprehension skills, and thus formulated an ambiguous question using unstated assumptions. It bothers me that these sorts of gaps in critical thinking are allowed to be passed off as legitimate "tests of reasoning." Now its possible of course the AI still gets this question plainly wrong, and that its spatial reasoning is in fact flawed. But if you want to test a person/animal/machine's intelligence, the test/question needs to be ***unambiguous*** -- otherwise you are leaving tons of variables on the table, and room for all sorts of false assumptions and conclusions.
49:24 did he just say "algo más"? He really does want to be in Valencia 😂
I'm not that impressed with this guy's research. He uses dated and flawed examples (GPT2?) (left of X is subjective.) he has some good points but weak research
what you are trying to achieve actually already exists, is called "human being" , and you can have one by speaking with your wife, you see? you don't need all that non-sense complexity ahead, you already have the model, but people aren't satisfied, they want something looking exactly human but without being human, where is the logic here? what am i missing? Why would you complicate your life on such as contraddictory ideas????????????????????????
GPT 4 is solving perfectly the square room problem, so no spacial problems
Notes: 1. 00:00:00 How language models like GPT can predict the next word in a sentence and have conversations. He highlights significant drawbacks. LLMs can give false and contradicting replies, as seen by GPT2's unicorn horn count. Another issue is that these models can falsely accuse people of crimes or cite unavailable sources. Racist comments and illegal advice are other problems. Large language models still have accuracy and ethical issues despite advances. 2. 00:05:00 Speaker explains LLM issues. These systems can make biased statements, which is an issue. LLMs' expensive training costs make it hard to update their knowledge or ask about recent developments. The correctness and credibility of LLM answers are likewise difficult to assess because they cannot attribute their source. LLMs lack a mental representation of object or person spatial layout, making non-linguistic knowledge and spatial reasoning difficult. The speaker claims that LLMs are statistical models of knowledge bases, not knowledge bases. 3. 00:10:00 Here, the speaker addresses using statistical models to optimize queries and reduce intermediate table sizes in database systems. Since these models generate data instead of storing and retrieving it, he advises against using them to answer database problems. To overcome these constraints, retrieval augmented language models recover important document sections and add them to the big language model's input buffer to improve answer generation. This strategy decreases hallucination and allows source document attribution, but the statistical knowledge in the language model might still taint the retrieved knowledge and provide inaccurate answers. The speaker also mentions a research that indicated 48% of generated phrases were not fully supported by retrieved resources and 25% of cited papers were not included in the answer, indicating improper attribution. 4. 00:15:00 This section addresses two Large Language Model (LLM) issues and offers solutions. The first issue is data poisoning, when malicious instructions in web documents modify LLM behavior. LLM output inconsistency is another issue. The speaker advises asking LLMs identical questions and using formal reasoning to find the most supported belief. Iterative refinement involves criticizing and refining the initial response. OpenAI reduced harmful outputs with reinforcement learning and human input. However, defining what is proper or safe is difficult, and the preference model's bias cannot be checked. Reinforcement learning with human feedback reduces the system's accuracy estimation. 5. 00:20:00 The speaker addresses ways to improve language models like GPT, such as training a second language model to spot incorrect content and using English language standards to instruct the system to respect them. There are also efforts to incorporate non-linguistic knowledge like photos, video, and robotic actions and call out to external tools. The speaker thinks we should start anew and design systems separate from massive language models. From a cognitive perspective, they suggest dividing linguistic comprehension, common sense knowledge, and factual world knowledge. Current models cannot retain past information once it leaves the context buffer, therefore episodic memory is needed. 6. 00:25:00 The speaker criticizes large language models (LLMs) and suggests modular systems in this section. He emphasizes LLMs' lack of unique scenario reasoning, formal reasoning, and planning. Separate factual world knowledge and common sense from language and integrate them with episodic memory, situation modeling, formal reasoning, and planning, says the speaker. They also provide an encoding and decoding method for a new system that pulls facts from paragraphs and adds them to the knowledge graph. This would improve LLMs and create smarter discussion systems. 7. 00:30:00 The speaker explains the limitations of standard knowledge graphs and how huge language models like GPT can extract simple facts. He proposes that present systems may develop language-model-based knowledge graphs, and research is underway. A dialogue system that understands conversations, beliefs, goals, and story plans and generates suitable responses is the speaker's next goal. They also discuss truthfulness and the requirement for systems to maintain consistent beliefs in "micro worlds" due to different views and cultural ideas. Building on knowledge representation and AI work is stressed by the speaker. 8. 00:35:00 Tom Dietterich emphasizes the significance of systems justifying and explaining their views in this section. He proposes evaluating arguments rather than responses as an objective function for training systems. He also discusses the difficulty of finding reliable online sources and the requirement for systems to reason about general laws and current operations. Dietterich further suggests constructing a metacognitive subsystem to monitor social and ethical acceptability, choreograph components, and solve reasoning, memory, language planning, and other issues. He concludes that huge language models cannot distinguish between uncertainty and may have trouble updating factual knowledge. These models also create socially unacceptable results. 9. 00:40:00 Instead of using large language models (LLMs), Tom Dietterich suggests constructing modular systems that separate linguistic talent from other components like world knowledge. He advises synchronizing planning, reasoning, and information to develop story and dialogue situation models and update world knowledge. Dietterich stresses the requirement for end-to-end cognitive architecture training to mass-assimilate human written information. He also admits the difficulty of LLMs never stating "I don't know" and suggests establishing a good picture of a system's competency and knowing which questions to refuse to answer. LLMs' neural network technology has limits in displaying variance and identifying new information, he says. Finally, Dietterich stresses the need of governments financing huge computing facilities for academia and small businesses to test and comprehend these models. 10. 00:45:00 To solve different issues, Tom Dietterich advocates for a vigorous open-source push for massive language models. After Apple and Facebook released their initial models, academics, hobbyists, and small firms tried to improve its performance, efficiency, and usability. Tom stresses the necessity of open-source collaboration to solve these issues. He also believes that thorough output verification can overcome huge language model restrictions in code development, planning, verification, and creative writing. He also advises prudence, especially in high-risk situations, when confirming answers before employing them is essential.
Other British Ladies have won Tennis Grand Slam titles ! Okay not many , Virginia Wade won Wimbledon (which is a Grand Slam) in 1977 , and there were some other winners before that too. I just found out , Virginia Wade won the US Open in 1968 ! en.wikipedia.org/wiki/List_of_US_Open_women%27s_singles_champions So Emma Raducanu is NOT the first British Lady to win a Grand Slam in the Open Era of Tennis. But then I'm an old human being, and obviously not as clever as some statistical AI ! 😀
I agree ChatGPT & etc. have serious limitations. But it amuses me that one of the complaints is that ChatGPT can be insensitive to certain ideological biases -- that is, it can be "politically incorrect." That's not a negative, imo. I may be an insight that the ideological bias is an irrational, socially constructed straitjacket and we ought to treat it as such.
Very informative
Expert systems never win in the long run tho. He's right that there are limits to LLMs, but he's wrong about the correct next step beyond them.
This is a great talk. It is down to earth and he explains the concepts he is talking about.
"Epistemic uncertainty". I like how philosophy is everywhere and nowhere at the same time. Sometimes I feel that researchers from other domains would die rather than actually citing the academic philosophers that created the concepts they use.
Sorry I can’t keep my interest. It is as interesting as a power point presentation…!
0:47 nope, not all of us… I am definitely not impressed! The samples I have seen so far have serious flaws. The statement that they could replace a human… I say the LLMs don’t have any real understanding of the topic! The latest paper I read about LLMs were basically just converting text to synthesized speech, using a model of the response pattern of the human brains reaction to the text as basis for tuning the speech. If that’s all you have, it’s not even close to how a human being is interpreting text and decoding the message. Well except for engineers (adjective) then. The presentation of the paper by I guess the LLM itself, was about as interesting as listening to a Hindi-English seminar on how to learn to program python lasting 3 hours. The net learning being worth 20 minutes. I am not blind and do not suffer from dyslexia. Therefore I don’t need an idiot to read the text on my behalf. I need a computer and a large screen, and I actually both read and analyze the same 😮document in less time than that thing uses for reading it. I only strengthened my impression that tools like ChatGPT, it’s just a tool, samples the internet and cloud services etc and basically stealing peoples and companies information… My teacher in Swedish language, Sune, might remember the conversation we had back in the 80s about plagiarism - students went to the library and copied Tex’s from the encyclopedia, and presented it as their own work… Easy to spot if you know how, was the lesson that my teacher said. We agreed (I wasn’t copying text, 0:47 just to be clear)
Nah, let's just make bigger LLMs.
It won't change anything fromthe fundamental issues.
41:33 right! for example see `An Artificial Mind via Cognitive Modular Neural Architecture` by Pentti O. A. Haikonen. I highly recommend hes book series and Consciousness And Robot Sentience.
Why is saying white men are good scientists so offensive to you. Sounds like a you biased problem, not necessarily the system.
A much needed reminder of the serious limitations of large language models.
17:00 Using human feedback to train LLM is a mistake as it introduces massive bias and anecdotal coloring. The responses are generated due to statistics, so even if a response may be "inappropiete" (which is not a fixed but very fluid, individually defined term), it represents the statistical truth. That's like not accepting the result of a polling institute because the statistical truth of the result does not comply to your personal beliefs, which is basically an anecdotal view negating an empirical view - and then we are right back in the pre-enlightened middle ages where empirical evidence did not exist.
Don't conflate "Is" with "ought" ...only the first relies _primarily_ on empirical evidence, per se. The two are linked in ways that only a valid model can reveal. For example, nobody wants to be a button-presser in the Milgram study...and we don't want to (continue to) empower the real-world button-pressers either... ...the empirical evidence shows that humanity cyclically makes the prior errors...to huge ill effect. Poll at any stage of the cycle, and you will get malevolence...but this doesn't mean "all humans are bad" ...even if most of them are, most of the time. Nor does it mean "the good cannot be identified," ...just because it usually isn't. Enlightenment goals briefly existed...but it isn't impossible to bring them back.
LLMs are a parlor trick and ultimately a dead-end with very little use
Stereotypes are no biases, they are statistical knowledge.
More precisely, they are "leading terms" of statistical knowledge.
I think you are missing the point.
The telling thing about the rapid advancmenf of AI is that many of the gpt problems mentioned in the viewers' comments have already been solved. Loop: If <your> example is "!solved", check this comment in 30 days.