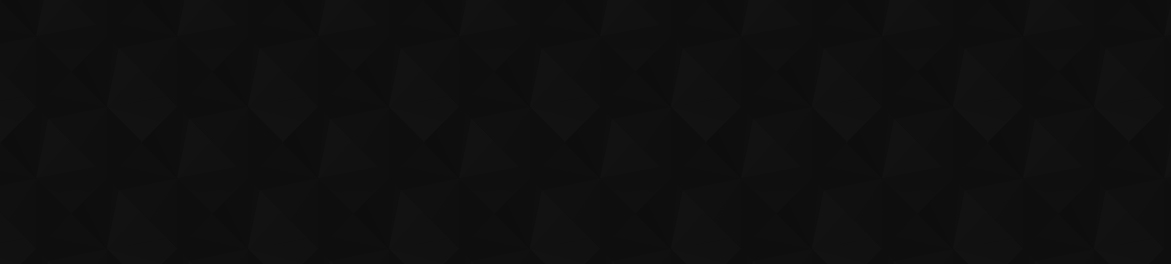
- Видео 256
- Просмотров 73 229
Data Science Salon
США
Добавлен 16 мар 2016
The Data Science Salon has been providing unique content and a diverse, vendor neutral community to data scientists, machine learning engineers and other subject matter experts since 2016.
Our ultimate goal is to eliminate bias, whether it’s in recruitment processes or within the algorithms themselves 🔸🔶
We gather face-to-face and virtually to educate each other, illuminate best practices and innovate new solutions in a casual atmosphere.
DSS has spinoffs like DSS Insider (our on-demand content portal) DSS Elevate (elevating women in data initiative) and other learning programs such as: DSS Webinars, Podcast, 20+ DSS Meetups, DSS Trainings, Newsletter, DSS Slack, Mentorship and Recruitment Initiatives.
See upcoming DSS events here: 👉 www.datascience.salon/upcoming-events/
This channel features selected insights for data science practitioners and managers who want to acquire new skills casually.
Our ultimate goal is to eliminate bias, whether it’s in recruitment processes or within the algorithms themselves 🔸🔶
We gather face-to-face and virtually to educate each other, illuminate best practices and innovate new solutions in a casual atmosphere.
DSS has spinoffs like DSS Insider (our on-demand content portal) DSS Elevate (elevating women in data initiative) and other learning programs such as: DSS Webinars, Podcast, 20+ DSS Meetups, DSS Trainings, Newsletter, DSS Slack, Mentorship and Recruitment Initiatives.
See upcoming DSS events here: 👉 www.datascience.salon/upcoming-events/
This channel features selected insights for data science practitioners and managers who want to acquire new skills casually.
Webinar: Top-10 Misconceptions About LLM Judges in Production
Implementing LLM “judges” in production settings can be a game-changer for evaluating AI behaviors, but it’s often more challenging than it appears. Many teams struggle with common pitfalls such as; high error rates and cost unpredictability to issues with latency and long-term maintenance. This webinar will break down the top 10 misconceptions around LLM judges, equipping you with the insights to avoid these challenges and build more reliable, production-ready evaluation systems.
Join us to learn:
- Key Misconceptions: Understand where teams often go wrong in deploying LLM judges.
- EvalOps Principles: Discover best practices and tools to operationalize evaluations effectively.
- Reliability...
Join us to learn:
- Key Misconceptions: Understand where teams often go wrong in deploying LLM judges.
- EvalOps Principles: Discover best practices and tools to operationalize evaluations effectively.
- Reliability...
Просмотров: 55
Видео
Building Patient Journeys from Multi-Modal Healthcare Data Using Medical Language Models
Просмотров 155Месяц назад
Data integration has been an enormous challenge in healthcare for decades. Issues of data standardization, data quality, legacy formats, unstructured data, and semantic inconsistencies have made it hard to answer basic questions about how a hospital operates or what should be done next for a patient. Recent advances in Healthcare AI combine to transform this age-old problem - enabling you to au...
Fireside Chat - Efficiency Unlocked: The Power of Synthetic Data in Modern Enterprises
Просмотров 582 месяца назад
Synthetic data has emerged as a powerful tool for organizations looking to enhance their operational efficiency and reduce costs. By generating artificial data that mimics real-world data, companies can overcome limitations related to data scarcity, privacy concerns, and high costs associated with data collection and storage. This conversation will delve into the practical benefits and real-wor...
Data Science Salon Miami 2024
Просмотров 282 месяца назад
Data Science Salon Miami is a one-day experience for senior managers, C-suite executives, and top local AI/ML researchers. This exclusive event is all about networking and connecting you with the right people you want to meet. It features a curated mix of use-cases, technical talks, workshops, and Q&A sessions, designed to foster direct interaction and collaboration among industry leaders. Secu...
Data Science Salon NYC 2024
Просмотров 515 месяцев назад
Missed the live action at DSS NYC? You can still catch all the presentations and discussions on demand for the next two weeks. Dive into sessions led by top experts from S&P Global, Nubank, Acorns, Capital One, Citi and many more! Access to the following sessions: 🔸 Navigating #bias in AI: Promoting Ethical Decision-Making in #fintech 🔸 Beyond Financial Services: How to Leverage Financial and A...
Applications of Natural Language Processing for Financial Engineering
Просмотров 818 месяцев назад
Presented by: Moody Hadi, Group Manager - New Product Development & Financial Engineering at S&P Global Natural Language Processing is a contemporary topic in a variety of different fields. In this talk, we will provide a framework for assessing different machine learning applications generally and specifically on sentiment analysis. We will then go over a couple of case studies on NLP apps for...
Framework of Enterprise Adoption of LLMs
Просмотров 1468 месяцев назад
Framework of Enterprise Adoption of LLMs
WELCOME to the Data Science Salon YouTube Channel
Просмотров 3138 месяцев назад
WELCOME to the Data Science Salon RUclips Channel
Operationalizing Large Language Models
Просмотров 33810 месяцев назад
Operationalizing Large Language Models
Navigating the Experimentation Labyrinth: Building Ethical AI Systems
Просмотров 9911 месяцев назад
Navigating the Experimentation Labyrinth: Building Ethical AI Systems
State-of-the-Art Medical Text Mining in 1 Line of Code - David Talby, John Snow Labs
Просмотров 106Год назад
State-of-the-Art Medical Text Mining in 1 Line of Code - David Talby, John Snow Labs
Product Innovation and Enterprise AI in Finance - Winnie Cheng
Просмотров 79Год назад
Product Innovation and Enterprise AI in Finance - Winnie Cheng
Building Efficient Recommender Systems: Techniques, Challenges, and Best Practices
Просмотров 155Год назад
Building Efficient Recommender Systems: Techniques, Challenges, and Best Practices
Fairness in Recommender Systems - Yifei Wang
Просмотров 207Год назад
Fairness in Recommender Systems - Yifei Wang
Applied Machine Learning (a.k.a Research vs. The Real World)
Просмотров 53Год назад
Applied Machine Learning (a.k.a Research vs. The Real World)
Miami Meetup Week: Data Science in Production - Bringing Machine Learning Models to Life
Просмотров 70Год назад
Miami Meetup Week: Data Science in Production - Bringing Machine Learning Models to Life
Creating Personalized & Optimized Marketing Campaigns using Machine Learning - Saumya Srivastava
Просмотров 195Год назад
Creating Personalized & Optimized Marketing Campaigns using Machine Learning - Saumya Srivastava
Machine Learning extending computer vision in Healthcare - Vaibhav Verdhan
Просмотров 82Год назад
Machine Learning extending computer vision in Healthcare - Vaibhav Verdhan
The Impact of Data Experience on Analytics and Machine Learning Solutions - Laura Gabrysiak
Просмотров 122Год назад
The Impact of Data Experience on Analytics and Machine Learning Solutions - Laura Gabrysiak
Zero to Advanced Analytics and Machine Learning in 90 Minutes with TigerGraph Cloud
Просмотров 315Год назад
Zero to Advanced Analytics and Machine Learning in 90 Minutes with TigerGraph Cloud
From big data to no data - two use cases in machine learning
Просмотров 26Год назад
From big data to no data - two use cases in machine learning
Graph Machine Learning: A quantum leap in performance
Просмотров 58Год назад
Graph Machine Learning: A quantum leap in performance
Traditional Software vs Machine Learning Software
Просмотров 130Год назад
Traditional Software vs Machine Learning Software
Application of Machine Learning for Spreading Financial Statements
Просмотров 214Год назад
Application of Machine Learning for Spreading Financial Statements
Machine Learning in Support of Trading and Trading Analytics - Erin Stanton
Просмотров 311Год назад
Machine Learning in Support of Trading and Trading Analytics - Erin Stanton
The Innovative Process of Machine Learning in Finance
Просмотров 53Год назад
The Innovative Process of Machine Learning in Finance
The Art of Observability in Machine Learning: Ensuring Performance and Reliability - Reah Miyara
Просмотров 150Год назад
The Art of Observability in Machine Learning: Ensuring Performance and Reliability - Reah Miyara
Building A Smarter Media Marketplace: ML & AI Demystified for TV Advertising - Yuling Ma
Просмотров 56Год назад
Building A Smarter Media Marketplace: ML & AI Demystified for TV Advertising - Yuling Ma
Excellent job! Congratulations!!!
Glad you enjoyed it!
Chegg is worst!!Their management is worst!!Their AI cant compete with gpt!!Their experts use chat gpt to give answer to students!!(You can confirm the same by looking throught trustpilot reviews). Chegg india support team is sleeping, i work as chegg expert who give solution to students. Colleague experts use chat gpt to answer questions,chegg quality team has given the solution reviewing work to experts only!!So experts are revewing their own solutions. Experts take account from other people and use gpt to reciew even solutioms. Chegg quality team Is doing no work,they are not even checking what sort of useless gpt answers being thrown to the students. We habe raised multiple tickets to stop all this,but support team ,doesnt do anything other thsn sending automated reply. Chegg has 2 way authentication only,they dont use otp for student subscription through credit card. Multiple people do carding fraud and chegg has to refund full amoint,chegg has lost million of amoint owing to this,many more things are there which are being doen via scams
Hey there Anna and Lovedeep... I would love to get guidance from you as to how to start a career in data science, followed by GenAI.
Nice introduction, any more details about tehcnical aspects of that case, instructions, tips on how to implement this kind of solution?
🎯 Key Takeaways for quick navigation: 00:31 *🔄 Gretel AI specializes in creating synthetic data for generative AI programs within enterprises, aiming to aggregate different use cases and implementation strategies.* 02:06 *📊 Synthetic data mirrors real-world data's statistical properties, enabling simulation of real-world situations without needing original data, covering various data modalities like tabular, natural language, and time series.* 03:02 *🚀 Gretel's platform allows for flexible deployment, offering cloud and hybrid models to experiment quickly or deploy at scale within an enterprise's environment.* 03:46 *📈 Use cases include creating synthetic versions of machine learning training datasets to safely share across the enterprise, enhancing data privacy and fidelity.* 05:24 *⏳ Time series synthetic data fills in gaps for expensive-to-collect physical sensor data, supporting simulations and augmenting sparse datasets.* 06:47 *🗣️ Natural language synthetic data supports internal GPT model development, enabling training for bots and detecting toxic language, spam, and fraud.* 07:14 *🖼️ Synthetic images generated through stable diffusion techniques can create costly or hard-to-acquire images for ML training, like in insurance or medtech.* 08:12 *🛠️ Example workflows illustrate how synthetic data can address ML challenges, such as imbalances in training data, by generating additional data samples.* 11:06 *🌐 For enterprises new to ML, synthetic data allows exploration of production databases for ML training set creation, overcoming privacy and access barriers.* 14:21 *📝 Deployment considerations for synthetic data solutions range from open source with various licenses to vendor-hosted or hybrid cloud models, highlighting the importance of understanding license restrictions and deployment needs.* Made with HARPA AI
Incredible conversation! Thanks, folks
Glad you enjoyed it - ty so much for tuning in
@@DataScienceSalon Absolutely, always looking forward to the Salon sessions. Thanks
i liked the way she explained everything - very clear and well-spoken
Marvelous video to learn the big data analysis!!!
great and informative
Excellent! Well presented. Thanks for sharing.
Explained very well. Im a BIG COM SCI GUY
*dark pools and naked shorts have entered the chat* *supply & demand economics has left the chat*
ጸ😊?ጻ❤🎉
🎯 Key Takeaways for quick navigation: 00:02 🎙️ *Introduction and Background* - Erin Stanton's introduction and her role in trading analytics. - Historical evolution of trading analytics from Excel to machine learning. 01:15 📊 *Concepts in Trading Analytics* - Explanation of Andre Pearl's concept of implementation shortfall. - The impact of time delays on trading outcomes. - The influence of supply and demand in economics on stock prices. 04:00 📈 *Challenges in Trading Analytics* - Challenges faced in trading analytics: lack of trust in models and data, data availability, skill set, and access to technical resources. - The importance of starting with a small, well-defined goal in machine learning projects. 07:44 🛠️ *Tackling Challenges in Machine Learning* - Approach to machine learning: starting with small, well-contained problems and involving stakeholders early. - The importance of transparency in model development, including data, features, and algorithms. - The value of building a community and sharing knowledge within the organization. 10:44 🔍 *Feature Selection and Transparency* - A detailed explanation of feature selection and its importance. - Examples of categorical, numerical, and excluded features in a model. - The role of transparency in feature selection and model building. 14:25 🌲 *Random Forest Feature Importance* - The use of Random Forest feature importance in explaining model outcomes. - How feature importance aids in building trust with traders. - The presentation of feature importance to make it understandable to non-ML practitioners. 17:44 🔄 *Simplifying Model Complexity* - Challenges faced in building a complex, all-encompassing model for trading analytics. - The decision to focus on a specific type of trade (algo wheel) and why it simplifies model development. - Explanations of data quality calls and the exclusion of certain data points. 21:53 📊 *Ongoing Model Development* - A continuation of the feature selection process for a more complex model. - The iterative nature of machine learning model development. - Transparency and openness in the model-building process. 23:02 📊 *Model Development and Transparency* - Model development process and transparency with clients. - Peer review process for model evaluation and challenging assumptions. - Exclusion of features based on endogeneity and visual model representation. 25:00 🧩 *Semi-Supervised Learning and Reports* - Use of semi-supervised learning for populating partially tagged data. - Splitting commission rate reports into execution and research components. - Successful outcomes with semi-supervised learning and execution-only reports. 26:29 🤖 *Trading Strategy Recommendation Engine* - Ongoing efforts to develop a versatile trading strategy recommendation engine. - Learnings from previous failures and breakthroughs in the last 18 months. - Expanding machine learning applications to various asset classes beyond stocks. 27:52 🐦 *Twitter Sentiment Analysis Experiment* - Experimentation with Twitter sentiment analysis for stock trading insights. - Challenges encountered in processing visceral language on Twitter. - Classifying this experiment as part of the "failure column." 28:34 🚀 *Confidence in ML Adoption in Trading Analytics* - Anticipation of 2023 as the year for ML adoption in trading analytics. - Building transparent models and addressing data set gaps. - Gaining trust, enhancing skills, and preparing for ML adoption in trading analytics. Made with HARPA AI
nice talk.
Can you share the code, please? tyty
fantastic talk ty!
I really enjoyed this presentation, especially the way that you got very granular with the case studies. Thank you!
Glad you enjoyed the presentation 😀
Thanks for this, I am still a beginner in the field.
what is the type of optimizer used , the audio is not very clear
Is it possible to get the python code and Tableau file to implement the same in my usecase
The Company faces tremendous losses ~97% since last year and is dues to Remote work so this is normal.I am not surprised. But the returns are less than more than 3 y ago. Does this new R&D ML architecture results in ROI increase, RnD in unreliable in measurement for many reasons of course but is there an estimate?
Very good presentation Jayeeta, thanks!
19:34 Amazing potential of transfer learning🙂
Voice quality is bad
So would profit be an input feature of the bayesian optimiser? As in, you multiply the predicted sales by the gross margin and use that as feature to optimise the price.
This video has nothing about Finance except 1 sentence on 1 slide. I suggest the better title would be "The Machine Learning Blueprint". Nothing new or specific about Finance, just common and general practice for any ML project.
Thank you for this nice representation! What are the use cases of ML in finance?
Great material, great perspective, well presented. Thanks
Your video is very beautiful. I like it a lot. I have been trying to talk to you for a long time. I am waiting for your response. Please give me a chance to talk to you..
Nice presentation. I was also hoping to understand 1. Types of visualisations available 2. Which visualisation works in a particular situation and how to arrive there
Very insightful video, thank you
This is great live session
Glad you enjoyed it!
Thanks for sharing this video; it is helpful
Very nice and informative!
Engaging conversation. Useful insights on sustainability with Blockchain technology and NFT use-cases with digital ownership of physical products.
Can you share the github repo for this algorithm please?