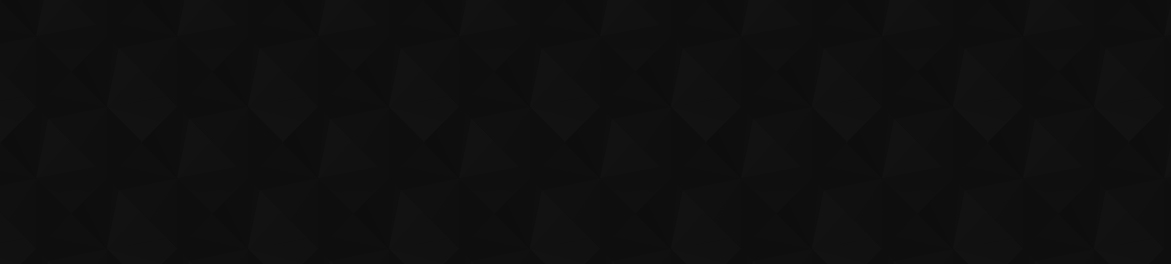
- Видео 32
- Просмотров 111 303
Woody Lewenstein
Добавлен 23 сен 2006
A Level Maths 2022 Edexcel Pure 2 Pure - COMPLETE SOLUTIONS
Complete step-by-step solutions to the 2022 Edexcel A-level Paper 2 (Pure)
I also have a COMPLETE online A-level course with video lessons, quizzes and worksheets covering the entire A-level Maths syllabus for Edexcel, AQA, OCR and MEI.
Get 30% off any of my A-Level maths courses using the code "RUclips30" at checkout. Head over the www.mathematicswithwoody.com to have a look. You can sign up for a free trial on all courses to see if they work for you.
I also have a COMPLETE online A-level course with video lessons, quizzes and worksheets covering the entire A-level Maths syllabus for Edexcel, AQA, OCR and MEI.
Get 30% off any of my A-Level maths courses using the code "RUclips30" at checkout. Head over the www.mathematicswithwoody.com to have a look. You can sign up for a free trial on all courses to see if they work for you.
Просмотров: 358
Видео
A Level Maths 2022 Edexcel Paper 1 Pure - COMPLETE SOLUTIONS
Просмотров 8216 месяцев назад
Complete step-by-step solutions to the 2022 Edexcel A-level Paper 1 (Pure) I also have a COMPLETE online A-level course with video lessons, quizzes and worksheets covering the entire A-level Maths syllabus for Edexcel, AQA, OCR and MEI. Get 30% off any of my A-Level maths courses using the code "RUclips30" at checkout. Head over the www.mathematicswithwoody.com to have a look. You can sign up f...
Introduction to Statistics for Business and Data Analysis
Просмотров 86110 месяцев назад
Introduction to Statistics for Business and Data Analysis
AS Maths 2020 Edexcel Paper 1 Pure - COMPLETE SOLUTIONS
Просмотров 19 тыс.2 года назад
Complete step-by-step solutions to the 2020 Edexcel AS Paper 1 (Pure) If you found this useful and want to support me make more videos like this, you can make a small one-off contribution here: buymeacoffee.com/woodylewenstein Get 50% off any of my A-Level maths courses using the code "RUclips" at checkout. Head over the www.mathematicswithwoody.com to have a look. You can sign up for a free tr...
Introduction to Bayesian Statistics - A Beginner's Guide
Просмотров 77 тыс.2 года назад
Bayesian statistics is used in many different areas, from machine learning, to data analysis, to sports betting and more. It's even been used by bounty hunters to track down shipwrecks full of gold! This beginner's course introduces Bayesian statistics from scratch. It is appropriate both for those just beginning their adventures in Bayesian statistics as well as those with experience who want ...
A-Level Maths: How to Solve a Quadratic Equation with a Calculator
Просмотров 1,2 тыс.2 года назад
How to solve quadratic equations using the calculator. Never mess up a quadratic question again! Save yourself time and effort. If you found this useful, check out the full courses for Pure, Mechanics and Statistics (Edexcel, OCR, AQA, MEI) on my website: mathematicswithwoody.thinkific.com/ Use code RUclipsHALFPRICE at checkout for 50% off all my courses!
A-Level Maths: The Quadratic Formula
Просмотров 922 года назад
How to solve quadratic equations using the quadratic formula. If you found this useful, check out the full courses for Pure, Mechanics and Statistics (Edexcel, OCR, AQA, MEI) on my website: mathematicswithwoody.thinkific.com/ Use code RUclipsHALFPRICE at checkout for 50% off all my courses!
Easy method for solving quadratics by factorising
Просмотров 1102 года назад
How to solve quadratic equations using factorising. Key A-Level maths skill! If you found this useful, check out the full courses for Pure, Mechanics and Statistics (Edexcel, OCR, AQA, MEI) on my website: mathematicswithwoody.thinkific.com/ Use code RUclipsHALFPRICE at checkout for 50% off all my courses!
Modelling Assumptions: A-Level Maths Mechanics Y12
Просмотров 3,7 тыс.4 года назад
In this video we look at all the modelling assumptions needed for A-Level Mechanics. For example, modelling objects as particles, or treating strings as light and inextensible. This is needed in A-Level Maths Mechanics, and is suitable for all major exam boards, such as Edexcel, AQA, OCR and MEI. I hope you found this useful! The full course is available on my website, where you can find full c...
Connected Particles - maximum height of an object over a pulley: A-Level Maths Mechanics Y12
Просмотров 7594 года назад
In this video we look at connected particles pulley questions, and focus on finding the maximum height reached by an object after the object at the other end of a string hits the ground. This is needed in A-Level Maths Mechanics, and is suitable for all major exam boards, such as Edexcel, AQA, OCR and MEI. If you found this useful, check out the full courses for Pure, Mechanics and Statistics (...
Connected Particles - particle strikes the ground: A-Level Maths Mechanics Y12
Просмотров 2334 года назад
In this video we look at connected particles, and focus on questions where one of the objects strikes the ground, making the string go slack. This is needed in A-Level Maths Mechanics, and is suitable for all major exam boards, such as Edexcel, AQA, OCR and MEI. I hope you found this useful! The full course is available on Udemy. Use this link to get 30% off: www.udemy.com/course/a-level-mechan...
Connected Particles - Pulleys: A-Level Maths Mechanics Y12 (part 2)
Просмотров 2594 года назад
In this video we look at connected particles, and go through a harder example of a pulleys question. This is needed in A-Level Maths Mechanics, and is suitable for all major exam boards, such as Edexcel, AQA, OCR and MEI. I hope you found this useful! The full course is available on Udemy. Use this link to get 30% off: www.udemy.com/course/a-level-mechanics-1/?couponCode=FOUNDONRUclips
Connected Particles - Pulleys: A-Level Maths Mechanics Y12 (part 1)
Просмотров 3104 года назад
In this video we look at connected particles, focusing on pulley questions. This involves using Newton's Second Law (F=ma) and SUVAT equations. This is needed in A-Level Maths Mechanics, and is suitable for all major exam boards, such as Edexcel, AQA, OCR and MEI. I hope you found this useful! The full course is available on Udemy. Use this link to get 30% off: www.udemy.com/course/a-level-mech...
Connected Particles - Car Towing Trailer: A-Level Maths Mechanics Y12
Просмотров 1,7 тыс.4 года назад
In this video we look at connected particles, focusing on situations where there is a car towing a trailer. This uses Newton's Second Law (F=ma) as well as the SUVAT equations. This is needed in A-Level Maths Mechanics, and is suitable for all major exam boards, such as Edexcel, AQA, OCR and MEI. I hope you found this useful! The full course is available on Udemy. Use this link to get 30% off: ...
Clearest explanation of Newton's Second Law and SUVAT: A-level Maths Mechanics Y12
Просмотров 1314 года назад
In this video I show you how to use Newton's second law (F=ma) in combination with kinematics (SUVAT equations). This is needed in A-Level Maths Mechanics, and is suitable for all major exam boards, such as Edexcel, AQA, OCR and MEI. I hope you found this useful! The full course is available at mathematicswithwoody.thinkific.com. Use code RUclipsHALFPRICE to get 50% off all courses!
Newton's Second Law of Motion (F=ma): A-level Maths Mechanics Y12
Просмотров 1064 года назад
Newton's Second Law of Motion (F=ma): A-level Maths Mechanics Y12
Newton's Second Law of Motion (F=ma): A-Level Maths Mechanics Y12 (part 2)
Просмотров 684 года назад
Newton's Second Law of Motion (F=ma): A-Level Maths Mechanics Y12 (part 2)
Newton's Second Law of Motion (F=ma): A-Level Maths Mechanics Y12 (part 1)
Просмотров 834 года назад
Newton's Second Law of Motion (F=ma): A-Level Maths Mechanics Y12 (part 1)
Parallel Vectors: A-Level Maths Mechanics Y12
Просмотров 6694 года назад
Parallel Vectors: A-Level Maths Mechanics Y12
Force as Vectors: A-Level Maths Mechanics Y12
Просмотров 754 года назад
Force as Vectors: A-Level Maths Mechanics Y12
Force as Vectors: A-Level Maths Mechanics Y12 (part 1)
Просмотров 1564 года назад
Force as Vectors: A-Level Maths Mechanics Y12 (part 1)
Magnitude and Direction of a Force Vector: A-Level Maths Mechanics Y12
Просмотров 7254 года назад
Magnitude and Direction of a Force Vector: A-Level Maths Mechanics Y12
Resultant Forces: A-Level Maths Mechanics Y12
Просмотров 2374 года назад
Resultant Forces: A-Level Maths Mechanics Y12
Force Diagrams: A-Level Maths Mechanics Y12 (part 2)
Просмотров 1984 года назад
Force Diagrams: A-Level Maths Mechanics Y12 (part 2)
Force Diagrams: A-level Maths Mechanics (part 1)
Просмотров 8024 года назад
Force Diagrams: A-level Maths Mechanics (part 1)
Modelling with straight lines in GCSE and A-Level maths (part 1)
Просмотров 5414 года назад
Modelling with straight lines in GCSE and A-Level maths (part 1)
Modelling with straight lines in GCSE and A-Level maths (part 2)
Просмотров 1594 года назад
Modelling with straight lines in GCSE and A-Level maths (part 2)
Areas between straight lines in GCSE and A-Level maths
Просмотров 874 года назад
Areas between straight lines in GCSE and A-Level maths
How to find the distance between two points
Просмотров 404 года назад
How to find the distance between two points
How to find the equation of a perpendicular bisector
Просмотров 2394 года назад
How to find the equation of a perpendicular bisector
Thank you for this absolute gem of a lecture. I think it might tickle you to know how I approached the problem re Tanya's tennis and sunny days (23:00 ish). As a healthcare professional, I'm much more familiar with sensitivity, specificity, positive/negative predictive values, prevalence etc, than I am with an equation for conditional probability. So for my first attempt at the problem, I basically framed the situation as "tennis" being a diagnostic test for "sunny", and drew a 2x2 table for "tennis" against "sunny"! The sensitivity of the test is 80%, ie. 80% of "sunnies" had a positive "tennis" result. The specificity of the test is 65%, ie. 65% of "not sunnies" had a negative "tennis" result. The "prevalence" of sunny is 60% and not sunny is 40%. Therefore, I just had to solve for the positive predictive value of the "tennis" test for "sunny", by using the relative prevalence to "weight" the sunnies vs not-sunnies within the "tennis" group. Et voila, it yielded the exact same process of multiplication as using the cond probability equation, which I used for my second attempt. I know this may sound like a much more complicated method, but seeing the probabilities in a table and applying concepts I already know truly helped me actually understand the multiplications within the conditional probability equation, rather than just solving for it blindly. This was a lightbulb moment for me. Thank you!
@@jayashrishobna really interesting! So nice to hear about how you went about solving this!
thanks dawg
This was an awesome video; really appreciate it!
This video deserves more likes.
I just love the flow of the lecture.
Thank you so much! Lovely to hear that!
thank you
Really appreciate your explanation of Q13 - I wondered how the mark scheme started with (2a-b)^2≥0
Excellent. In general I leave comments 0.5% of the time. But when I think something is really superb I always leave a comment. What is the chance I thought Woody’s tutorial was really superb?
Thanks! Glad my video made the cut!
Timestamps would help greatly. Great course anyways ❤
Question: at 34:18 The question is if a child take the pill then how likely they will be a genius. Why at 35:25, it is that being a genius is a given? shouldn't it be P(genius | pill)?
🎯 Key Takeaways for quick navigation: 00:00 *🎓 Introduction to Bayesian Statistics* - Exploring Bayesian statistics from scratch. - Suitable for anyone interested in probability and statistics, from students to professionals. - Starting with fundamental questions about probability and its applications. 01:10 *🎲 Objective vs. Subjective Views on Probability* - Contrasting objective (frequentist) and subjective (Bayesian) views on probability. - Highlighting limitations of frequentist approach, especially for one-off events like horse races. - Illustrating subjective Bayesian model's flexibility and rationality in handling uncertainty. 09:33 *📊 Degrees of Belief in Bayesian Probability* - Bayesian probability as degrees of belief or uncertainty measures. - Illustrating subjective probabilities through scenarios involving pregnancy and gender prediction. - Emphasizing rationality in adjusting beliefs based on available evidence. 10:01 *🧠 Conditional Probability Basics* - Introduction to conditional probability using simple visual examples. - Building intuition for conditional probability through visualizations. - Setting the stage for understanding Bayes' theorem. 13:19 *📝 Formulating Baby Bayes Theorem* - Deriving a simplified version of Bayes' theorem using visual probability representations. - Demonstrating application of the theorem in simple probability problems. - Introducing notation and terminology for hypothesis and evidence probabilities. 20:20 *🌳 Bayes Theorem Application with Tree Diagrams* - Applying Bayes' theorem to complex scenarios using tree diagrams. - Solving probability problems involving multiple events and conditional probabilities. - Demonstrating how evidence updates prior probabilities to yield posterior probabilities. 23:34 *📈 Bayesian statistics application example: Updating probability with evidence* - Bayes' theorem updates the probability of an event given new evidence. - Example: Given the probability of sunny weather and playing tennis, Bayes' theorem helps update the probability of sunny weather given that tennis was played. - Demonstrates how prior beliefs are adjusted based on new information. 24:45 *📊 Bayesian statistics application example: Probabilistic analysis in economics* - Scenario: Analyzing the probability of a recession given job loss using Bayes' theorem. - Demonstrates the use of prior probabilities and conditional probabilities in economic analysis. - Shows how Bayesian statistics can be applied to decision-making in economic forecasting. 29:22 *🏃♀️ Bayesian statistics application example: Probability distributions in sports* - Example: Analyzing the probability of a girl running 100 meters in a certain time frame using normal distribution. - Shows how Bayesian statistics is used to update probabilities based on additional information (e.g., being in the school running team). - Illustrates how conditional probability influences the assessment of outcomes in sports. 33:19 *🧠 Bayesian statistics application example: Counter-intuitive results* - Examines counter-intuitive outcomes using conditional probability in IQ distribution scenarios. - Demonstrates how small changes in distributions can lead to significant shifts in probabilities. - Highlights the importance of understanding conditional probability in interpreting statistical results. 41:42 *🦠 Bayesian statistics application example: Medical diagnosis* - Examines a medical diagnosis scenario using Bayes' theorem. - Illustrates how prior beliefs are updated based on diagnostic test results. - Emphasizes the significance of understanding conditional probability in medical decision-making. 48:11 *📊 Understanding Bayes Theorem through an Example* - Explains the application of Bayes Theorem using an example involving Steve, a shy individual, to illustrate how prior probabilities and evidence combine. - Demonstrates how intuition can be misleading when prior probabilities and evidence are not considered. - Breaks down the calculation process step by step, showing the application of Bayes Theorem in determining the probability of Steve being a librarian given certain traits. 51:42 *📈 Formal Naming and Components of Bayes Theorem* - Defines the formal components of Bayes Theorem: prior, posterior, likelihood, and evidence. - Illustrates the terminology used in relation to each component, such as "prior" for the initial probability, "posterior" for the updated probability, "likelihood" for the probability of evidence given a hypothesis, and "evidence" for the total probability of the observed evidence. - Provides insights into the significance of each component in Bayesian inference and decision-making processes. 56:36 *🔍 Exploring a Complex Example: Bayesian Approach vs. Frequentist Approach* - Introduces a more complex example involving a game between Alice and Bob to compare Bayesian and frequentist approaches. - Contrasts the frequentist method, which relies on straightforward calculations, with the Bayesian method, which involves applying Bayes Theorem to update probabilities based on evidence. - Demonstrates how Bayesian inference can provide more accurate predictions by considering prior probabilities and updating them with observed evidence, even in complex scenarios. 48:40 *📊 Bayesian Intuition: Steve's Occupation* - Daniel Kahneman presents a scenario about Steve, a shy and tidy individual, posing the question of whether he's more likely to be a farmer or a librarian. - Despite intuitive judgment favoring Steve being a librarian, Bayesian analysis challenges this assumption by considering the proportion of farmers and librarians meeting Steve's criteria. - Applying Bayesian theorem, the analysis shows that Steve is more likely to be a farmer, emphasizing the importance of considering the base rate in making probability assessments. 51:28 *📈 Bayesian Terminology: Understanding Bayes Theorem Components* - Prior: The probability of a hypothesis before considering any new evidence. - Posterior: The probability of a hypothesis after considering new evidence. - Likelihood: The probability of observing the evidence given that the hypothesis is true. - Evidence (Marginal Likelihood): The probability of observing the evidence, accounting for both scenarios where the hypothesis is true and where it's not. 56:36 *🎲 Bayesian Approach: Alice and Bob's Game* - Illustration of a game between Alice and Bob where a ball is randomly placed on a table, dividing it into two sections, with each player scoring points based on where the ball lands. - Contrasting frequentist and Bayesian approaches in assessing Bob's probability of winning the game. - Through simulation, Bayesian analysis consistently yields a higher probability of Bob winning compared to the frequentist approach, demonstrating the Bayesian method's reliability in probabilistic assessments. Made with HARPA AI
Thanks
41:28 similar to IQ distributions for men and woman
@ 38 minutes you say "1 in a Million" and represent it as 0.00000011. "That's 1.1 in 10 million" You are getting this from a normal distribution and I think you have misinterpreted the statistic, rather than used the wrong figure. 0.1 is "1 in 10", 0.01 "1 in 100", 0.001 "1 in 1,000" etc
Good spot that there's an issue here. I'd never noticed! I just re-did the calculation, and in fact I said the correct thing but wrote down the incorrect number. The actual probabilities are 1.1 x 10^-6 and 2.1 x 10^-6. Roughly 1 in a million and 2 in a million. But I seem to have put extra zeros in my decimals. How annoying! Thanks for letting me know though!
Thank You. So helpful!
Thanks! Glad you found it helpful!
Do you also have videos on binomial probability? Or perhaps you know of a good introductory course or book?
But aren't you assuming that P(Bob wins) is 100%? So you start with a very strong prior. Or where I am wrong? ;-)
I don't think I ever assume this, but can you let me know at which point you think I might have?
I guess what u are referring to is the part where he derive the equation for P(a = 5 \land b = 3 | bob win)*P(bob win) on 1:07:49? This probability simplify to P((a = 5 \land b = 3) \land bob win), which should be equivalent to P(a = 5 \land b =6): this is the only state under which bob could win.
Thank you for this video. What I missed is how you calculated the probability in your first example for normal distribution. You just said look at the area under the curve and you said calculate and get the value.
Calculating probabilities with the normal distribution essentially involves using a computer or a calculator to find the area. While there is a (complicated) function for the normal distribution, there is no formula for computing the area under the curve. you have to just use a computer/calculator to find it. Before computers, it just had to be approximated by hand!
Excellent presentation. Wouldn't the Monty Hall Problem be an example of where using Bayes would be helpful? The update info would be that the host, Monty Hall will switch to a door with a goat. The setup is this: Monty Hall is the host of a TV Show, where a contestant must choose one of three doors , where there are goats behind two of the doors, and a car behind the other door. If the contestant chooses the door with the car, they get to keep it. If they choose a door with a goat behind it, they're out. The additional info is that once the contestant selects one of the doors, Monty will stop the show, open up one of the doors containing a goat, and proceed to ask the contestant if they'd prefer to switch to another door. Then the question is whether it is a good idea for the contestant to switch. Answer is yes, given by choosing at random, uniformly, the contestant will have initially chosen the car only 1/3 of the time, and one of the two goats 2/3 of the time. So, 2/3 of the time, contestant will have made the wrong choice, and will improve the odds by switching. I hope this isn't too confusing.
Exactly! Yes, the Monty Hall problem is a very good example of Bayes' theorem in practice!
@@woodyrowHi again, if I may ask a more general question in Statistics: Once a hypthesis test has been setup: Difference of Means, Proportion, Anova, etc. Is there a "Natural" way of defining the statistic to be used to determine whether we reject or don't reject the Null Hypothesis?
Great question@@fernandojackson7207 . I suppose overall I'd say there is not a general natural way of doing this. In practice, you learn when and where to use each one through practice. I think the best I could do is maybe to suggest thinking through the following: 1. Type of Data: The nature of the data (e.g., categorical vs. continuous, paired vs. independent samples) significantly influences the choice of the test statistic. For example, a t-test is appropriate for comparing the means of two independent samples of continuous data, whereas a chi-square test is used for categorical data. 2. Hypothesis Being Tested: The hypothesis itself (difference of means, proportion, variance, etc.) guides the choice of the statistic. For instance: For testing differences between means, you might use a t-statistic in a t-test. For proportions, a z-statistic might be used in a z-test. For comparing variances, an F-statistic is used in ANOVA (Analysis of Variance). 3. Assumptions Underlying the Statistical Test: Each statistical test comes with its own set of assumptions (e.g., normality, homogeneity of variances, independence). The choice of statistic is contingent upon whether these assumptions are met. For example: A t-test assumes normally distributed differences, but if this assumption is violated, a non-parametric test like the Mann-Whitney U test might be more appropriate. ANOVA assumes homogeneity of variances among groups; if this is not met, you might use a Welch's ANOVA instead. 4. Design of the Study: The study design (e.g., matched pairs, blocked designs) also influences the choice. For matched pairs, a paired t-test uses the differences within each pair as the data for analysis.
But beyond these sorts of observations, it's often just the case that through experience you begin to recognise what is appropriate in which situations. Hope that helps a little!
@@woodyrow Thank you so much for the explanation, Woody.
Thank you!
My pleasure!
Thanks for the interesting and clear video! I have a doubt about Amira and Jane problem: why do you assume that Jane being late has an impact on Amira being late? From what we know, one could come from the Moon and the other from Mars. Did I miss something?
Good question. I don't assume that Jane being late had an impact on Amira (in the sense that one caused the other), but we have proved that the events are not independent. This means there is some relationship between one being late and the other being late. If they were truly independent of one another, we would see the property that P(A and J)=P(A)P(J). Since we don't see this, they are not independent. So this could be that Jane being late caused Amira to be late, it could be that Amira being late caused Jane to be late, or it could be some other thing caused both to be late. Eg one is coming from the moon and one is coming from Mars, and a meteor shower caused both rockets to do an extra loop before landing!
Great !!!
Great session sir! Just one que: Why did you take rand() < a particular column.. why not >?
Hi. Sorry for the slow reply! It's just a handy way to get a random variable based on a specific probability. If I say rand()<0.2, then this will occur 0.2 of the time. Remember, the rand() function creates a number between 0 and 1. If I'd done rand()>0.2, this will happen 0.8 of the time. Hope that clears it up!
Excellent video! I needed to refresh my Bayesian statistics knowledge and this was a perfect start.
Glad it helped!
Indeed your video is FANTASTIC and IMMENSILY helpful. Thanks!!!
Thanks! Glad you liked it!
Woah! Quality stuff and your examples added more to grasp the intuition underlying these Bayesian concepts. Regards from Pakistan <3
Thanks so much!
Correction @26:49 'we therefore know that there's a 95% chance that he will NOT lose his job ' instead of 'we therefore know that there's a 95% chance that he will lose his job '
Good spot! 4 years and I never noticed this!
Great lesson! Thank you. Keep up the good work.
I'm not sure if I agree with your solution at 20:13. This works assuming conditional probability (or Jane being late is influenced by Amira). But if these were independent events then the answer would just be 20%. If you know nothing about Jane and Amira, is it rational to assume it's conditional or independent? Lastly does causal reasoning play a part here? Thanks :)
You are absolutely right that if the events were independent then the probability of Amira being late would just be 20% still. However, the information given in the question actually proves they are not independent. If we knew nothing about them then it's hard to say if it's rational to assume independence. But we DO know something about them: thr information given in the question. So this shows their lateness is not independent of each other. But this does not indicate causality. It might be that Jane's lateness causes Amira's, or the other way around. Or maybe something else causes both (eg maybe they catch the same bus and when this arrives late they are often both late).
@@woodyrow Thanks for your reply! I only just discovered your channel and I really like it. I've been going through examples slowly to build intuition. What if Janes lateness was always caused by bus delays, and Amiras lateness was always caused by bad weather. In this case, they would both be independent because there's no way Janes lateness could depend on Amiras lateness and visa versa. Why does the information in question prove that they're dependant on each other? Is it because of the "70% neither of them is late" fact?
@@matthewjames7513 Sorry for the slow reply! If Jane's lateness was always caused by the bus, and Amira's was caused by bad weather, and these didn't interact, then we might expect the events to be independent. However, the probabilities absolutely prove they are not! Remember, the formula P(A&B) = P(A)P(B) is the necessary and sufficient condition for events to be independent. Look at the conditional calculation for P(Amira late given Jane is late). In general, Amira is late 20% of the time. But when Jane is late, Amira is late 60% of the time! Here's what this means: If I just asked you "what is the probability that Amira is late?", you should say "20%". However, if I also tell you that Jane is late, you should say "60%". That is, if you learn that Jane is late, you change your view about the likelihood of Amira being late. This is what it means for events to be not independent. If one happens, you adjust your view on the other. These figures (20% vs 60%) emerge mathematically from the Venn diagram. And they tell us the events aren't independent. I hope that all makes sense! I'm really glad you enjoyed this video by the way. Thanks for the questions!
“Ignore biology and assume everyone is male or female”? Huh?
Better guide to Bayes ruclips.net/video/cqTwHnNbc8g/видео.htmlsi=6s0zOwifUtggbolo
A small % of people are intersex. I have no idea what this % is, but just acknowledging that it's not exactly 50% male, 50% female. Pretending it is makes the probability mucb easier!
👏
Brilliant course, thank you for your efforts & the material!
Thanks so much! So glad you liked it!
I knew I recognised your name! I do believe I taught you maths and/or further maths a million or so years ago? You're teaching now?!
Ronnie! Yes you did, and I absolutely loved your lessons! I've been teaching maths for 10 years now. And you?
This might be the greatest comment of all time
This is amazing😭
Hi Woody, Thanks for this lesson! It is very useful. It's quite challenging to get rid of the frequentist mind after spending my entire life as so, though. I just a have a question: in the simulation, why did you calculated the last probability by using the number of rounds won by either Alice or Bob and not by the number of rounds (10000)? That's how simulations usually work. So I'll be more than grateful if you could help me out here with this doubt.
Hi Jessica. We are trying to work out the probability of someone winning from a position where they are losing (by 5 points to 3). So we simulate and find all the situations where someone was losing by this score, and then see (out of these!) how many times they go on to win. If we divided by 10000 we would be working out the probability that someone plays the game, falls 5-3 behind and then goes on to win, which is a different question. Our question was: IF someone is already 5-3 behind, what is the probability that they win. To use the lingo, we want to probability they win "given" that they are 5-3 behind. Hope that makes sense!
great explanation, i like how you gradually introduced the different concepts from probability -> conditional probability -> baby bayes -> bayes
This is the best explanation I ever saw.
The first lesson is everything! I finally understand the fundamental difference between Bayesian and frequentist statistics. 🎉 beautifully explained, thank you!
Thank you so much!
As I wrote before this is the best ever explanation of the Bayesian statistics - THANK YOU VERY MUCH!!! and I am coming back to it every time to refresh this concept and vocabualry :)! When we talk about the numerator ( P(a=5, b=3 | Bob wins)*P(Bob wins) ) of the probability equation (that Bob wins given A=5 and B=3) at 1:07:20. Basically we can not really operate with these terms separately (P(a=5, b=3 | Bob wins) * 0.057) and have to merge them together into the distribution (y=(8x3)x^6(1-x)^5). Just trying to catch a moment and a point when it pivots from the frequentist to the bayesian :). In other words the point is that when we look at this formula as a math expression then we can camcel out the y=(8x3)x^3(1-x)^5 while left only with (3/8)^3 which then would be purely frequentist estimation of the probability of Bob winning (I do understand that in fact we have the areas under the graphs for the respective distributions in numerator and denominator). If you were so patient to having read till this point :) - what is confusing is the P(a=5, b=3 | Bob wins) part of the numerator which is hard to imagine...
really enjoyed
Thanks so much! Really glad you enjoyed it!
excellent lecture
The best tutorial on youtube that explains Bayesian Statistics so far! 🌷
Wonderful teaching, earlier i found it difficult to understand the probability but now its seems easy. thanks prof.
For question 11 could you have used 6 then squared it to 36. If you go up by 6 you're also going out of the 4th quadrant, why would it only be horizontal and not vertical
It’s because the smallest distance it could go out the 4th quadrant is 4. If you did 6, it would definitely go out of the quadrant horizontally as its more than 4 so it’s more convenient to use 4 and do it horizontally. Hope that makes sense.
Thank u sooo much. I really understood everything
Thank you! So glad you found it useful!
Great video, thank you. I feel like the pill sample calculation is not correct. If there is a very tiny change in mean like 100 -> 100.0000000001, the calc made(from the video) will yield almost 50% that the genius has taken the pill which seems incorrect. I feel like it should be [P(102)-P(100)]/P(100) - I may be wrong but the original calc seems wrong to me. Also the number of zeros for calculated probability should be 5 instead of 6 zeros. Thanks
So clear, excelllent video! Was very helpful 👏🏼👏🏼 Thank you!
Thanks so much! Really glad you enjoyed it!
How can I simulate the billiards example in R?
I did it myself. Actually I was doing it correctly. I was getting the distribution of the bernoulli probability of x as 0.4 which is correct based on the solution given in BDA ie (k+1)/(n+2) which is (3+1)/8+2 = 0.4. Also the MAP estimate was 3/8. My mistake was that I was taking the point estimate and cubing it. In reality I had to play 3 bernoulli trials with the obtained value of theta and see which one is actually winning using the uniform rng like you did in excel. After doing that, I can see the mean of quantity generated to be 0.09 and SD of 0.29
Best explanation! Finally I get it😂
Thanks so much! So pleased to hear this.
I have been interested in Bayesian analysis for a few years and seen dozens of videos. This is the best video I have seen to learn the concepts. Thank you so much for producing and sharing this knowledge!
I'm thrilled to hear this! So glad you enjoyed it. Woody
@@woodyrow Most europeans have 100IQ, East asians 105, ashkenazis 112, african americans 85, sub saharan africans 70.
Wow, thank you.