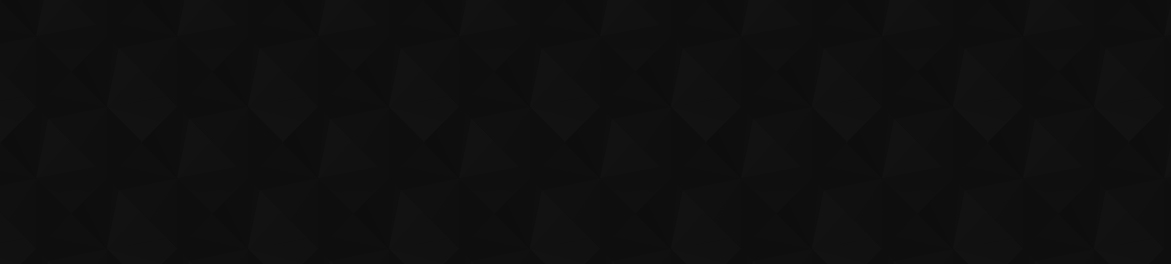
- Видео 13
- Просмотров 35 954
Greg Kahn
Добавлен 28 сен 2011
LaND: Learning to Navigate from Disengagements
Gregory Kahn, Pieter Abbeel, Sergey Levine
Berkeley AI Research (BAIR), University of California, Berkeley
Paper: coming soon
Code: github.com/gkahn13/LaND
Website: sites.google.com/view/sidewalk-learning
Berkeley AI Research (BAIR), University of California, Berkeley
Paper: coming soon
Code: github.com/gkahn13/LaND
Website: sites.google.com/view/sidewalk-learning
Просмотров: 2 012
Видео
Dissertation Talk: Mobile Robot Learning
Просмотров 7343 года назад
Dissertation Talk: Mobile Robot Learning
Model-Based Meta-Reinforcement Learning for Flight with Suspended Payloads
Просмотров 2,8 тыс.4 года назад
Suneel Belkhale, Rachel Li, Gregory Kahn, Rowan McAllister, Roberto Calandra, Sergey Levine Berkeley AI Research (BAIR), Facebook AI Research (FAIR) Paper: arxiv.org/abs/2004.11345 Website: sites.google.com/view/meta-rl-for-flight
BADGR: An Autonomous Self-Supervised Learning-Based Navigation System
Просмотров 2,4 тыс.4 года назад
Gregory Kahn, Pieter Abbeel, Sergey Levine Berkeley AI Research (BAIR), University of California, Berkeley Paper: arxiv.org/pdf/2002.05700.pdf Code: github.com/gkahn13/badgr Website: sites.google.com/view/badgr
Generalization through Simulation
Просмотров 2,5 тыс.5 лет назад
Generalization through Simulation: Integrating Simulated and Real Data into Deep Reinforcement Learning for Vision-Based Autonomous Flight Katie Kang*, Suneel Belkhale*, Gregory Kahn*, Pieter Abbeel, Sergey Levine Berkeley AI Research (BAIR), University of California, Berkeley arxiv.org/abs/1902.03701 github.com/gkahn13/GtS
Composable Action-Conditioned Predictors: Flexible Off-Policy Learning for Robot Navigation
Просмотров 1,3 тыс.5 лет назад
Paper: arxiv.org/abs/1810.07167 Gregory Kahn, Adam Villaflor, Pieter Abbeel, Sergey Levine Berkeley AI Research (BAIR), UC Berkeley In proceedings of the Conference on Robot Learning 2018 Code: github.com/gkahn13/CAPs
Self-supervised Deep Reinforcement Learning with Generalized Computation Graphs for Robot Navigation
Просмотров 24 тыс.7 лет назад
Self-supervised Deep Reinforcement Learning with Generalized Computation Graphs for Robot Navigation
WOW. This is amazing. Thank you for sharing your work. Where can I find journal version of this paper?
Great work !!!
Excellent Work!!
Im curious how the Tello drone is able to stabilize/hover with that payload, a tiny swing of the payload would obstruct the visual odometer at the belly of the aircraft and might cause a runaway perturbation of the hover
@greg kahn i will try to implement this project, any sort of help will be appreciated
Congratulations!
I'm looking similar project this one, do you have github code for this?do you employ opencv?
What kind of MPC did you use? Also, how did you set up the hyperparameter of MPC such as horizon and action candidates? I could not find any reference about details of MPC on the paper. I think PETs needs huge computational effort, and it made me wonder how you implemented PETs online...
what about the code bro?
Jesus Christ I thought that I would never have found this topic and suddenly here it is. Great job and I hope that you will create much more similar content. Keep it up!
your picture in picture doesn't seem to match up. the follow cam shows the drone do one thing, drone does a different thing.
Great talk, I really liked the last part. Also thanks for having a such a good homepage, it is pretty helpful. Congratulations for your phd!
Nice
Wow how many ubereats doordash we can delivery now
In the paper, you claim that your approach learns from scratch to navigate using monocular images solely in the "real-world". So, did you train in a simulation and then evaluate the trained model in the real world or train it directly in the real world (it may be a real collision happen to the car to get more experience) ?
I can see why I’m the comment number 3
what about use many linear neural net to works as seprarte networks but are targeted the same travel path by let each network read the same input data but compete for their output by forwarding into a output network that will select the optimum path ahed of time before the actual try? would not this speed up the overall learning performace?
Which simulator using to testing?
CARLA github.com/carla-simulator/carla
so uc berkley isnt all bad😂 jk but this is siiick
this is bloody awesome!
Fake! You can clearly hear the mouse you've trained to push it along! Nice work :)
dont know if you are sarcastic. Im guessing a mouse wouldn't push this fast.
I enjoyed this.
Can it simulate in gym-gazebo?
The simulator in this release uses Bullet (for physics simulation) and Panda3d for graphics rendering. However, adding an interface to a new environment should hopefully be straightforward.
good job
Do you have a paper published ? Thanks!
arxiv.org/abs/1709.10489
Hi, It was a great stuff. Anyway, a question about how you trained the agent. Is there any terminal state? For example when the agent hit the wall or flipped and how about the reward? Thank you
Good question. Yes, any type of collision is a terminal state. After a collision, the car performs a hard-coded backup procedure and then continues learning. The hard-coded backup is not necessarily needed, and we are going to remove it soon. When evaluating the (N-step) Q-learning prior methods, the reward was the speed of the car, or 0 if a collision occurred. We tried adding a negative reward for collisions, but this actually hurt the performance of Q-learning.
Thank you for the reply. Can I contact you directly in case I have any further question? I am doing a Deep RL for robotic too, but a lot simple than yours.