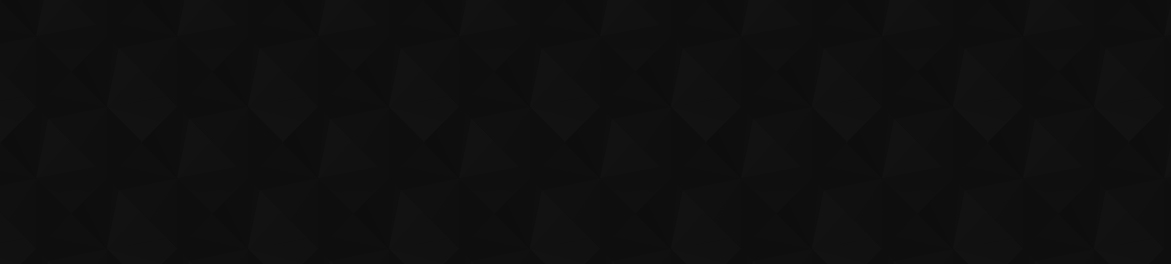
- Видео 192
- Просмотров 551 766
MIT HAN Lab
США
Добавлен 28 ноя 2018
MIT HAN Lab: Hardware, AI and Neural-nets
Accelerate Deep Learning Computing
Group website: hanlab.mit.edu
TinyML Project: tinyml.mit.edu
TinyML Course: efficientml.ai, streamed via live.efficientml.ai every Tuesday/Thursday 3:35-5:00pm ET, fall 2022.
Accelerate Deep Learning Computing
Group website: hanlab.mit.edu
TinyML Project: tinyml.mit.edu
TinyML Course: efficientml.ai, streamed via live.efficientml.ai every Tuesday/Thursday 3:35-5:00pm ET, fall 2022.
EfficientML.ai Lecture 1 - Introduction (MIT 6.5940, Fall 2024)
EfficientML.ai Lecture 1 - Introduction (MIT 6.5940, Fall 2024)
Lecture 1: Introduction
Instructor: Prof. Song Han
Slides: efficientml.ai
Lecture 1: Introduction
Instructor: Prof. Song Han
Slides: efficientml.ai
Просмотров: 1 240
Видео
EfficientML.ai Lecture 1 - Introduction (MIT 6.5940, Fall 2024, Zoom Recording)
Просмотров 57121 час назад
EfficientML.ai Lecture 1 - Introduction (MIT 6.5940, Fall 2024) Lecture 1: Introduction Instructor: Prof. Song Han Slides: efficientml.ai
ISCA 2024 "Atomique: A Quantum Compiler for Reconfigurable Neutral Atom Arrays" by Hanrui Wang
Просмотров 3922 месяца назад
ISCA 2024 Atomique: A Quantum Compiler for Reconfigurable Neutral Atom Arrays Hanrui Wang, Pengyu Liu, Daniel Bochen Tan, Yilian Liu, Jiaqi Gu, David Z. Pan, Jason Cong, Umut Acar, Song Han The neutral atom array has gained prominence in quantum computing for its scalability and operation fidelity. Previous works focus on fixed atom arrays (FAAs) that require extensive SWAP operations for long-...
MLSys'24 Best Paper - AWQ: Activation-aware Weight Quantization for LLM Compression and Acceleration
Просмотров 1,6 тыс.3 месяца назад
Talk video for MLSys 2024 Best Paper: "AWQ: Activation-aware Weight Quantization for LLM Compression and Acceleration" (May 14th at Santa Clara Convention Center, CA, US) Ji Lin*, Jiaming Tang*, Haotian Tang†, Shang Yang†, Wei-Ming Chen, Wei-Chen Wang, Guangxuan Xiao, Xingyu Dang, Chuang Gan, Song Han For more info, please visit: AWQ website: hanlab.mit.edu/projects/awq Paper: arxiv.org/abs/230...
DistriFusion: Distributed Parallel Inferencefor High-Resolution Diffusion Models
Просмотров 6303 месяца назад
In this video, we introduce DistriFusion, a training-free algorithm to harness multiple GPUs to accelerate diffusion model inference without sacrificing image quality. It can reduce SDXL latency by up to 6.1× on 8 A100s. Our work has been accepted by CVPR 2024 as a highlight.
Condition-Aware Neural Network for Controlled Image Generation
Просмотров 2543 месяца назад
Condition-Aware Neural Network for Controlled Image Generation CVPR'24
GazeSAM Demo: Combining EfficientViT-SAM with Gaze Estimation
Просмотров 9813 месяца назад
github.com/mit-han-lab/efficientvit/tree/master/demo/gazesam
Hanrui Wang's PhD Defense, Toward Practical Quantum Computing Systems @ MIT
Просмотров 8414 месяца назад
Hanrui Wang completed his PhD degree from MIT EECS in May 2024, advised by Prof. Song Han. He received his Bachelor of Engineering degree from Fudan University in 2018 and Master of Science degree from MIT in 2020. His research focuses on architecture and system-level supports for quantum computing, and AI for quantum. His work appears in conferences such as ISCA, MICRO, HPCA, QCE, DAC, ICCAD, ...
TinyML Demo on Microcontrollers (short)
Просмотров 5914 месяца назад
TinyML Demo on Microcontrollers (short)
TinyChat Computer running Llama2-7B Jetson Orin Nano. Key technique: AWQ 4bit quantization.
Просмотров 1,7 тыс.4 месяца назад
AWQ and TinyChat: Activation-aware Weight Quantization for LLM Compression and Acceleration on Edge Device (Jetson Orin Nano) Large language models (LLMs) have shown excellent performance on various tasks, but the astronomical model size raises the hardware barrier for serving (memory size) and slows down token generation (memory bandwidth). In this paper, we pro- pose Activation-aware Weight Q...
[Demo] On-Device Training Under 256KB Memory
Просмотров 2 тыс.6 месяцев назад
This is the demo for our paper: On-Device Training Under 256KB Memory. We are transferring an ImageNet-pretrained model to the VWW dataset (person/no person) by updating the model within the tight memory budget.
Ji Lin's PhD Defense, Efficient Deep Learning Computing: From TinyML to Large Language Model. @MIT
Просмотров 9 тыс.7 месяцев назад
Ji Lin completed his PhD degree from MIT EECS in December 2023, advised by Prof. Song Han. His research focuses on efficient deep learning computing for ML and accelerating large language models (LLMs). Ji is pioneering the research in the field of TinyML, including MCUNet, MCUNetV2, MCUNetV3 (on-device training), AMC, TSM (highlighted and integrated by NVIDIA), AnyCost GAN. Recently, he propos...
EfficientML.ai Lecture 23: Noise Robust Quantum ML (MIT 6.5940, Fall 2023, Zoom)
Просмотров 1,9 тыс.9 месяцев назад
EfficientML.ai Lecture 23: Noise Robust Quantum ML (MIT 6.5940, Fall 2023, Zoom) Instructor: Hanrui Wang Slides: efficientml.ai
EfficientML.ai Lecture 23: Noise Robust Quantum ML (MIT 6.5940, Fall 2023)
Просмотров 9729 месяцев назад
EfficientML.ai Lecture 23: Noise Robust Quantum ML (MIT 6.5940, Fall 2023) Instructor: Hanrui Wang Slides: efficientml.ai
EfficientML.ai Lecture 22: Quantum Machine Learning (MIT 6.5940, Fall 2023, Zoom)
Просмотров 9329 месяцев назад
EfficientML.ai Lecture 22: Quantum Machine Learning (MIT 6.5940, Fall 2023, Zoom) Instructor: Hanrui Wang Slides: efficientml.ai
EfficientML.ai Lecture 22: Quantum Machine Learning (MIT 6.5940, Fall 2023)
Просмотров 9829 месяцев назад
EfficientML.ai Lecture 22: Quantum Machine Learning (MIT 6.5940, Fall 2023)
EfficientML.ai Lecture 21: Basics of Quantum Computing (MIT 6.5940, Fall 2023)
Просмотров 1,8 тыс.9 месяцев назад
EfficientML.ai Lecture 21: Basics of Quantum Computing (MIT 6.5940, Fall 2023)
EfficientML.ai Lecture 21: Basics of Quantum Computing (MIT 6.5940, Fall 2023, Zoom)
Просмотров 1,3 тыс.9 месяцев назад
EfficientML.ai Lecture 21: Basics of Quantum Computing (MIT 6.5940, Fall 2023, Zoom)
EfficientML.ai Lecture 20: Efficient Fine-tuning and Prompt Engineering (MIT 6.5940, Fall 2023)
Просмотров 2,3 тыс.9 месяцев назад
EfficientML.ai Lecture 20: Efficient Fine-tuning and Prompt Engineering (MIT 6.5940, Fall 2023)
EfficientML.ai Lecture 20: Efficient Fine-tuning and Prompt Engineering (MIT 6.5940,Fall 2023,Zoom)
Просмотров 1,2 тыс.9 месяцев назад
EfficientML.ai Lecture 20: Efficient Fine-tuning and Prompt Engineering (MIT 6.5940,Fall 2023,Zoom)
EfficientML.ai Lecture 19: On-Device Training and Transfer Learning (MIT 6.5940, Fall 2023)
Просмотров 1,6 тыс.9 месяцев назад
EfficientML.ai Lecture 19: On-Device Training and Transfer Learning (MIT 6.5940, Fall 2023)
EfficientML.ai Lecture 19: On-Device Training and Transfer Learning (MIT 6.5940, Fall 2023, Zoom)
Просмотров 8669 месяцев назад
EfficientML.ai Lecture 19: On-Device Training and Transfer Learning (MIT 6.5940, Fall 2023, Zoom)
MICRO'23 PockEngine: Sparse and Efficient Fine-tuning in a Pocket
Просмотров 8159 месяцев назад
MICRO'23 PockEngine: Sparse and Efficient Fine-tuning in a Pocket
EfficientML.ai Lecture 18: Distributed Training (Part II) (MIT 6.5940, Fall 2023, Zoom)
Просмотров 9569 месяцев назад
EfficientML.ai Lecture 18: Distributed Training (Part II) (MIT 6.5940, Fall 2023, Zoom)
EfficientML.ai Lecture 18: Distributed Training (Part II) (MIT 6.5940, Fall 2023)
Просмотров 1,6 тыс.9 месяцев назад
EfficientML.ai Lecture 18: Distributed Training (Part II) (MIT 6.5940, Fall 2023)
EfficientML.ai Lecture 17: Distributed Training (Part I) (MIT 6.5940, Fall 2023, Zoom)
Просмотров 1,5 тыс.10 месяцев назад
EfficientML.ai Lecture 17: Distributed Training (Part I) (MIT 6.5940, Fall 2023, Zoom)
EfficientML.ai Lecture 17: Distributed Training (Part I) (MIT 6.5940, Fall 2023)
Просмотров 2,6 тыс.10 месяцев назад
EfficientML.ai Lecture 17: Distributed Training (Part I) (MIT 6.5940, Fall 2023)
EfficientML.ai Lecture 16 - Diffusion Model (MIT 6.5940, Fall 2023, Zoom)
Просмотров 1,6 тыс.10 месяцев назад
EfficientML.ai Lecture 16 - Diffusion Model (MIT 6.5940, Fall 2023, Zoom)
EfficientML.ai Lecture 16 - Diffusion Model (MIT 6.5940, Fall 2023)
Просмотров 6 тыс.10 месяцев назад
EfficientML.ai Lecture 16 - Diffusion Model (MIT 6.5940, Fall 2023)
EfficientML.ai Lecture 15 - GAN, Video, and Point Cloud (MIT 6.5940, Fall 2023, Zoom)
Просмотров 83810 месяцев назад
EfficientML.ai Lecture 15 - GAN, Video, and Point Cloud (MIT 6.5940, Fall 2023, Zoom)
Can you please share the link to the ppt ?
Its hard to see in this recording, but an other video posted by UPenn has the screen view along with the audio ruclips.net/video/5v9wa-UCFMM/видео.html
@@kiase978thanks 😊
would you tell me how to determine the value of k(top-k)? by train in pytorch?
Hi bang bang, thanks for your interest in our work! The k is a hyper-parameter we need to determine ahead of time. For example, if we choose 50% pruning ratio for a 20-token sentence, then the k will be 10.
Can u send the code on this email please sir athaarhmo@gmail.com