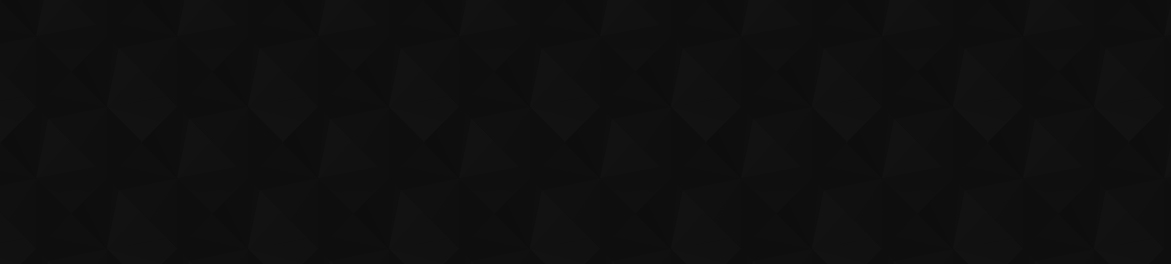
- Видео 28
- Просмотров 55 021
MFANS
Добавлен 29 сен 2020
The Official RUclips Channel of the Michigan Football Analytics Society (MFANS)
MSAP Episode 8- Football: Penalty Analytics w/ Joseph Hefner
In this football episode, Jake Grossman and Joseph Hefner discuss QB accuracy metrics, talk about the Chiefs are stacking up in their attempt to three-peat, and review some penalty data to see which teams have been lucky/unlucky so far this season.
Просмотров: 40
Видео
MSAP Episode 7- Football: Creating A New Currency For Draft Pick Valuation w/ Josh Queipo
Просмотров 392 месяца назад
In this football episode, Jake Grossman and Josh Queipo discuss the concept of positional value, break down the playoff picture as it stands and the recently-signed Deommodore Lenoir extension, and discuss Josh's research valuing draft picks and player performance.
MSAP Episode 6- Football: College Football Recruiting Rankings w/ Conor McQuiston
Просмотров 292 месяца назад
In this football episode, Jake and Conor break down field goal probability models, consider the state of the NFL and whether there is a QB development problem, and discuss Conor's recruiting rankings and other interesting college football research on his blog, The Evaluation Period. Link: www.evaluationperiod.info/
MSAP Episode 5- Football: NFL Draft Curves w/ Ryan Brill
Просмотров 772 месяца назад
In this football episode, Jake Grossman and Ryan Brill discuss the intricacies of fourth-down decision models, analyze the New Orleans Saints' decision to fire Dennis Allen, and dive into Ryan's new research on NFL Draft curves.
MSAP Episode 4- Football: Correlating Tail Outcomes w/ Tej Seth
Просмотров 433 месяца назад
In this football episode, Jake Grossman and Tej Seth discuss tracking data metrics, Bite Distance Under Expected and Ground Covered Over Expected, review the first half of the NFL season and make predictions for the second half, and outline how an NFL team might approach correlating its tail outcomes.
MSAP Episode 3- Football: Trade deadline and more w/ Daniel Salib
Просмотров 573 месяца назад
In this extended football episode, Jake Grossman and Daniel Salib explore the differences between cash and cap spending, analyze the recent trades for Davante Adams and Amari Cooper, share predictions for the upcoming trade deadline, and break down the extension market for this season as well as the free agency landscape for next offseason.
MSAP Episode 2- Football: New PFF Stats w/ Weller Ross
Просмотров 483 месяца назад
In our debut football-focused episode, Jake Grossman and Weller Ross break down PFF grades, tackle the latest NFL news surrounding the Jets and Patriots, and explore the wealth of new metrics now available on PFF’s site.
MSAP Episode One: Welcome to the Michigan Sports Analytics Podcast!
Просмотров 824 месяца назад
Welcome and thank you for joining us for the very first episode of the Michigan Sports Analytics podcast! Our opening discussion dives into WAR as a stat in both baseball and football, the greatness of Shohei Ohtani, the state of NFL offense, rule changes, and more!
K-Nearest Neighbors tutorial using NFL Combine data in R
Просмотров 4999 месяцев назад
Github: github.com/arjunmenon10/MFANS-Coding-Tutorials/blob/main/KNNImputationtutorial.R
Data Manipulation & Data Visualization with NFL Coverage Data in R (2023)
Просмотров 1,4 тыс.Год назад
Github link: github.com/arjunmenon10/MFANS-Coding-Tutorials/blob/main/coveragedatatutorial.R
MFANS: Scouting Team Tendencies with NFL Data in R (2023)
Просмотров 1,7 тыс.Год назад
Github link: github.com/arjunmenon10/MFANS-Coding-Tutorials/blob/main/playerrunpasstutorial.R
Logistic Regression Modeling With NFL Data in Python (2023)
Просмотров 4,1 тыс.Год назад
Code: github.com/tejseth/nfl-tutorials-2022/blob/master/nfl_data_py_2.ipynb
Introduction to NFL Data with Python (2023)
Просмотров 16 тыс.2 года назад
Code: github.com/tejseth/nfl-tutorials-2022/blob/master/nfl_data_py_1.ipynb
M-FANS: Making a Random Forest and XGBoost Model with NFL Data
Просмотров 2,6 тыс.2 года назад
Github: github.com/tejseth/nfl-tutorials-2022/blob/master/4-random-forest-xgboost.R
M-FANS: Making Logistic and Linear Regression Models with Football Data
Просмотров 2,6 тыс.2 года назад
Github: github.com/tejseth/nfl-tutorials-2022/blob/master/3-linear-regression-modeling.R
M-FANS: Data Viz with NFL Data (2022)
Просмотров 1,9 тыс.2 года назад
M-FANS: Data Viz with NFL Data (2022)
M-FANS: Introduction to NFL Data with R (2022)
Просмотров 7 тыс.2 года назад
M-FANS: Introduction to NFL Data with R (2022)
Random Forest Modeling Using NFL Data
Просмотров 1 тыс.3 года назад
Random Forest Modeling Using NFL Data
Tutorial #3: Basic Modeling Using NFL Data
Просмотров 1,7 тыс.3 года назад
Tutorial #3: Basic Modeling Using NFL Data
Data Viz in R tutorial with nflfastR
Просмотров 1,5 тыс.3 года назад
Data Viz in R tutorial with nflfastR
MFANS Technical Meeting 11/17/2020 Modelling in Python
Просмотров 2554 года назад
MFANS Technical Meeting 11/17/2020 Modelling in Python
MFANS Technical Meeting 10/27/2020 - Intro to Python with nflfastR Data
Просмотров 4944 года назад
MFANS Technical Meeting 10/27/2020 - Intro to Python with nflfastR Data
MFANS Technical Meeting 10/13/2020 - Intro to Linear Modelling in R with nflfastR
Просмотров 4854 года назад
MFANS Technical Meeting 10/13/2020 - Intro to Linear Modelling in R with nflfastR
MFANS Technical Meeting 10/6/2020 - Intro to R Data Visualization with R
Просмотров 4184 года назад
MFANS Technical Meeting 10/6/2020 - Intro to R Data Visualization with R
MFANS Technical Meeting 9/29/2020 - Intro to R with nflfastR
Просмотров 1,4 тыс.4 года назад
MFANS Technical Meeting 9/29/2020 - Intro to R with nflfastR
Are the games rigged?
Great pod and great content recently, big fan. This channel deserves more recognition for sure
great video - I wish this channel was still making content of this caliber.
Tej is the 🐐
I want a video on soccer I have to submit project tomorrow
Nice video Arjun, would love to see more on this channel. Especially if any Python videos are in the works! (Otherwise I'm gonna have to learn R too!)
Any plans to do any other videos? Would love to see more stuff in Python
Any plans to do anymore of these in Python?
I'm at 33:11 but 3 of my team graphics (Packers, Raiders, and Jets) are still huge while all the others are small like in the example. I even copied and pasted the last few cells of code from the premade notebook into the one I'm coding along in. Even if I run the code in the premade notebook 2 of the team logos are huge on the EPA chart. Anyone have any advice? This is the notebook I've been building while following along: colab.research.google.com/drive/1kA0TBNMKZlGkhtndPSvPu3fvDuPGNHpX?usp=sharing
I fixed it by using the code below. The graphics for GB, LV, and NYJ were 4096x4096 while the others were 500x500. !pip install --upgrade Pillow #Import the Image module from the PIL library from PIL import Image import matplotlib.pyplot as plt from matplotlib.offsetbox import OffsetImage, AnnotationBbox plt.rcParams["figure.figsize"] = [15, 9] plt.rcParams["figure.autolayout"] = True def getImage(path): # Open the image with PIL img = Image.open(path) # Resize the image to 500x500 using Image.LANCZOS img = img.resize((500, 500), Image.LANCZOS) # Convert the PIL image to a format that matplotlib understands return OffsetImage(img, zoom=.1) x = epa_with_logos['pass_epa'] y = epa_with_logos['rush_epa'] paths = epa_with_logos['logo_path'] fig, ax = plt.subplots() for x0, y0, path in zip(x, y, paths): ab = AnnotationBbox(getImage(path), (x0, y0), frameon=False) ax.add_artist(ab) plt.xlim(-0.2, 0.3) plt.ylim(-0.25, 0.15) plt.title("Rush and Pass EPA, 2023") plt.xlabel("EPA/Pass") plt.ylabel("EPA/Rush") plt.show()
Trying to figure out why it's listing 36 teams (0 thru 35) in the 2022 NFL season.
Hi - Are you still reviewing and by chance answering questions about the code here. I am brand new to python and coding really. i am trying to load in all data for the last 5 years and predict how many rushing yards someone will have in an upcoming game. Basically trying to validate the Over / Under for Rush Yards against what DraftKings has. Do you have any code or videos that would help pull this specific data. I am using this video as a starter. getting there I think, but if you have anything specific around specific data types, it would be helpful
DMwR has been deprecated D:
This is awesome. Thank you for doing it. Do you know why some of the logos did not scale down for me?
I have the same Problem !
I have the same issue (Raiders, Packers & Jets)
Same issue here. Any fix?
I pre-processed the images to same size using numpy and pillow "PIL" to size all the images to same size.
Continuing my review of AI/ML after about 6 weeks with Deep Learning and PyTorch I have been looking to upgrade my Colts By Numbers website with some interesting comparisons and projections. In the last few days I'm sorting out the models and figuring out data splits, model training and predictions. Probably looking at time series visualizations. This was very helpful. Getting a nice project plan and will probably use nfl_data_py to train some models on some ideas. Then when the regular season starts pull current season data, run predictions and make some cool comparisons and projections and rotate feature stats.
U should be using environments right? Doesn’t pip install with no environment plug up your pc in the long run? Also u want the environment to explain in a readme file what u where using when it worked. Let me kmow
I don’t like just installing personally
I dig the teaching do it in python
Bro why u using r as a primary’s
Came back to this video (6/18/2024) right after the NumPy 2.0.0 version release. I am brand new to coding, but for this program to work, you have to have NumPy version 1.26.4 to work. If you do a simple Gemini/ ChatGPT search on how to change NumPy verions, its quite simple Best of luck to all!
The little squiggly thing 🤣🤣
Is there a way I can get the raw pbp data that you use?
one thing I discovered while running this with 2023 numbers was that B. Robinson was counted as Bijan and Brian.. so for me the targets total was 129 (combined of the two) and it seemed to do the same with their other stats. Is there a better way to grab their names without just using first initial and last name?
I ran into the same issue. With the help from AI, I was able to split Bijan and Brian. pbp_rp['receiver_player_name'] = pbp_rp.apply(lambda row: 'Bijan Robinson' if row['receiver_player_name'] == 'B.Robinson' and row['posteam'] == 'ATL' else ('Brian Robinson' if row['receiver_player_name'] == 'B.Robinson' and row['posteam'] == 'WAS' else row['receiver_player_name']), axis=1) pbp_rp['rusher_player_name'] = pbp_rp.apply(lambda row: 'Bijan Robinson' if row['rusher_player_name'] == 'B.Robinson' and row['posteam'] == 'ATL' else ('Brian Robinson' if row['rusher_player_name'] == 'B.Robinson' and row['posteam'] == 'WAS' else row['rusher_player_name']), axis=1)
Hey this is great. What is the best way to get updated data for this past season 23-24?
The nfl python packages update automatically so to get updated data you just have to reload the packages
This is super cool man. Thanks for sharing. I'm just getting started but having someone explain code like this is easily my best way to learn.
Sadly NFL participation data is no longer supported outside of PrimeTime games - do you plan to source this data from somewhere else?
unfortunately I can't really control that so we're left with just primetime games after week 15. Not much on my end I can do about it since it's a Next Gen Stats thing :(
I'm getting stuck at the very beginning because the load_participation function is giving me this error: Error in data.table::setkeyv(pbp, c("game_id", "play_id")) : some columns are not in the data.table: game_id,play_id Any idea how to fix this?
Appreciate these brotha! Best of luck to you
The name of the symbols used to quote column names is backticks (or grave accent). Great video. Thanks for it. I’m keen on your next tutorial.
I opened colab and ran the code and am getting this error ModuleNotFoundError: No module named 'nfl_data_py'
I had to remove the # first, I think it was ignoring the import because it thought it was a comment
@@gilbster thanks I appreciate your help!
Is there a website where we can follow your work more consistently
Great stuff
Great job, Arjun! Would love to see more of these.
Great job - always love the analysis and insights
Thanks for your introduction video. I wanted to create a formula that shows QB EPA without YAC oe from the receiver. This is my formula, but it doesn't work: nflfastR::load_pbp(2023) %>% dplyr::group_by(passer, passer_id, posteam) %>% dplyr::mutate(attempts = sum(pass_attempt)) %>% dplyr::filter(attempts >= 100) %>% dplyr::summarize( epa_qb = mean(qb_epa + xyac_epa - yac_epa), qb_epa = mean(qb_epa), xyac_epa = mean(xyac_epa), yac_epa = mean(yac_epa), yac_oe = mean(yac_epa - xyac_epa), att = dplyr::n() ) %>% dplyr::ungroup() %>% dplyr::select(passer, posteam, att, epa_qb, qb_epa, yac_epa, xyac_epa, yac_oe) %>% dplyr::arrange(-epa_qb) %>% utils::head(32) %>% knitr::kable(digits = 3) Can you pls fix it? Thank you!
Do you have a video on quaterback cummulative epa over many seasons?
It is in the video right before this im pretty sure. Titled "Data Viz in R" or something like that
GO SEAHAWKS!
Love this! Happy I randomly found your work - It's very well thought out and presented!
This is awesome, learned a lot. Thanks for sharing.
Can you make more videos of python and nfl? 🥺
Great video, very helpful information for a novice who's trying to learn.
Enjoyed this young man...thanks for teaching an old guy some new tricks 👍
Will this auto update throughout the season?
Really cool
Thank you so much for these videos. I’m recently getting into tech and was struggling to find projects that’d keep my interest. You’ve given me many ideas and I’ll be back to thank you again after I land a job in Data Science.
This was perfect! thanks so much for doing this. looking forward to future videos!
Great stuff Tej!
Tej, this is amazing. You are one of, if not the only, one doing this sort of work for NFL. In my studying, you have helped me immensely, and I hope that you continue to share your work through RUclips. I am hoping for your success brother.
Awesome tutorial!!
Super helpful demo and very easy to follow! Question-will this type of data be available in-season? Like could I add 2023 to my season parameter after week 1 is complete or would I have to wait until season is over to get any 2023 data?
yes it’s available during the season!
Great stuff! Thank you for sharing
Amazing! Thank you so much