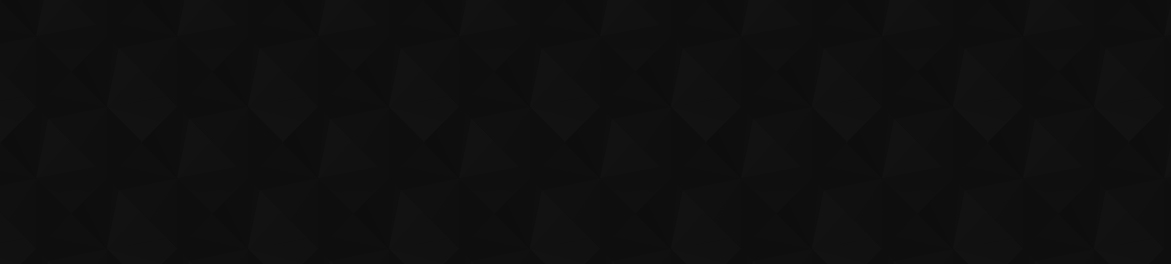
- Видео 98
- Просмотров 217 964
Zardoua Yassir
Добавлен 9 апр 2015
Demo: A Siamese Object Tracker Adapted for 360° Videos
This video demonstrates the result of a single object tracking algorithm that I have adapted from regular 2D tracking to work on 360° videos. The original 2D tracking algorithm is described in the paper titled: Joint Classification and Regression for Visual Tracking with Fully Convolutional Siamese Networks.
The result at 1:14 is a projection of the original 360° video, centered on the target of interest. The tracking result in this video is based on material from the original 360° video linked below:
• Title: 2019 04 28 vr360m dog walk ford lake park
• Thanks to Channel Owner: @wenelson1968 for allowing video reuse.
The result at 1:14 is a projection of the original 360° video, centered on the target of interest. The tracking result in this video is based on material from the original 360° video linked below:
• Title: 2019 04 28 vr360m dog walk ford lake park
• Thanks to Channel Owner: @wenelson1968 for allowing video reuse.
Просмотров: 52
Видео
10- NE555: diodes de protection (partie1/2)
Просмотров 9313 года назад
10- NE555: diodes de protection (partie1/2)
1- Comparateur à un seul seuil partie (1/3)
Просмотров 6 тыс.3 года назад
1- Comparateur à un seul seuil partie (1/3)
11- NE555: diodes de protection (partie2/2)
Просмотров 6773 года назад
11- NE555: diodes de protection (partie2/2)
3- Comparateur à un seul seuil (partie3/3)
Просмотров 1,5 тыс.3 года назад
3- Comparateur à un seul seuil (partie3/3)
2- Comparateur à un seul seuil partie (2/3)
Просмотров 2,2 тыс.3 года назад
2- Comparateur à un seul seuil partie (2/3)
7- Astable à base d'amplificateur opérationnel partie (2/2)
Просмотров 1,9 тыс.3 года назад
7- Astable à base d'amplificateur opérationnel partie (2/2)
13- NE 555: circuit astable partie (2/2)
Просмотров 2,7 тыс.3 года назад
13- NE 555: circuit astable partie (2/2)
14- Oscillateurs électroniques: rappel sur fonction de transfert réelle et complexe.
Просмотров 8733 года назад
14- Oscillateurs électroniques: rappel sur fonction de transfert réelle et complexe.
15- Oscillateurs électronique: Gain en boucle
Просмотров 5393 года назад
15- Oscillateurs électronique: Gain en boucle
12- NE 555: circuit astable partie (1/2)
Просмотров 12 тыс.3 года назад
12- NE 555: circuit astable partie (1/2)
6- Astable à base d'amplificateur opérationnel partie (1/2)
Просмотров 3,2 тыс.3 года назад
6- Astable à base d'amplificateur opérationnel partie (1/2)
8- NE555: principe de fonctionnement
Просмотров 4,5 тыс.3 года назад
8- NE555: principe de fonctionnement
4- Comparateur à deux seuils : Trigger de Schmitt - partie (1/2)
Просмотров 7 тыс.3 года назад
4- Comparateur à deux seuils : Trigger de Schmitt - partie (1/2)
16- Oscillateurs électroniques: bruit thermique
Просмотров 7493 года назад
16- Oscillateurs électroniques: bruit thermique
17- Oscillateur à pont de Wien : circuit et fonctionnement
Просмотров 1,1 тыс.3 года назад
17- Oscillateur à pont de Wien : circuit et fonctionnement
5- Comparateur à deux seuils : Trigger de Schmitt - partie (2/2)
Просмотров 2,7 тыс.3 года назад
5- Comparateur à deux seuils : Trigger de Schmitt - partie (2/2)
3- Alimentation de l'amplificateur opérationnel (rappel pratique)
Просмотров 3 тыс.3 года назад
3- Alimentation de l'amplificateur opérationnel (rappel pratique)
2- Alimentation de l'amplificateur opérationnel (rappel théorique)
Просмотров 1,6 тыс.3 года назад
2- Alimentation de l'amplificateur opérationnel (rappel théorique)
5- GBF (Générateur Basses Fréquences)
Просмотров 1,4 тыс.3 года назад
5- GBF (Générateur Basses Fréquences)
(12/12) MobileNets: MobileNetV2 (Part5)
Просмотров 3,6 тыс.3 года назад
(12/12) MobileNets: MobileNetV2 (Part5)
(11/12) MobileNets: MobileNetV2 (Part4)
Просмотров 3,2 тыс.3 года назад
(11/12) MobileNets: MobileNetV2 (Part4)
(10/12) MobileNets: MobileNetV2 (Part3)
Просмотров 4 тыс.3 года назад
(10/12) MobileNets: MobileNetV2 (Part3)
(9/12) MobileNets: MobileNetV2 (Part2)
Просмотров 6 тыс.3 года назад
(9/12) MobileNets: MobileNetV2 (Part2)
(8/12) MobileNets: MobileNetV2 (Part1)
Просмотров 24 тыс.3 года назад
(8/12) MobileNets: MobileNetV2 (Part1)
(7/12) MobileNets: MobileNetV1: Resolution Multiplier and Key Results
Просмотров 9993 года назад
(7/12) MobileNets: MobileNetV1: Resolution Multiplier and Key Results
Awesome vid to get familiarized with MNv2 arquitecture!
5 likes | 4 years ago why did not continue posting videos ?
thanks for the explanation :)
are you alive?
great video, thanks for sharing this. May i ask what does "2D filter" mean in the video description and why it only takes 5% of the computational load? Thank you in advance.
merci bcp 😁
de rien, bonne chance !
Underrated 🫡
Thanks 😁
Merci, j'ai un TP de partiels Mardi et j'avais pas particulièrement envoie de tombé sur des AOP sans savoir faire les branchements nécessaire. C'était très Claire, Merci
@@shiimadraw3155 bonne chance
You are a legend. I watched all 12 episodes and they were amazing. I always searched for someone that can explain the why rather than the how. Thank you so much :) You're the best.
The 'why' is key. Once you understand it, the brain quickly grasps the 'how.' Good luck my friend!
great video really love your way of explaining the things very intuitively. Would enjoy if you would do more of such series. F.e. about yolo and all the progressions. ;)
Thank you! I clear with your clip.
Merci vous me sauvé la vie
Thanks for the nice lecture. I have doubt you said that low dimensional activation can alone solve the problems we donot need much channels so whole thing is to reduce number of channels thats why we are going from d to d' but the problem is we cannot apply relu on lower dimensional activation hence we first increase the dimension and then applied relu and then again converted back to lower dimensional . but in lecture you are saying that d is small . but how can we get d small.? are we using less number for kernels for getting d smaller.
@@none-hr6zh I don't remeber the specific notations but I see ur question is basically how do we get less channels (i.e., compress a feature map). Well yes we use less kernels because each kernel outptus one channel, so more kernels means more output channels. Less kernels means less output channels. Let me know if u have more doubts
Very very nice. I went through hell to find you.
@@wb7779 Feel free to post any question if you need help with the next videos. Good luck
great video really helped
Great series so far. Loving it
Thanks. Enjoy the rest
Great explanation but i have doubt. My dataset gas three features. Now im interested increase the dimension of this. Let us say my old dataset as 'x'. If use the polynomial kernel with degree 2 means, (1+x.(x.T))**2 right? My question is if we are doing the dot product means ( i mean my dataset shape is 200x3 and if transpose it means it become 3x200 and if do x.T*x then ill get 3x3 right). So ny question is where the dimensions are increased?
no where ur dimensions will be increased because ur doing the wrong math. dot product takes two input vectors, not matrices. x should denote a feature vector, not a dataset.
@@zardouayassir7359 but my dataset is non linear. I have already performed soft margin svm with the scratch code. But the soft margin allows more misclassifications due to the type of data. Now I'm interested to increase the dimension. If I want to use rbf or any other kernel, what should I do technically for increasing the dimension?
@@gunasekhar8440 "but my dataset is non linear". My answer to you applies regardless of your dataset properties. Tye kernel trick I'm explaining here is used for non linear boundaries. What you need technically is first to get ur math right. Good luck
V-=Vc ?
Thank you for clear explanation, this is great
Thanks for the sorted explanation......my all doubts got cleared by your video
your explanation is awesome bro! can u please tell me where did u get all these points(not in paper) . can u refer any resources?
Happy to help. "where did u get all these points": as far as I remember, I did the following while creating this series: * Elaborate the key points in the paper by reading relevant references (such as the idea of depthwise seperable convolution) * Consult books to double check (I didn't find any book discussing this matter) * Look for concepts I've heard about for the first time (like the Manifold of Interest concept in MobileNetV2) * Once I grasp a concept, I may try to convey it with my own examples (such as the knobs controlling the pixels of a TV) * I have even asked a question on ResearchGate, and contacted some AI engineers on FB to discuss or double check my understanding. The original paper does not contain all of this stuff. In fact, the authors did a terrible job at explaining their idea, and sometimes they use expressions that are not clear. For instance, if my memory is correct, the authors in MobileNetV2 said that ReLU collapses the activations. What does "collapse" mean exactly? All I could do is guessing that it means clipping negative values (in ReLU). The authors had multiple chances to clearly explain their idea but they didn't. This happens in a lot of scientific papers. Once I read the entire paper, I realize that it's easy to explain the core idea in the abstract only, but the authors just don't do it and let you wast your time by going over the entire Flowchart and description of their algorithm to infer their key idea. Hope this helps
this is my second time watching this series, thank you so much. I wish you could explain more interesting deep-learning architecture like this.
Ur welcome
Very useful. Thanks a lot for this content Sir
I go over the entire web and didn't find an explanation for MobileNetV2 except your videos Your explanation is great, detailed, and easy to understand thank you very much
I appreciate your feedback. Thank you
Pas besoin tu as déjà un mode série qui connecte les borne en interne
Le besoin initial d'un étudiant n'est pas l'économie des connexions mais plutôt la clarté. Nous fournissons deux alimentations DC indépendantes à certains étudiants et tu ne pourras pas choisir le mode SERIES.
Thank you so much for making this topic understandable so easily.
Thats you so much for the Series Very clean explanation of the MobileNet v2 paper
merci beaucoup
merci beaucoupppp❤
Bonne chance
جزاك الله خيرا
بالتوفيق
Merci
Bonne chance
Can you please make a video like this but with MobileNetv3? 🥺🙏
I appreciate that you wanted a video from my channel. My time is quite limited at the moment, but I'll definitely consider your request. Thanks for your understanding
how do we choose a kernel and can we expect to find such a kernel that can arrive at the correct inner product within the feature space?
You can identify the kernel for your SVM based on empirical testing. It's better to start with simpler kernels first, then move to the more complicated ones if needed.
the invertible pt s(k), summation range should be u instead of k
Correct. Thanks
it is better to consider that the dimensions of the pointwise kenel is 1×1×d×dt, where the quantity "dt" is the number of kernels and the "t" in "dt" is again the expansion factor
please read my reponse to the pinned comment. I believe I had answered there the same question. Let me know if it's not the answer you want.
I see all MobileNet videos, thanks so much for doing them, you are really clear!
Happy to help Agustina. Thank you for the comment
hi, but here the 0.784 is the accuracy per class...?, the 0.7 was the overall accuracy or for only class A...?
Your question is already answered in the video : 0.7 is the probability that each of the three classifiers (C1,2,3) woud produce the right classification. 0.784 is the probability that the predictions of the three classifiers would contain at least two correct classifications. Since the correct prediction is Class A, then the probability that the predictions of the three classifiers would contain at least two correct classifications is equivalent to the probability of getting 2A and 1B + the probability of getting 3B.
@@zardouayassir7359 thank you
@@mohamed-rayanelakehal1324 feel free to ask other questions. Good luck
merci beaucoup une bonne explication
Je ne comprends pas pourquoi ve'=ve avec la présence de R
La présence de R ne peut pas provoquer une chute de tension sans avoir un courant qui la traverse. Par exemple, si les deux diodes D1 et D2 sont bloquées, aucun courant ne passera à travers R. Avec les deux diodes D1 et D2 bloquées, vous pensez peut-être qu'il y aura un courant circulant à travers le pin 6 et le pin 2 du circuit NE, mais ces deux entrées (Pin 2 et 6) ont une résistance infinie, empéchant ainsi le courant de passer à travers la résistance R. Donc la chute de tension à travers R est nulle. Ainsi, ve = ve'.
here in start 00:20 you said it was explained it earlier video as to how it came but in previous video you just wrote we have to find max of 2/||w||?
Thank you very much. You've clearly explained everything. I really enjoyed the series. It was a great helping hand while reading the papers. Specially I was not able to understand V2 paper, the paper is difficult to understand for a lot of reasons, your video really worked like magic. Thank you again.
Thank you so much for this wonderful course, you don't know how much you helped me, I have a question about the gamma parameter, could you tell us about its effect on our model as you did with the C parameter
at 15:00 , I don't understand why we have two different indices for w when it is the same
Hi Samy, Please excuse me for my late response. Your question is related to a math issue. Let's assume we have the following expression: (Σ n) and I want to multiply it by itself like that: (Σ n) (Σ n). Now to specify the starting and ending index of each sigma, I'll use this notation: (Σim n), where the first letter ("i" in this case) and second letter ("m" in this case) right after the sigma Σ represent the starting and ending index of the sigma, respectively. With this in mind, I can rewrite (Σ n).(Σ n) as (Σim n).(Σim n). Based on math properties, we can merge the two sigmas Σ together like that: (ΣimΣjm n.n). Your question now is: why did I switch from i to j in the second sigma after merging the two sigmas? The answer is that, when merging the two sigmas, we must start from the inner sigma. I mean by "start" incrementing the index of the first sigma until the end and doing the sum after each increment. During this process (computation of the inner sigma), the outer sigma MUST HAVE A FIXED INDEX, WHICH WE INCREMENT BY ONE ONLY WHEN WE FINISH COMPUTING THE INNER SIGMA (i.e., we reach the final index of the inner sigma). Once we increment the outer sigma, we repeat the same process again (initialize the index j and re-compute the inner sigma). The computation would be complete once the index of the inner and outer sigmas both reach the maximum value ("m" in this case). This mechanism is not possible if we keep the same index "i" for both sigmas because as soon as I increment the index of the inner sigma, the index of the outer sigma will be incremented along as well, which violates the process I've just described. To avoid this issue and get the desired process, we must change the variable of the inner sigma to something else ("j" in our case). Hope this helps.
Superbe vidéo merci beaucoup 👌👌👌👌👌
thank god you made a video on this, thank you
Thanks satoko
Good explaining but you might wanna use some darker color pen for writing can't see what you are writing.
Of course. Thanks
❤
MEOWING.....yes, she's meowing alright! I'd think she's a hungry hungry kitten! She's beautiful...I love animals that are vocal💖💖💖💖
so cute ❤
Just finished the playlist,it cleared up a lot of things .Thanks
Good news