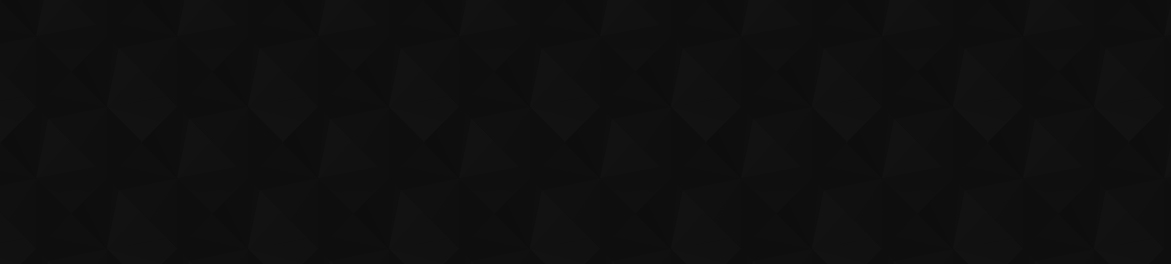
- Видео 169
- Просмотров 975 762
DataMListic
Добавлен 12 июн 2020
Welcome to DataMListic (former WhyML)! On this channel I explain various ML concepts that I encounter in my learning journey. Enjoy the ride! ;)
The best way to support the channel is to share the content. However, If you'd like to also support the channel financially, donating the price of a coffee is always warmly welcomed! (completely optional and voluntary)
► Patreon: www.patreon.com/datamlistic
► Bitcoin (BTC): 3C6Pkzyb5CjAUYrJxmpCaaNPVRgRVxxyTq
► Ethereum (ETH): 0x9Ac4eB94386C3e02b96599C05B7a8C71773c9281
► Cardano (ADA): addr1v95rfxlslfzkvd8sr3exkh7st4qmgj4ywf5zcaxgqgdyunsj5juw5
► Tether (USDT): 0xeC261d9b2EE4B6997a6a424067af165BAA4afE1a
The best way to support the channel is to share the content. However, If you'd like to also support the channel financially, donating the price of a coffee is always warmly welcomed! (completely optional and voluntary)
► Patreon: www.patreon.com/datamlistic
► Bitcoin (BTC): 3C6Pkzyb5CjAUYrJxmpCaaNPVRgRVxxyTq
► Ethereum (ETH): 0x9Ac4eB94386C3e02b96599C05B7a8C71773c9281
► Cardano (ADA): addr1v95rfxlslfzkvd8sr3exkh7st4qmgj4ywf5zcaxgqgdyunsj5juw5
► Tether (USDT): 0xeC261d9b2EE4B6997a6a424067af165BAA4afE1a
Why L1 Regularization Produces Sparse Weights
In this video, we talk about why the L1 regularization produces sparse weights.
*References*
▬▬▬▬▬▬▬▬▬▬▬▬▬▬▬▬▬▬▬▬▬▬▬▬
L1 vs L2 Regularization: ruclips.net/video/aBgMRXSqd04/видео.html
*Related Videos*
▬▬▬▬▬▬▬▬▬▬▬▬▬▬▬▬▬▬▬▬▬▬▬▬
Overfitting vs Underfitting: ruclips.net/video/B9rhzg6_LLw/видео.html
Why Models Overfit and Underfit - The Bias Variance Trade-off: ruclips.net/video/5mbX6ITznHk/видео.html
Least Squares vs Maximum Likelihood: ruclips.net/video/WCP98USBZ0w/видео.html
Why We Don't Use the Mean Squared Error (MSE) Loss in Classification: ruclips.net/video/bNwI3IUOKyg/видео.html
Hyperparameters Tuning: Grid Search vs Random Search: ruclips.net/video/G-fXV-o9QV8/видео.html
XGBoost Explained in U...
*References*
▬▬▬▬▬▬▬▬▬▬▬▬▬▬▬▬▬▬▬▬▬▬▬▬
L1 vs L2 Regularization: ruclips.net/video/aBgMRXSqd04/видео.html
*Related Videos*
▬▬▬▬▬▬▬▬▬▬▬▬▬▬▬▬▬▬▬▬▬▬▬▬
Overfitting vs Underfitting: ruclips.net/video/B9rhzg6_LLw/видео.html
Why Models Overfit and Underfit - The Bias Variance Trade-off: ruclips.net/video/5mbX6ITznHk/видео.html
Least Squares vs Maximum Likelihood: ruclips.net/video/WCP98USBZ0w/видео.html
Why We Don't Use the Mean Squared Error (MSE) Loss in Classification: ruclips.net/video/bNwI3IUOKyg/видео.html
Hyperparameters Tuning: Grid Search vs Random Search: ruclips.net/video/G-fXV-o9QV8/видео.html
XGBoost Explained in U...
Просмотров: 1 204
Видео
Overfitting vs Underfitting - Explained
Просмотров 1,1 тыс.21 день назад
In this video, we explore two of the fundamental concepts in machine learning (ML): overfitting and underfitting. *Related Videos* ▬▬▬▬▬▬▬▬▬▬▬▬▬▬▬▬▬▬▬▬▬▬▬▬ Bias-Variance Trade-Off: ruclips.net/video/5mbX6ITznHk/видео.html Why We Don't Use the Mean Squared Error (MSE) Loss in Classification: ruclips.net/video/bNwI3IUOKyg/видео.html The Bessel's Correction: ruclips.net/video/E3_408q1mjo/видео.htm...
Confidence Intervals Explained
Просмотров 706Месяц назад
In this video, we dive into confidence intervals, a fundamental concept in statistics that provides a range of values likely to contain a population parameter, such as the mean. *References* ▬▬▬▬▬▬▬▬▬▬▬▬▬▬▬▬▬▬▬▬▬▬▬▬ T-test Explained: ruclips.net/video/7KtLCXeXmiU/видео.html Z-test Explained: ruclips.net/video/u0EdFFp_U0c/видео.html *Related Videos* ▬▬▬▬▬▬▬▬▬▬▬▬▬▬▬▬▬▬▬▬▬▬▬▬ Basic Probability Dis...
Z-Test Explained
Просмотров 1,3 тыс.Месяц назад
In this video, we talk about the z-test, a statistical method used to determine whether there is a significant difference between sample data and a population mean. We also discuss how it differs from the t-test, focusing on when to use each test based on sample size and whether the population standard deviation is known. *References* ▬▬▬▬▬▬▬▬▬▬▬▬▬▬▬▬▬▬▬▬▬▬▬▬ T-test Explained: ruclips.net/video...
L1 vs L2 Regularization
Просмотров 2,2 тыс.Месяц назад
In this video, we talk about the L1 and L2 regularization, two techniques that help prevent overfitting, and explore the differences between them. *References* ▬▬▬▬▬▬▬▬▬▬▬▬▬▬▬▬▬▬▬▬▬▬▬▬ Why Weight Regularization Reduces Overfitting: ruclips.net/video/Im91WYsbZ_U/видео.html *Related Videos* ▬▬▬▬▬▬▬▬▬▬▬▬▬▬▬▬▬▬▬▬▬▬▬▬ Why Models Overfit and Underfit - The Bias Variance Trade-off: ruclips.net/video/5...
Poisson Distribution - Explained
Просмотров 1,4 тыс.Месяц назад
In this video, we talk about the Poisson distribution and how it is derived as an edge case of the Binomial distribution when the probability of success tends to 0 and the number of trials tends to infinity. *References* ▬▬▬▬▬▬▬▬▬▬▬▬▬▬▬▬▬▬▬▬▬▬▬▬ Basic Probability Distributions Explained: Bernoulli, Binomial, Categorical, Multinomial: ruclips.net/video/PPfjlRLNqEs/видео.html *Related Videos* ▬▬▬...
Basic Probability Distributions Explained: Bernoulli, Binomial, Categorical, Multinomial
Просмотров 7572 месяца назад
In this video, we talk about some of the fundamental concepts in probability theory: the marginal probability, the joint probability and the conditional probability, together with the mathematical links between them. *References* ▬▬▬▬▬▬▬▬▬▬▬▬▬▬▬▬▬▬▬▬▬▬▬▬ Combinations explained: ruclips.net/video/p8vIcmr_Pqo/видео.html *Related Videos* ▬▬▬▬▬▬▬▬▬▬▬▬▬▬▬▬▬▬▬▬▬▬▬▬ Marginal, Joint and Conditional Pro...
AI Weekly Brief - Week 2: Llama 3.2, OpenAI Voice Mode, Mira Murati leaves OpenAI
Просмотров 2853 месяца назад
AI Weekly Brief - Week 2: Llama 3.2, OpenAI Voice Mode, Mira Murati leaves OpenAI
AI Weekly Brief - Week 2: LlamaCoder, Eureka, YouTube GenAI, Pixtral 12B
Просмотров 3344 месяца назад
AI Weekly Brief - Week 2: LlamaCoder, Eureka, RUclips GenAI, Pixtral 12B
AI Weekly Brief - Week 1: OpenAI o1-preview, DataGemma, AlphaProteo
Просмотров 2474 месяца назад
AI Weekly Brief - Week 1: OpenAI o1-preview, DataGemma, AlphaProteo
Marginal, Joint and Conditional Probabilities Explained
Просмотров 8 тыс.5 месяцев назад
Marginal, Joint and Conditional Probabilities Explained
Least Squares vs Maximum Likelihood
Просмотров 20 тыс.6 месяцев назад
Least Squares vs Maximum Likelihood
AI Reading List (by Ilya Sutskever) - Part 5
Просмотров 1,4 тыс.7 месяцев назад
AI Reading List (by Ilya Sutskever) - Part 5
AI Reading List (by Ilya Sutskever) - Part 4
Просмотров 1,2 тыс.7 месяцев назад
AI Reading List (by Ilya Sutskever) - Part 4
AI Reading List (by Ilya Sutskever) - Part 3
Просмотров 1,7 тыс.7 месяцев назад
AI Reading List (by Ilya Sutskever) - Part 3
AI Reading List (by Ilya Sutskever) - Part 2
Просмотров 2,4 тыс.7 месяцев назад
AI Reading List (by Ilya Sutskever) - Part 2
AI Reading List (by Ilya Sutskever) - Part 1
Просмотров 17 тыс.7 месяцев назад
AI Reading List (by Ilya Sutskever) - Part 1
Vector Database Search - Hierarchical Navigable Small Worlds (HNSW) Explained
Просмотров 9 тыс.8 месяцев назад
Vector Database Search - Hierarchical Navigable Small Worlds (HNSW) Explained
Singular Value Decomposition (SVD) Explained
Просмотров 4,3 тыс.8 месяцев назад
Singular Value Decomposition (SVD) Explained
Sliding Window Attention (Longformer) Explained
Просмотров 3 тыс.9 месяцев назад
Sliding Window Attention (Longformer) Explained
BART Explained: Denoising Sequence-to-Sequence Pre-training
Просмотров 2,2 тыс.9 месяцев назад
BART Explained: Denoising Sequence-to-Sequence Pre-training
RLHF: Training Language Models to Follow Instructions with Human Feedback - Paper Explained
Просмотров 1 тыс.10 месяцев назад
RLHF: Training Language Models to Follow Instructions with Human Feedback - Paper Explained
Chain-of-Verification (COVE) Reduces Hallucination in Large Language Models - Paper Explained
Просмотров 74310 месяцев назад
Chain-of-Verification (COVE) Reduces Hallucination in Large Language Models - Paper Explained
The Era of 1-bit LLMs: All Large Language Models are in 1.58 Bits - Paper Explained
Просмотров 2,6 тыс.10 месяцев назад
The Era of 1-bit LLMs: All Large Language Models are in 1.58 Bits - Paper Explained
Amazing video ✨
this is awesome
Thanks!
Why is grid search computationally more expensive - it depends on how many grid points there are - if your random search has more random hp picks, then the random search takes longer, no?
Both can be computationally expensive! In practice however, what i've seen is that people do a big grid search, but random search only for a certain amount of time.
Thank you! That visualisation with explaining sample mean being closer made A LOT OF SENSE! Though I do not understand the degrees of freedom part and it always bothered me! Could you make a video on degrees of freedom as well (I can see how we get x3 from the other info but I do not get how it helps anything and how it is related to dividing 2?? Thanks a lot in advance!)
great video, thx!
thank you for the explantion!
great job, finally useful video to make this theorem simple and clear , keep working
Thanks! Will do! :)
But how do you get Q? How do you get K? How are the values of those vectors defined?
So in poor words. Oscillation in time domain gets converted into a sum of rotational frequencies in complex domain?
Exactly
Fascinating stuff cheers mate
Thanks! Glad you liked it! :)
Video errors: - at 03:36 I should have written "low variance" on the screen for underfitting instead of "high variance". Thanks @nuktu for noticing this!
Full video at: ruclips.net/video/B9rhzg6_LLw/видео.html
Great explanation! Thankyou so much!
Thanks! Glad it was helpful! Make sure to check the other videos in the series if you wanna learn more about object detection. :)
dada tank u
Where did you get the original explanation for this?
There are more places which explains this in a similar manner. For instance, I read this blog a while ago: explained.ai/regularization/
Why are the circles getting bigger as you minimise the loss?
I think if you consider the concentric circles as a top down view of a hill, it topological view. Gradient descent would involve going down the hill from the top (the smallest circles) down the slope (to larger and larger circles). Not sure if this is accurate, but this is my sense of what he was describing in the video.
@@kippie608 That's exactly what I had in mind. Now that I think about it, it might be a bit confusing as people tend to depict gradient descent the other way around.
Great explanation!
Glad it was helpful! :)
Learn more about the differences between L1 and L2 regularization here: ruclips.net/video/aBgMRXSqd04/видео.html
Question, if in 2:15 the feature map passes through a CNN of 3X3 , then h and w in the minute 3:05 wouldn't be of the height and width of the feature map less 2 ?(3-1 both due to the fact that is 3X3 the CNN) or may be a padding of 1 should be applied first to the feature map ?
This is probably the best explanation of this concept on RUclips. Every other video on standard deviation glosses over why we use Bessel’s correction.
Thank you! Happy to hear that! :)
Can you elaborate more on how the degrees of freedom are connected to the bias? Why is it that we need to consider degrees of freedom in the sample metrics but not in the population metrics?
Sometimes the market needs a good laugh, and $LLM delivers. But jokes aside, it’s launching on SOLANA and hitting BingX tomorrow. I’ll admit it-I’m curious to see where this goes.
recently i discovered this channel and i have to say your explanations are so simple its very good to understand and i want to thank you
Omg, thank you for the explanation! I was eagerly searching for this one ❤
Thanks! Happy to hear this! :)
Arey bhai aur thode khatin words use kar le na simple chiz ko samjhane ke liye
😂
Excellent
Thanks :)
Lovely video. Great explanation!!!🎉🎉
Thanks! Glad you liked it! :)
Why do I get a different mean from your sample of [173,173,172,161] in your example at 2:00? I get a mean of 169.75 not 170.38.
nice video dude clearly explaining the concept, thx
Happy to hear that! :)
-1 is not going upward, to the previous hidden state, but to the right, toward the point wise multiplication of zt and h(tilde)t
At 03:36 you say "low variance" for underfitting models, but the screen says "high variance" on the right hand side box. I assume that should say "low variance" too?
Yes, I should have written "low variance" on the screen. Underfitting has high bias, and a low variance in general. Sorry for the confusion and thanks for noticing this! I will ad a pin to this video where I mention this error so others don't get confused.
Full video link: ruclips.net/video/8ZYLWipgPT0/видео.html
One question, for the total gradient for we need to calculate for o1,o2 too and add them together right?
A more theoretical overview of these issues are presented in my video about the bias-variance trade-off: ruclips.net/video/5mbX6ITznHk/видео.html
Must Love them Hungarians!
Thank you so much for explaining this topic in such an easy way.
preparing for a job interview and im handling object detection, this is definitely a must for everyone interested in learning more about this topic.
So, BART was made “outdated” by which new technology? What is the best tool for NLP tasks?
Thanks for the clear explanation!
how we get shared key? bro
This was a really good explanation of the Fourier Teansform.
Correction -- the Gaussian distribution is NOT on the *perpendicular distance* of the input-label tuple from the mean line, but instead the *vertical distance* (along the label dimension).
This is a very nice crisp content. Thank you.
Thanks! Glad you liked it! :)
The best ML channel
Amazing content my friend, keep going !
Thanks! Glad you liked it! :)
Waaaejtg5f e rf
Man, it's literally the first time I ever comment a video on Yt. I don't have much time to prepare for the exam, but your delievery and explanations are so clear. Be blessed and keep on going!!
Many thanks! Make sure to also check the other videos as well! :)
Thanks for this! very useful for revision when preparing for a test or interview.
Glad it was helpful! :)
You didn't explain anything. What is the intuition behind q,k,v ? Just rewrote the formula
I kinda agree with this. It's an old video of mine and it's not that polished. Sorry you didn't find in it what you were looking for.