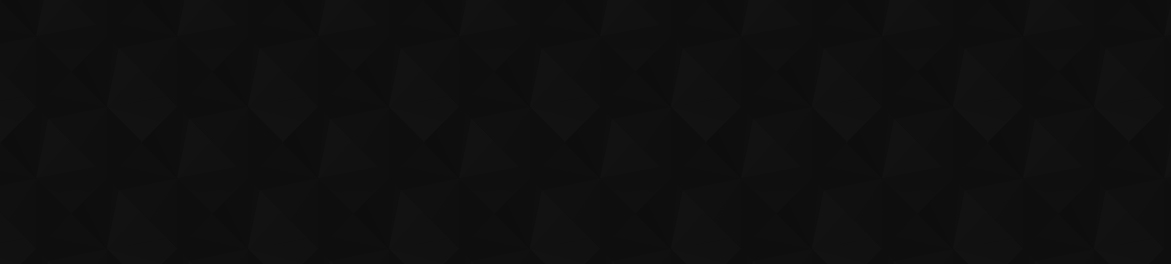
- Видео 56
- Просмотров 23 114
AI for health
США
Добавлен 1 янв 2021
Hello everyone,
This is Milky, Danica, and Jimeng. We provide short videos teaching artificial intelligence (AI) for healthcare, including machine learning (ML), deep learning (DL), and their applications to healthcare data.
And we also provide short cat videos, a scientifically proven method for helping to learn difficult subjects like AI and ML.
About:
Danica works for the health data science industry as a VP for AI at GE Healthcare. sites.google.com/view/danicaxiao/home
Jimeng is a computer science professor at the Univ of Illinois, Urbana-Champaign.
sunlab.org/
They both research AI for healthcare.
Milky is the most curious and energetic cat in the world. She likes to eat, jump, and play fetch.
This is Milky, Danica, and Jimeng. We provide short videos teaching artificial intelligence (AI) for healthcare, including machine learning (ML), deep learning (DL), and their applications to healthcare data.
And we also provide short cat videos, a scientifically proven method for helping to learn difficult subjects like AI and ML.
About:
Danica works for the health data science industry as a VP for AI at GE Healthcare. sites.google.com/view/danicaxiao/home
Jimeng is a computer science professor at the Univ of Illinois, Urbana-Champaign.
sunlab.org/
They both research AI for healthcare.
Milky is the most curious and energetic cat in the world. She likes to eat, jump, and play fetch.
Видео
Baking Responsibility into the AI for Health Lifecycle
Просмотров 62Месяц назад
Abstract: AI, and particularly machine learning, is reshaping the way we think about scientific research and healthcare. And yet, the collection and use of patient data, its subsequent conversion into foundation and predication models, and their application in biomedical research and healthcare raises many ethical questions and societal quandaries that have the potential to thwart such activiti...
Pranav Rajpurkar, Harvard University, The Generalist Medical AI Will See You Now,
Просмотров 296Месяц назад
Title: The Generalist Medical AI Will See You Now Abstract: Accurate interpretation of medical images is crucial for disease diagnosis and treatment, and AI has the potential to minimize errors, reduce delays, and improve accessibility. The focal point of this presentation lies in a grand ambition: the development of 'Generalist Medical AI' systems that can closely resemble doctors in their abi...
Multimodal Generative AI for Precision Health
Просмотров 227Месяц назад
Title: Multimodal Generative AI for Precision Health Abstract: The dream of precision health is to develop a data-driven, continuous learning system where new health information is instantly incorporated to optimize care delivery and accelerate biomedical discovery. The confluence of technological advances and social policies has led to rapid digitization of multimodal, longitudinal patient jou...
AI and the healthcare data logistics problem by Vera Mucaj
Просмотров 64Месяц назад
Title: AI and the healthcare data logistics problem Abstract: Healthcare decisions depend heavily on comprehensive, accessible data. Without these, patient outcomes may suffer. However, healthcare data is often fragmented across multiple silos, limiting its true utility. In this lecture, we will discuss healthcare data fragmentation, and how overcoming it can lead to better datasets that can be...
Unleashing the power of Medical Imaging Foundation Models
Просмотров 125Месяц назад
Title: Unleashing the power of Medical Imaging Foundation Models Description: This session will explore cutting-edge AI and machine learning advancements that are set to transform workflow across technologists and clinicians. Discover how the power of recent advances in AI/ML and generative AI can optimize processes and revolutionize healthcare. Bio: Parminder Bhatia (Chief AI Officer - GE Heal...
Accelerate clinical trials with AI - ICML'24 presentation
Просмотров 1633 месяца назад
Accelerate clinical trials with AI - ICML'24 presentation
Panacea - finetune a small LLM for clinical trial tasks
Просмотров 443 месяца назад
Panacea - finetune a small LLM for clinical trial tasks
Automated systematic reviews with TrialMind
Просмотров 3005 месяцев назад
Automated systematic reviews with TrialMind
TrialMind data science assistant for clinical trial projects
Просмотров 1115 месяцев назад
TrialMind data science assistant for clinical trial projects
Keiji AI's TrialMind demo for trial eligibility criteria drafting and feasibility analysis
Просмотров 2977 месяцев назад
Effective clinical trial design is crucial to the success of any clinical trial. In this demonstration, we showcase how Keiji AI's TrialMind agents can assist clinical trial designers in creating eligibility criteria for trials and conducting feasibility analysis against a real-world patient database through an interactive interface and natural language queries. Check out more about Keiji AI's ...
Automated cancer Survivorship Care Plan (SCP) generation with large language models
Просмотров 739 месяцев назад
Automated cancer Survivorship Care Plan (SCP) generation with large language models
Medisyn demo - Clinical predictive modeling with synthetic data augmentation
Просмотров 84Год назад
To build an accurate clinical predictive model, diverse and high-quality training data is crucial. However, in the real world, patient data is often limited and constrained for use in training. Medisyn offers a solution by augmenting the limited patient data with diverse and vast synthetic patient data. This approach improves model accuracy, as demonstrated in a case study on a mortality predic...
(Q&A) AI Maturity - how to maximize the impact of AI via a disciplined approach
Просмотров 63Год назад
Lecture Time: Mar 29, 2023 Q&A sessions for "AI Maturity: how to maximize the impact of AI via a disciplined approach". Watch the actual presentation in the last video. Content: 00:00 - Diff between AI solutions and products 04:41 - Diff between AI products and softwares 11:38 - How to sell AI solution to external orgs 22:30 - How to implement models following AI standards in healthcare systems...
AI Maturity: how to maximize the impact of AI via a disciplined approach
Просмотров 64Год назад
Lecture Time: Mar 29, 2023 Title: AI Maturity - how to maximize the impact of AI via a disciplined approach Bio: Dr. Sipes is a Chief Data Scientist at UnitedHealthcare, and avid Advocate of modern Machine Learning approaches, products, and technologies, and a big proponent of moving companies forward on their AI Maturity journey. She has over twenty years of ML leadership and innovation experi...
Computer Science for Neurodegenerative disorders
Просмотров 192Год назад
Computer Science for Neurodegenerative disorders
Using ML to Make Cell Therapy Safer & More Accessible
Просмотров 100Год назад
Using ML to Make Cell Therapy Safer & More Accessible
PyHealth Live - 09 Support Medical Imaging Data
Просмотров 225Год назад
PyHealth Live - 09 Support Medical Imaging Data
(Q&A) MLOps - Sustaining Data Science in Production
Просмотров 34Год назад
(Q&A) MLOps - Sustaining Data Science in Production
MLOps - Sustaining Data Science in Production
Просмотров 80Год назад
MLOps - Sustaining Data Science in Production
PyHealth Live - 08 (Part II) Demo: Build Biosignal Pipeline in PyHealth
Просмотров 130Год назад
PyHealth Live - 08 (Part II) Demo: Build Biosignal Pipeline in PyHealth
PyHealth Live - 08 (Part I) Support Biosignals Inputs (e.g., EEG, ECG) with the Same APIs
Просмотров 93Год назад
PyHealth Live - 08 (Part I) Support Biosignals Inputs (e.g., EEG, ECG) with the Same APIs
PyHealth Live - 07 Adopt Customized Model into Pyhealth
Просмотров 143Год назад
PyHealth Live - 07 Adopt Customized Model into Pyhealth
PyHealth Live - 06 Use Custom Dataset with PyHealth
Просмотров 250Год назад
PyHealth Live - 06 Use Custom Dataset with PyHealth
PyHealth Live - 05 (Part II) Step-by-Step Live Demo on Building ML Pipeline
Просмотров 371Год назад
PyHealth Live - 05 (Part II) Step-by-Step Live Demo on Building ML Pipeline
PyHealth Live - 05 (Part I) Use pyhealth to Build a Healthcare ML Pipeline
Просмотров 163Год назад
PyHealth Live - 05 (Part I) Use pyhealth to Build a Healthcare ML Pipeline
PyHealth Live - 04 (Part II) Tokenizers for Medical ML Applications
Просмотров 178Год назад
PyHealth Live - 04 (Part II) Tokenizers for Medical ML Applications
PyHealth Live - 04 (Part I) Medical Code Mapping and Lookup in PyHealth
Просмотров 271Год назад
PyHealth Live - 04 (Part I) Medical Code Mapping and Lookup in PyHealth
PyHealth Live - 03 (Part III) PyHealth's Metrics
Просмотров 151Год назад
PyHealth Live - 03 (Part III) PyHealth's Metrics
PyHealth Live - 03 (Part II) PyHealth's Trainer
Просмотров 171Год назад
PyHealth Live - 03 (Part II) PyHealth's Trainer
Can you share the code
Nice content you're really doing great
This is amazing!
You should spend more time on explaining the model itself because that's what is the most interesting. You just showed a picture without any explanation.
could you share the slides please?
This could be the dumbest question you get, but how does someone take a trained model after using PyHealth and turn it into something like an API to call and get predictions from?
thanks!
Hi Thank you for sharing this! I wonder if it’s worked for MIMIC-IV. Thank you!
'PromoSM' 💥
Nice work, where can I get access to these slides
This is awesome! Thank you guys
Content: 00:00 - Intro 00:54 - PyHealth Overview 03:25 - Live Session Schedule 04:41 - 5-stage Pipeline 05:58 - Useful Resources
Outline: 00:00 - Intro 01:09 - Customized SampleDataset Example 05:14 - Summary of the Live 07:21 - Useful resources
Outline: 00:00 - Intro (slides) 00:22 - Intro (Colab) 01:24 - Usecase example 02:20 - Task example
Outline: 00:00 - Overview of this Live (slides) 02:00 - Instructions for pyhealth.datasets (Colab) 04:46 - Examples to process MIMIC-III 06:27 - Query information after processing 09:11 - Examples to process OMOP-CDM datasets
Outline: 00:00 - Intro 01:40 - Data structure (slides) 04:18 - Event structure 05:40 - Visit structure 08:08 - Patient structure
Outline: ------------------------------ 00:00 - Introduction 03:58 - Overview of PyHealth 09:12 - Key designs in PyHealth 13:24 - PyHealth installation 13:48 - 5-stage ML pipeline 15:20 - Example 1: Relieve the pain of data processing 20:04 - Example 2: Build the model for you 23:13 - Example 3: Master the medical coding systems 26:57 - Summary of the PyHealth 28:04 - Development Team and Acknowledgements 28:36 - PyHealth resources and call for contributions
Terrific interpretation. JT-VAE has been so popular that you would like to come across this approach in every summary paper regarding molecule generation. For the first time I almost get the essence behind it.
Can you explain how can you find dimension-wise probability?
excellent and important work; typical of your and your team's work and contributions. We are trying to adopt your work to predict adverse events in cancer patients. We downloaded your code and it works fine using the mimic3 dataset as input. We have successfully run the trained model and the test one with no errors.
Thanks Nabil! Hope everything well with you and your team.
Man, such awesome video, sooo under-rated
Please share with me your email i need your advise
Thank you brother
what is there in the embedding module and transforming module, and where did you get the data for interaction module ?
i am also curious about those, better start reading the paper :)
🤤 Promo-SM!!
Hello Professor, Is this book required for the CSE6250 for OMSA in Georgia Tech? I am planning to take the course in Spring2022 semester and was thinking to get prepared for the course.
It is relevant for the deep learning part of the course but not spark and hadoop part.
Dear professor, Great piece of work. Curious to know how handy it is in terms of looking into relevant jupyter notebooks or repository for hands on case studies. Thank you.
I'm glad you ask that, the book is more about the concepts, but very soon I will release a set of coursera MOOCs on DL for healthcare, which will be more hands-on and we do have a new project to create codebooks for these case studies, but that is just getting started.
@@AIforhealth Atleast would love some snippets of it on this channel soon. Thank you and awaiting!
@@AIforhealth Will be awaiting the course as well. Thank you!
It's a very interesting work with high potentials. However, its implementation code is poorly written with many bugs. We have managed to resolve some of the bugs but not all. Unfortunately, the first author is not responding to our emails. It'd be great if you can help us reach the first author, we only wish to test in our hospital setting. Thanks.
Feel free to copy me on those emails. Love to help.
Paper: arxiv.org/abs/1911.10395
Thanks for the explaining
No problem!
Did anyone implemented this paper ? If so please send the google Colab link. Thank U.
It is just using RNN (GRU) model, so you should be able to find that model in any deep learning package. You can also check out github.com/yzhao062/PyHealth which has more advanced/specific deep learning models for health data.
Hello Jimeng, This is Soumava Dey, a student of your DLH course, just subscribed to your channel. Videos look very good and informative. I do have my own RUclips channel on Data Science and I will definitely share your RUclips channel link on my platform for better reach.
nice quick review of RNN (recurrent neural networks) @ 1:18 & GRU (gated recurrent unit) @ 5:55 Doctor AI paper coverage proper starts @ 11:12
Milky makes a cameo at the beginning
Thank you professor Jim Sun. I did you Big Data For Health and one of best course in GT. Thank you for sharing.
Related papers: sleep.csail.mit.edu/files/rfsleep-paper.pdf www.ijcai.org/Proceedings/2019/0817.pdf
This is the related video about STAN paper ruclips.net/video/t6BAAzk6ZK0/видео.html&ab_channel=AIforhealth
Here is the paper. academic.oup.com/jamia/advance-article/doi/10.1093/jamia/ocaa322/6118380
SkipGNN code github.com/kexinhuang12345/SkipGNN
SkipGNN paper www.nature.com/articles/s41598-020-77766-9
MolTrans code link github.com/kexinhuang12345/MolTrans
MolTrans paper link academic.oup.com/bioinformatics/advance-article/doi/10.1093/bioinformatics/btaa880/5929692
Thanks for this!
I am glad this is helpful
hi