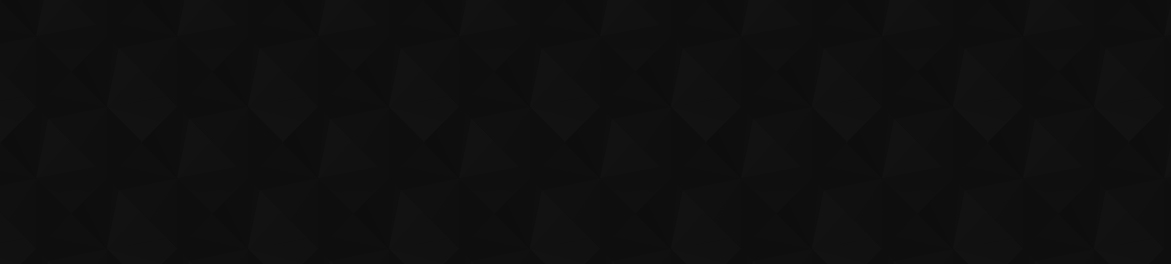
- Видео 8
- Просмотров 40 291
Bioimage Informatics Facility
Швеция
Добавлен 16 май 2020
The BioImage Informatics Facility (SciLifeLab, NMI) provides the support and training you need to perform state-of-the-art analyses on your image data. Our experts can help you deploy computational methods using computer vision, machine learning, and bioinformatics to analyze your images. Whether you are looking for training on how to use existing software solutions or help developing a custom workflow, we can help you get your analysis running.
Next-generation bioimage file formats in spatial biology
Speaker: Jonas Windhager, BIIF
Seminar series of the Centre for Image Analysis (CBA)
www.it.uu.se/about_us/divisions/vi3/seminars-list/image_analysis
Methods for biological imaging and in situ profiling of RNA and/or protein expression in biological tissue are rapidly evolving, pushing the limits of existing computational analysis infrastructure. To cope with increased computational demands, novel approaches for storing, accessing and sharing bioimaging data are currently emerging. In this practically-minded seminar, I discuss ongoing community efforts in standardizing the next generation of bioimage file formats, facilitating the integration of bioimaging data with spatially resolved (e.g.,...
Seminar series of the Centre for Image Analysis (CBA)
www.it.uu.se/about_us/divisions/vi3/seminars-list/image_analysis
Methods for biological imaging and in situ profiling of RNA and/or protein expression in biological tissue are rapidly evolving, pushing the limits of existing computational analysis infrastructure. To cope with increased computational demands, novel approaches for storing, accessing and sharing bioimaging data are currently emerging. In this practically-minded seminar, I discuss ongoing community efforts in standardizing the next generation of bioimage file formats, facilitating the integration of bioimaging data with spatially resolved (e.g.,...
Просмотров: 95
Видео
Introduction to the steinbock toolkit for multiplexed tissue image processing
Просмотров 1,5 тыс.Год назад
In this hands-on webinar we showcase steinbock, a computational toolkit for batch-processing multiplexed tissue images using established tools and approaches. The toolkit can be used to rapidly extract spatially resolved single-cell data for downstream statistical analysis, interfacing with popular data analysis environments such as Python or R/Bioconductor. Further information and material can...
TissUUmaps 3.1: Interactive visualization and quality assessment of large-scale spatial omics
Просмотров 866Год назад
We present TissUUmaps, browser-based tool for GPU-accelerated visualization and interactive exploration of tens of millions of datapoints overlaying tissue samples. Users can visualize markers and regions, explore spatial statistics and quantitative analyses of tissue morphology, and assess the quality of decoding in situ transcriptomics data. TissUUmaps provides instant multi-resolution image ...
TissUUmaps 3.0 Introduction
Просмотров 4472 года назад
We present TissUUmaps 3.0, a browser-based tool for GPU-accelerated visualization and interactive exploration of millions of datapoints overlaying tissue samples. Users can visualize markers and regions, explore spatial statistics and quantitative analyses of tissue morphology, and assess the quality of decoding in situ transcriptomics data. TissUUmaps provides instant multi-resolution image vi...
Introduction to QuPath for Multiplexed Fluorescence Microscopy
Просмотров 21 тыс.2 года назад
00:51 Data set 01:50 Creating a QuPath project, importing and inspecting a data set 06:42 Cell detection 11:15 Cell measurements 13:12 Result tables - Annotation and detection measurements 14:54 Cell classification using a single measurement classifier 20:31 Inspection of classified cells 23:32 Outlook: training a cell classifier 24:03 Set a simple pixel threshold to create annotations 30:47 Ou...
Pixel Classification using Weka in Fiji
Просмотров 2,1 тыс.4 года назад
Demonstration of the Trainable Weka Segmentation" plugin in Fiji. Online Documentation: imagej.net/Trainable_Weka_Segmentation References: Arganda-Carreras, I. et al. Trainable Weka Segmentation: a machine learning tool for microscopy pixel classification. Bioinformatics 33, 2424-2426 (2017) Schindelin, J.; Arganda-Carreras, I. & Frise, E. et al. (2012), "Fiji: an open-source platform for biolo...
Pixel Classification using Ilastik
Просмотров 2,3 тыс.4 года назад
Demonstration of the basics of pixel classification using Ilastik. Online Documentation: www.ilastik.org/documentation/ Cite it when you use it: Berg, S. et al. ilastik: interactive machine learning for (bio)image analysis. Nature Methods 1-7 (2019) Data source: ISBI Challenge, brainiac2.mit.edu/isbi_challenge Presenter: A. Klemm, BioImage Informatics Facility, SciLifeLab
CellProfiler Introduction
Просмотров 12 тыс.4 года назад
A first introduction into BioImage Analysis using CellProfiler. Material: github.com/ahklemm/CellProfiler_Introduction 4:48 Cellprofiler interface and first steps 15:51 Getting outlines of the nuclei: IdentifyPrimaryObjects 22:20 IdentifyPrimaryObjects: Which steps would you need to do in Fiji? 24:08 IdentifyPrimaryObjects: Advanced settings 35:48 IdentifyPrimaryObjects: Final settings 37:20 Ge...
Hello, thank you for your video. I wondered why my image's background is not 0, but up to 1000.
Are there some more advanced AI driven tools that surpass Cellprofiler on tasks like cell classification with images?
Thanks for the video. I can't find a link to the workflow via QuPath documentation you mention in the beginning of the video. Could you please share this?
very good explanation you save my life much easier
Hi! Thanks for this detailed and precise description! This is very helpful. I had a question: I am following the steps you described ( analyzing a 16-bit, 3-channel fluorescent IHC image), but my background shows high pixel values, too ( for example, I'm not seeing 0 for dapi where there's no dapi). Should I do a background correction?
This is a good video. Too bad there are so many commercials that I can barely watch it. Every time I pause to try to repeat it in QuPath I need to watch 2 more commercials. It makes it pretty hard to learn like this 😞
Hello, if you still have trouble watching youtube with too many commercials, search for an applicable adblocker for your browser!
Hi, what can I do if multiple nuclei are detected as one?
Thank you very much for this great video! for me the single measurement classifier doesn´t detect positive cells even if I play with threshold. Do you have any ideas about this? that would be of a great help
A good trick is to move the threshold completely to the left to see if cell detections light up in the right color. Make sure that you have the detections visible and that the color of the cell class is different from the color of unclassified cell detections (red by default). If you still have problems please post the problem on forum.image.sc for a continued discussion.
What image file types did you import here? Were they already spectrally unmixed with Inform software prior to analyzing with Qupath?
Yes, it is the spectrally unmixed data sets exported by the Inform software.
pls i want soft ware
Is it possible, or practical, to do multi-class learning and classification? I have tried this before, but I couldn't get it to work. This is what keeps me using Inform, which after years of no development, is a slow and unreliable piece of software.
QuPath can use ML for classification of classes - see here github.com/BIIFSweden/SpatialWorkshop/blob/main/part1_qupath/README.md#cell-classification or on the QuPath documentation.
Thank you for the content, it really helped.
Thanks a lot for this video.
Thank you, Anna! This video saved me hours in front of imageJ. Very nicely explained and easy to understand and to follow. I am so happy I found this video since I exactly needed this quantification (ratio cytoplasm:nucleus) for my last figure in the manuscript! Thank you! Greetings to Sweden, Andrea (wet lab scientist at University of Bern)
Hi Andrea, happy to hear that it was helpful!
excellent voice quality and pronunciation