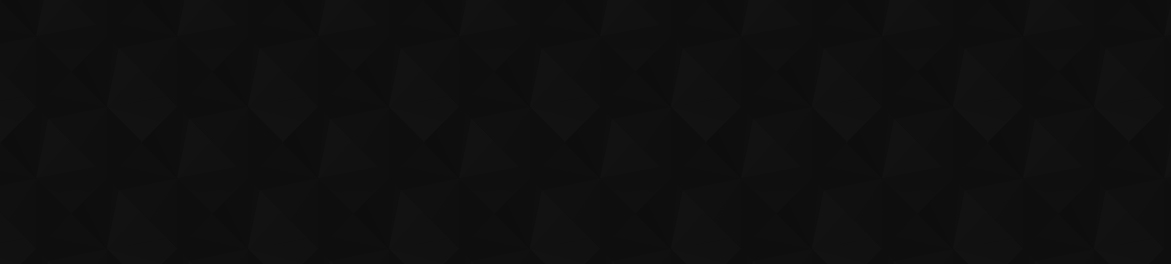
- Видео 100
- Просмотров 295 331
Volodymyr Kuleshov
Добавлен 13 май 2007
Machine Learning and Artificial Intelligence.
Cornell CS 6785: Deep Generative Models. Lecture 1: Course Introduction
Cornell CS 6785: Deep Generative Models. Lecture 1: Course Introduction
Presented by Prof. Kuleshov from Cornell University | Curated & Edited by Michael Ahedor
Instructor: www.cs.cornell.edu/~kuleshov/
Course Website: kuleshov-group.github.io/dgm-website/
Follow us on Twitter/X here: volokuleshov
Presented by Prof. Kuleshov from Cornell University | Curated & Edited by Michael Ahedor
Instructor: www.cs.cornell.edu/~kuleshov/
Course Website: kuleshov-group.github.io/dgm-website/
Follow us on Twitter/X here: volokuleshov
Просмотров: 11 819
Видео
Cornell CS 6785: Deep Generative Models. Lecture 17: Probabilistic Reasoning
Просмотров 1,3 тыс.9 месяцев назад
Cornell CS 6785: Deep Generative Models. Lecture 17: Probabilistic Reasoning Presented by Prof. Kuleshov from Cornell University | Curated & Edited by Michael Ahedor Instructor: www.cs.cornell.edu/~kuleshov/ Course Website: kuleshov-group.github.io/dgm-website/ Follow us on Twitter/X here: volokuleshov 00:00 Intro 01:40 Lecture 1:10:46 Summary
Cornell CS 6785: Deep Generative Models. Lecture 16: Discrete Deep Generative Models
Просмотров 1 тыс.9 месяцев назад
Cornell CS 6785: Deep Generative Models. Lecture 16: Discrete Deep Generative Models Presented by Prof. Kuleshov from Cornell University | Curated & Edited by Michael Ahedor Instructor: www.cs.cornell.edu/~kuleshov/ Course Website: kuleshov-group.github.io/dgm-website/ Follow us on Twitter/X here: volokuleshov 00:00 Intro 02:45 Lecture 1:11:13 Summary
Cornell CS 6785: Deep Generative Models. Lecture 15: Combining Generative Model Families
Просмотров 9309 месяцев назад
Cornell CS 6785: Deep Generative Models. Lecture 15: Combining Generative Model Families Presented by Prof. Kuleshov from Cornell University | Curated & Edited by Michael Ahedor Instructor: www.cs.cornell.edu/~kuleshov/ Course Website: kuleshov-group.github.io/dgm-website/ Follow us on Twitter/X here: volokuleshov 00:00 Intro 04:07 Lecture 1:07:57 Summary
Cornell CS 6785: Deep Generative Models. Lecture 14: Evaluating Generative Models
Просмотров 1,1 тыс.9 месяцев назад
Cornell CS 6785: Deep Generative Models. Lecture 14: Evaluating Generative Models Presented by Prof. Kuleshov from Cornell University | Curated & Edited by Michael Ahedor Instructor: www.cs.cornell.edu/~kuleshov/ Course Website: kuleshov-group.github.io/dgm-website/ Follow us on Twitter/X here: volokuleshov 00:00 Intro 06:11 Lecture 1:09:29 Summary
Cornell CS 6785: Deep Generative Models. Lecture 13: Diffusion Models
Просмотров 2,5 тыс.9 месяцев назад
Cornell CS 6785: Deep Generative Models. Lecture 13: Diffusion Models Presented by Prof. Kuleshov from Cornell University | Curated & Edited by Michael Ahedor Instructor: www.cs.cornell.edu/~kuleshov/ Course Website: kuleshov-group.github.io/dgm-website/ Follow us on Twitter/X here: volokuleshov 00:00 Intro 14:03 Lecture 1:11:05 Summary
Cornell CS 6785: Deep Generative Models. Lecture 12: Score-Based Generative Models
Просмотров 2,2 тыс.9 месяцев назад
Cornell CS 6785: Deep Generative Models. Lecture 12: Score-Based Generative Models Presented by Prof. Kuleshov from Cornell University | Curated & Edited by Michael Ahedor Instructor: www.cs.cornell.edu/~kuleshov/ Course Website: kuleshov-group.github.io/dgm-website/ Follow us on Twitter/X here: volokuleshov 00:00 Intro 14:08 Lecture 1:15:32 Summary
Cornell CS 6785: Deep Generative Models. Lecture 11: Energy-Based Models
Просмотров 2,3 тыс.10 месяцев назад
Cornell CS 6785: Deep Generative Models. Lecture 11: Energy-Based Models Presented by Prof. Kuleshov from Cornell University | Curated & Edited by Michael Ahedor Instructor: www.cs.cornell.edu/~kuleshov/ Course Website: kuleshov-group.github.io/dgm-website/ Follow us on Twitter/X here: volokuleshov 00:00 Intro 03:38 Lecture 1:10:31 Summary
Cornell CS 6785: Deep Generative Models. Lecture 10: Advanced Topics in GANs
Просмотров 1,2 тыс.10 месяцев назад
Cornell CS 6785: Deep Generative Models. Lecture 10: Advanced Topics in Generative Adversarial Networks Presented by Prof. Kuleshov from Cornell University | Curated & Edited by Michael Ahedor Instructor: www.cs.cornell.edu/~kuleshov/ Course Website: kuleshov-group.github.io/dgm-website/ Follow us on Twitter/X here: volokuleshov 00:00 Intro 21:24 Lecture 1:04:00 Summary
Cornell CS 6785: Deep Generative Models. Lecture 9: Generative Adversarial Networks
Просмотров 1,4 тыс.10 месяцев назад
Cornell CS 6785: Deep Generative Models. Lecture 9: Generative Adversarial Networks Presented by Prof. Kuleshov from Cornell University | Curated & Edited by Michael Ahedor Instructor: www.cs.cornell.edu/~kuleshov/ Course Website: kuleshov-group.github.io/dgm-website/ Follow us on Twitter/X here: volokuleshov 00:00 Intro 08:51 Lecture 1:07:13 Summary
Cornell CS 6785: Deep Generative Models. Lecture 8: Advanced Flow Models
Просмотров 1,5 тыс.10 месяцев назад
Cornell CS 6785: Deep Generative Models. Lecture 8: Advanced Flow Models Presented by Prof. Kuleshov from Cornell University | Curated & Edited by Michael Ahedor Instructor: www.cs.cornell.edu/~kuleshov/ Course Website: kuleshov-group.github.io/dgm-website/ Follow us on Twitter/X here: volokuleshov 00:00 Intro 17:00 Lecture 1:07:32 Summary
Cornell CS 6785: Deep Generative Models. Lecture 7: Normalizing Flows
Просмотров 2 тыс.10 месяцев назад
Cornell CS 6785: Deep Generative Models. Lecture 7: Normalizing Flows Presented by Prof. Kuleshov from Cornell University | Curated & Edited by Michael Ahedor Instructor: www.cs.cornell.edu/~kuleshov/ Course Website: kuleshov-group.github.io/dgm-website/ Follow us on Twitter/X here: volokuleshov 00:00 Intro 08:27 Lecture 57:52 Summary
Cornell CS 6785: Deep Generative Models. Lecture 6: Learning Latent Variable Models
Просмотров 2,2 тыс.11 месяцев назад
Cornell CS 6785: Deep Generative Models. Lecture 6: Learning Latent Variable Models Presented by Prof. Kuleshov from Cornell University | Curated & Edited by Michael Ahedor Instructor: www.cs.cornell.edu/~kuleshov/ Course Website: kuleshov-group.github.io/dgm-website/ Follow us on Twitter/X here: volokuleshov
Cornell CS 6785: Deep Generative Models. Lecture 5: Latent Variable Models
Просмотров 2,6 тыс.11 месяцев назад
Cornell CS 6785: Deep Generative Models. Lecture 5: Latent Variable Models Presented by Prof. Kuleshov from Cornell University | Curated & Edited by Michael Ahedor Instructor: www.cs.cornell.edu/~kuleshov/ Course Website: kuleshov-group.github.io/dgm-website/ Follow us on Twitter/X here: volokuleshov
Cornell CS 6785: Deep Generative Models. Lecture 4: Maximum Likelihood Learning
Просмотров 2,9 тыс.11 месяцев назад
Cornell CS 6785: Deep Generative Models. Lecture 4: Maximum Likelihood Learning Presented by Prof. Kuleshov from Cornell University | Curated & Edited by Michael Ahedor Instructor: www.cs.cornell.edu/~kuleshov/ Course Website: kuleshov-group.github.io/dgm-website/ Follow us on Twitter/X here: volokuleshov
Cornell CS 6785: Deep Generative Models. Lecture 3: Autoregressive Models
Просмотров 4,1 тыс.11 месяцев назад
Cornell CS 6785: Deep Generative Models. Lecture 3: Autoregressive Models
Cornell CS 6785: Deep Generative Models. Lecture 2: Introduction to Probabilistic Modeling
Просмотров 6 тыс.11 месяцев назад
Cornell CS 6785: Deep Generative Models. Lecture 2: Introduction to Probabilistic Modeling
Cornell CS 6785: Deep Generative Models. Lecture 1: Course Introduction
Просмотров 3,7 тыс.11 месяцев назад
Cornell CS 6785: Deep Generative Models. Lecture 1: Course Introduction
Cornell CS 5787: Applied Machine Learning. Lecture 1. Part 1: Introduction to Machine Learning
Просмотров 56 тыс.3 года назад
Cornell CS 5787: Applied Machine Learning. Lecture 1. Part 1: Introduction to Machine Learning
Cornell CS 5787: Applied Machine Learning. Lecture 1. Part 4: Logistics and Other Information
Просмотров 8 тыс.3 года назад
Cornell CS 5787: Applied Machine Learning. Lecture 1. Part 4: Logistics and Other Information
Cornell CS 5787: Applied Machine Learning. Lecture 2 - Part 1: A Supervised Machine Learning Problem
Просмотров 11 тыс.3 года назад
Cornell CS 5787: Applied Machine Learning. Lecture 2 - Part 1: A Supervised Machine Learning Problem
Applied Machine Learning. Lecture 2 - Part 2: Anatomy of Supervised Machine Learning: The Dataset
Просмотров 8 тыс.3 года назад
Applied Machine Learning. Lecture 2 - Part 2: Anatomy of Supervised Machine Learning: The Dataset
Cornell CS 5787: Applied Machine Learning. Lecture 1. Part 3: About the Course
Просмотров 10 тыс.3 года назад
Cornell CS 5787: Applied Machine Learning. Lecture 1. Part 3: About the Course
Cornell CS 5787: Applied Machine Learning. Lecture 21. Part 2: Bias / Variance Analysis
Просмотров 9523 года назад
Cornell CS 5787: Applied Machine Learning. Lecture 21. Part 2: Bias / Variance Analysis
Cornell CS 5787: Applied Machine Learning. Lecture 22. Part 1: Learning Curves
Просмотров 7 тыс.3 года назад
Cornell CS 5787: Applied Machine Learning. Lecture 22. Part 1: Learning Curves
Cornell CS 5787: Applied Machine Learning. Lecture 1. Part 2: Three Approaches to Machine Learning
Просмотров 17 тыс.3 года назад
Cornell CS 5787: Applied Machine Learning. Lecture 1. Part 2: Three Approaches to Machine Learning
Cornell CS 5787: Applied Machine Learning. Lecture 22. Part 2: Loss Curves
Просмотров 1,9 тыс.3 года назад
Cornell CS 5787: Applied Machine Learning. Lecture 22. Part 2: Loss Curves
Cornell CS 5787: Applied Machine Learning. Lecture 22. Part 4: Distribution Mismatch
Просмотров 1,6 тыс.3 года назад
Cornell CS 5787: Applied Machine Learning. Lecture 22. Part 4: Distribution Mismatch
Applied Machine Learning. Lecture 2. Part 3: Anatomy of Supervised Learning: Learning Algorithms
Просмотров 6 тыс.3 года назад
Applied Machine Learning. Lecture 2. Part 3: Anatomy of Supervised Learning: Learning Algorithms
Cornell CS 5787: Applied Machine Learning. Lecture 21. Part 1: Error Analysis
Просмотров 2,2 тыс.3 года назад
Cornell CS 5787: Applied Machine Learning. Lecture 21. Part 1: Error Analysis
Question on slide 12: As I understand it, we do know what the denoising process would be for an image. Just substract the noise that was added to generate the noisy version. But we want a NN to learn these parameters so that once it's trained, we can feed it random noise and it will output novel images. Is this statement correct?
thank you so much🤎🤎🤎
Great video!
@34:34 - The last integral on the RHS, why is there an S_theta(x) "square" term ? .. shudn't it just be s_theta(x) ??
It should be -y.x if y.f(x) < 1
On page 16 (around 38minutes): "The probability is non convex". I think the important thing is that the problem is non convex on Mu and sigma. It is true that P(x) is non-convex but this wouldn't be a problem if it was convex on mu/sigma while non convex on X. I got a little confused by the image that shows the mixture is non convex on the x axis and wanted to clarify if anyone also finds useful :) (and hope it makes sense)
the f-divergence part of the lecture is NOT very clear ... :(
great lecture but i wonder the slides look an awful lot like the standford cs236 lecture slides did you develope the course together 🙈
yes, we have been working on this course together for many years now: kuleshov-group.github.io/dgm-website/
What is meant by parameters? How do you calculate the number of parameters? After 25:56 it is mentioned number of parameters is 2^n-1
Well explained
H(1-\epsilon)^n isn't a probability as is unbounded. To make it a probability must be defined as min(1, H(1-\epsilon)^n).
Great lecture!
I havent seen the best course like that taking from basics, I love this !!
Where to find the assignments? They are absent in the website.
Hi professor, once Neural Autoregressive model(NADE) is trained or for that matter any model like wavenet or pixel CNN, How do i compute the probability of a new image belonging to learnt distribution?
Definitely one of the best courses on the topic !
Can you recommend any specific books that we can follow alongside this course ?
Excellent ! By far the Bestest lecture series on Generative Models simply because it explains the intuition behind all the underlying math .. just fabulous !!
Awesome !
Loving it !! ... Can't thank enough ..
Terrific Lectures ... just bang on the target .. addresses all the issues which are a bit tricky to understand .. Amazin !.. Thanks a ton
Excellent ! .. Thanks ! .. addresses almost all of the pain points pertaining to Probabilitistic Machine Learning
Turing out to be just amazing set of lectures .. exactly touching upon the most painful (to understand) points in "probabilistic machine learning" .. awesome !
thank you!
some feedback : very shallow , non concrete throw of words without deep explanation. for ex : 1) P(x|y) , no mention of what y is. 2) super resolution and signal processing examples : why is this not representation learning , model still learns a representaiton in these cases 3) imitation learning , again why is this not supervised learning (just because its RL) . Too much usage of jargon and no depth
it's just an intro bro relax...
Amazing lectures, thanks a lot! But I am getting a lot of adds on those lectures. For me its around 30 seconds of adds every 5 minutes. It makes it hard to stay locked in... especially on this lecture, which demands a bit more effort from me
Hey Volodymr -- Any chance that additional lectures will be added? I loved this first one
Hi, where can I get the slides of the course?
its only theory it would have been so much better if done practical side by side too.
One of the most amazing lectures. Ive never seen a lecture on generative models that is so connected like these, from simple autoregressive, to latent models, to gans, to energy-based, langevin dynamics, and finally to diffusion models, all are connected! The connectedness and story telling are so amazing! thank you Prof!
very high quality and amazing lectures, thank you Prof!
Prof could you please upload the slides since your github slides arent updated?
Bro these slides are exactly same as stanford cs236 slides. You can easily download them. He is teaching the same material.
Please use generative AI to super resolution the audio behind this video!
Why is pixel x3 dependent on x1, x2, but not x4..? Okay I get it it is using Chain rule in Probability to decompose the joint probability distribution.. Not saying x3 depends only on x1 and x2, but learni learning that marginal distribution as a way to predict joint distribution.
applied machine learning but i can only see ppts not practical implementation? how can this be applied?
I had been looking for CS236 videos and couldn't find any, then the next day I find this course that teaches from the same slides. Woohoo!!!
Thanks a lot. I really liked your teaching method. Some channels do not go into the details of the statistics but statistics is more important than just applying the method.
Great lecture, thanks!
Thank you for releasing this online! You're a legend
Thanks so much for fixing the audio! Looking forward the course!
why is the score function graph at around 21:00 postive after 5ish? As soon as you past the point corresponding to the mode at around 5 shouldn't it point left (be negative)? Same question for the other mode around -4
even i have the same doubt
Thanks for sharing such good materials! Does RNN belong to autoregressive models?
thank you, and yes they are: we have an example later on in this lecture
excellent lecture, horrible sound
Thanks for sharing these amazing lectures!
There are too much noise in audio. It will be great if you enhance the audio by using a generative model. Thanks for the video by the way...
agreed, lectures are awesome, but the sound is unfortunately captured poorly
Thanks for the lecture!
Hello, Thank you for providing this valuable course on generative modeling. I would like to ask a question about slide 18. Should the multiplication for alpha t hat be from alpha 1 to alpha t (small t) instead of alpha T (capital T)? Is my understanding correct?
Presenting the unified view of Energy, Score and Diffusion models is invaluable. My coursework didnt cover generative methods beyond VAE and GANs but this presentation has been a great surrogate. Thanks! A small correction to the proof on slide 14. I think there's possibly a rogue squared s_theta(x) in the third term.
Can you please share the slides? Thanks. It is an interesting and useful course!
Amazing Lectures! Can't wait to watch the next diffusion lecture! Awesome!
First comment !! I guess watching videos fully has its advantages