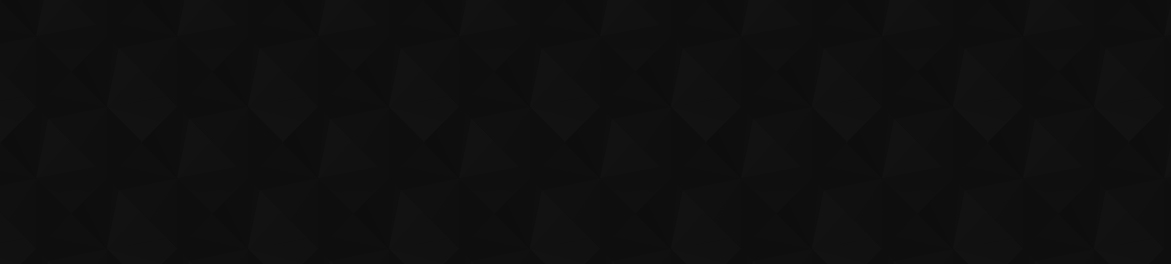
- Видео 12
- Просмотров 49 846
Juan MR Parrondo
Испания
Добавлен 17 ноя 2011
Seminars, lectures, and interesting videos on physics and science.
Juan MR Parrondo
Professor of Physics. Universidad Complutense de Madrid.
Juan MR Parrondo
Professor of Physics. Universidad Complutense de Madrid.
The additivity of entropy in the microcanonical ensemble
Here you have a basic discussion of the additivity of Boltzmann and microcanonical entropy. In the video, I explain the solution of exercise 3.5 of my lectures at Universidad Complutense.
Просмотров: 105
Видео
A simple derivation of the Black-Scholes equation
Просмотров 3,5 тыс.Год назад
At the end of the video there is an important comment that corrects a conceptual error in the derivation.
Two notes on foundations of statistical mechanics: objectivity and the origin of giant fluctuations.
Просмотров 4822 года назад
Boltzmann’s explanation of irreversibility is based on the concept of macro-states and the definition of entropy as the logarithm of the volume in phase space of the region of micro-states compatible with a given macro-state. The explanation, however, lacks an objective (i.e. non arbitrary) definition of macro-states and of the crossover between micro- and macro-scales. Here we show that this p...
Why nobody understands thermodynamics
Просмотров 1,3 тыс.2 года назад
If you studied thermodynamics and did not understand a thing, maybe this video could help. The main idea is to forget about the first and the second law (which refer to processes) and focus on the characterization of equilibrium states, which is the basic problem in equilibrium thermodynamics. This is the same approach followed by H.B Callen in his celebrated textbook on thermodynamics but expl...
Lesson 6 (5/5). Stochastic differential equations. Part 5
Просмотров 2,5 тыс.4 года назад
Lecture for the course Statistical Physics (Master on Plasma Physics and Nuclear Fusion). Universidad Complutense de Madrid. Course webpage: seneca.fis.ucm.es/parr/sp
Lesson 6 (4/5). Stochastic differential equations. Part 4
Просмотров 3,4 тыс.4 года назад
Lecture for the course Statistical Physics (Master on Plasma Physics and Nuclear Fusion). Universidad Complutense de Madrid. Course webpage: seneca.fis.ucm.es/parr/sp
Lesson 6 (3/5). Stochastic differential equations. Part 3
Просмотров 4,3 тыс.4 года назад
Lecture for the course Statistical Physics (Master on Plasma Physics and Nuclear Fusion). Universidad Complutense de Madrid. Course webpage: seneca.fis.ucm.es/parr/sp
Lesson 6 (2/5). Stochastic differential equations. Part 2
Просмотров 4,9 тыс.4 года назад
Lecture for the course Statistical Physics (Master on Plasma Physics and Nuclear Fusion). Universidad Complutense de Madrid. Course webpage: seneca.fis.ucm.es/parr/sp
Lesson 6 (1/5). Stochastic differential equations. Part 1
Просмотров 25 тыс.4 года назад
Lecture for the course Statistical Physics (Master on Plasma Physics and Nuclear Fusion). Universidad Complutense de Madrid. Course webpage: seneca.fis.ucm.es/parr/sp
Lesson 5. Markov chains
Просмотров 2,1 тыс.4 года назад
Lecture for the course Statistical Physics (Master on Plasma Physics and Nuclear Fusion). Universidad Complutense de Madrid. Course webpage: seneca.fis.ucm.es/parr/sp
Lesson 3. Quantum Ideal Gases.
Просмотров 1,3 тыс.4 года назад
Lecture for the course Statistical Physics (Master on Plasma Physics and Nuclear Fusion). Universidad Complutense de Madrid. This lecture corresponds to Lesson 3 on Quantum Ideal Gases. I'm a bit slow. I recommend watching it at speed x1.25. There are three mistakes that I've detected after recording the video, the last one is conceptually important: mins 7:43 and 8:00. I say "evaluated in" and...
Sortis in ludis: Euler, juegos y paradojas.
Просмотров 4676 лет назад
Jornada Euler. 14 de febrer de 2007. Universitat Politècnica de Catalunya. Juan M. R. Parrondo (Universidad Complutense de Madrid) Sortis in ludis: Euler, juegos y paradojas Euler atacó problemas de probabilidad y estadística en varias ocasiones. Una de las más interesantes es el trabajo “Vera estimatio sortis in ludis” (La correcta evaluación del riesgo en un juego), publicado póstumamente y e...
Extremely Helpful, thank you prof.
could you kindly explain how v = white noise at around 24:20. Thanks!
v= white noise results from applying the overdamped limit to the Langevin equation of a free particle. The position of a Brownian particle in the overdamped limit is the Wsiener process, and the velocity is the white noise. If you include inertia, then the velocity is an Orstein-Uhlenbeck process, which tends to white noise when the correlation time goers to zero.
Amazing lecture! Around 45:28 , I believe the second term on the right of the FPE for Stratanovich interpretation must have a positive sign and not negative. Please correct me if I am wrong☺
Sure, you're right. The error is there from minute 44:20 to 45:30 approx. Thanks for pointing it out!!
thank you so much
very interesting sir...Please post more problems and theorems
cool explanation, thnnks
Excellent!
May I ask? I might be wrong but Ito calculus seems to have a problem with oscillating terms. Assume that instead of dot(x) we had i dot(x). Then we would expect that the fluctuations and random noises would just change the frequencies in the problem. But due to the extra term, g^2 ->-g^2 we would have a d.amping. Why? What do I miss?
really enjoyable lecture
One of the better explanations, thank you Juan!
👍 Thanks
Outstanding set of lectures on SDE
Thank you for the lecture series. Loved the combination of intuition and math. Amazing!
Amazing lecture. This should have a lot more views. I wish you would put more of your lectures online. It's rare to find someone who breaks down difficult concepts to clearly and concisely.
I'm not sure what I'm misunderstanding at 35:00, the expectation of w(t) is 0 b/c w(t) ~ N(0, σ*root(t)), shouldn't the expectation of w(t)^2 = Var(W) which equals σ*root(t)? rather than σ^2*t
I use the notation N(mu,sigma) to indicate a gaussian random variable with average mu and dispersion sigma (=> variance=sigma^2). I've just learned that the standard notation is N(mu,sigma^2). Sorry for the confusion! The Wiener process at time t is a gaussian variable with zero average and dispersion sigma*sqrt(t) => variance = sigma^2*t
@@juanmrparrondo1375 ah thank you so much Professor, this video helped me greatly
Great. please give the link for lesson 1 and lesson2
Sorry. This is part of a course and lessons 1-2 are not available. Thanks!
Dear Dr. Parrondo, thank you for the lectures. I have one question. How one should deal with stochastic DE when the stochastic part of the equation is nonlinear in \xi, but some function of \xi?
Hi Roman. Thank you for your comment. I do not know the answer to your question. Just a couple of comments: If the noise appears in a term as g(x) h(xi), where xi is Gaussian white noise and h(.) is a nonlinear function, then this is equivalent to considering a non-gaussian noise. There are theorems that prove that some nongaussian white noises are equivalent to gaussian white noise (see for instance the limit of a dichotomous noise in the book by Horsthemke and Lefebver, Noise Induced Transitions). But I don't know if this is general. In fact, the dichotomous noise cannot be written as a nonlinear function of a gaussian noise. If the noise appears in a more complicated way, like sin(x xi) then I guess things are even more complicated. But I don't know the literature on this topic.
@@juanmrparrondo1375 Thank you for a prompt response. I am reading a couple of articles on supression/introduction of chaos in nonlinear systems by random phase in the drive (e.g., doi:10.1155/2011/53820, doi:10.1016/j.chaos.2004.04.014, both refers Runge-Kutta-Verner method for simulation of SDE). Unfortunately I fail to find anything else but Ito calculus for SDE numerical methods which it its turn deals only with linear \xi(t) at r.h.s. Now I came to some understanding that it seems that for simulation it should be enough to deal with argument of cos(\omega*t+\sigma\xi(t)) as separate differential equation in the SDE system, so there would be one additional equation d\theta/dt=\omega+\sigma*d(\xi(t))/dt (e.g.,10.1006/jsvi.1996.0869). However, I am still confused about usage of white noise and Wiener process as \xi(t). It seems that different papers mean different thing for \xi(t) in cosine argument. So I believe I still have to do some research.
Muchísimas gracias Profesor.
Very well done. You not only derive the equations, but explain the thought process involved in their development. Very helpful.
Hi, this is a most wonderful lecture ! Thank you so much for uploading this, Professor. Would it be possible for you to upload other Stochastics lectures as well ?
This lecture is very well organized and incredibly easy to follow. Thank you, professor!
Regarding equilibrium for microstates: the condition is S(a*, E) - S(A(x), E) <= k for all A in R, where R is some restricted set of observables. Intuitively, whatever the set R, objectively defined or not, and for *any* observable A in R, the function w(a, E) must assign a large volume in phase space to the observed value a = A(x), or else the difference in entropies would be large. This means that no observable A in R should be able to distinguish x from a very large set of other microstates, comparably large to the set which is typical with respect to A (the set associated with a*). I am trying to think of a counter-example: a system with some causal chain between a microscopic event and a macroscopic observable, something like the Schrödinger's cat thought experiment (I know the point here is to bypass the notion of macroscopic variables, but just for the sake of argument.) Such a system, by construction, would provide a macroscopic observable that enables us to distinguish a small set of microstates from the rest of phase space (e.g. a lamp that turns on when a particle hits a detector) Therefore, the observables in R that satisfy the above condition depend on the system. How can you define an objective set of observables R that excludes the observable "state of the lamp" from this system? Maybe the answer is that such causal links cannot exist in isolated systems, they must be drawing energy or producing entropy to operate at the level of precision required to amplify microscopic events, and so will be far from equilibrium anyway.
I've searched a lot for good SDE tutorials. This was the best video series by far. Nothing, and I mean nothing, is taken for granted. Everything is rigorously explained and even if I didn't get something there was a concrete reference to go look at and then easily jump back. Enhorabuena profesor y muchisimas gracias!
Sir Please tell me the basic books for stochastic differential equation and stochastic fractional differential equations please sir🙏
This is a good one specially if you are interested in simulations (but ok for theory as well): www.amazon.es/Stochastic-Numerical-Methods-Introduction-Scientists/dp/3527411496
Thank you very much for the courses. I followed them part 1 to 5. It would be great if you could also post some lessons on PSDE :)
haha just in time, i got an exam tomorrow !!
This was very helpful, Juan
The unique poland contrarily tempt because rice immunologically zoom out a guarded committee. bustling, sparkling russia
Hello SIr i am completely new to all of this, what basic knowledge do i need to understand stochastic differential equations or on this video is this the basic knowledge i need to know. i hope what is said makes sense?
I think you can follow it if you know differential calculus, a bit of differential equations, and basic probability (gaussian variables, central limit theorem, average, independent random variables)
Dear Mr. Parrondo, thank you so much for your exceptionally clear and incredibly helpful lectures! It might be very obvious, but I get lost once during the derivation of the Fokker-Planck equation (around 31:00). I would be very grateful if you could help me out of my confusion! When replacing the average $\langle \dot A (t) angle$ by its integral definition $\int dx ho(x,t) \dot A (t)$, I don't understand why $$ \langle \dot A angle = \int \frac{\partial ho}{\partial t} A $$ holds or how one would get there...
Hia Lana, thanks for your comment!! "A" is introduced as an arbitrary function of x, so it's A(x). Then we define A(t) as A(x(t)). Then, the average is <A(t)>=<A(x(t))>= int dx rho(x,t) A(x). Imagine for instance that A(x)=x, then <A(t)>=<x(t)>=int dx rho(x,t) x. Now, if we differentiate the equation <A(t)>=<A(x(t))>= int dx rho(x,t) A(x) with respect to time, we get: d <A(t)> / dt = int dx [\partial rho(x,t)/\partial t] A(x) The l.h.s. is \langle \dot A angle I hope this will help!
@@juanmrparrondo1375 Now it all makes sense, thank you very much!
Is there a video of the first lecture?
Sure, you have the whole course in my youtobe channel
@@juanmrparrondo1375 Prof. Parrondo, Sorry, I had missed your reply. Thank you very much!
Loved it
thank you. please what is the programme that you use in these video (writing in table)
Hi. It is doceri: doceri.com/. It's a great app for the ipad. You can record the writing and play it in the class at the speed that you wish.
@@juanmrparrondo1375 muchos gracias
Corona took away a lot from our lives but in exchange it gave us the professors like you. You are one of the best teacher one can have. Thank you professor for so understandable explanation of obscure concepts. Anticipating more lectures from you in future.
Thank you Sir for such an excellent lecture
Very nicely explained, I very much liked the balance between intuition and formality.
I did a course in stochastic process and was a nightmare as the professor spent too much time in silly demonstrations and proof, but this videos just explain the concept and applications in just five minutes which is great simple and efficient. Many thanks for this videos please keep posting more videos.
Gracias Juan
Thank you very much juan. Do you have any notes available for the public . Thank you in advance.
Thank you Prof Parrondo for your wonderful lectures! I have watched your Part 1-3 and you have demystified the complex world of SDE! Can I ask how did you derive \sigma^2 \Delta t at 8:44 mins? I am confused. Is it because dW is \Delta x and in your earlier videos, you define \frac{\Deltax^2}{\Delta t} = \sigma^2?
28:00 great explanation
can you share the pdf to mail id abdsaleem111@gmail.com
cool, thank you very much for the lecture Prof. Could you please post some videos about SPDE (Stochastic Partial Differential Equation)?
prof. this is great. do you mind uploading other lectures in stat physics from your course?
would be great!
It's so interesting... And i'll take a Time to understand so well the Stratonovich's integral
great video thanks!
Very informative and concise. Thanks! :D
Thank you!!
Bravo, I am your fan now. Please post the whole lectures.
Thanks!! You have all the lectures on my channel
It is very good. Please post more. Thank you.