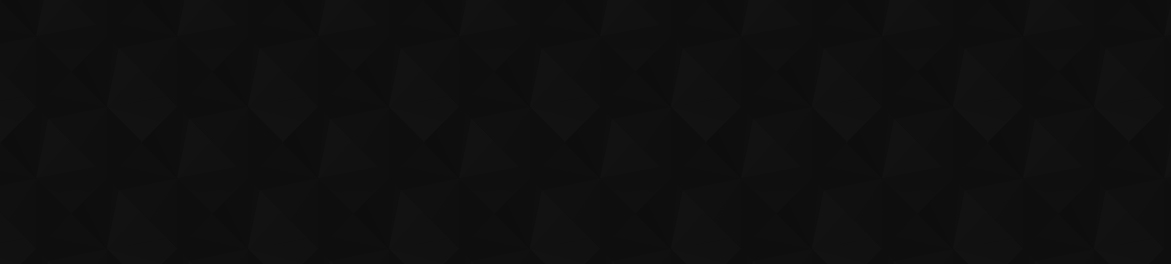
- Видео 262
- Просмотров 969 086
Probability, Stochastic Processes - Videos
США
Добавлен 8 окт 2014
Student-centered teaching. Considering the short attention span of modern students, short (5 - 10 to 15 min) short videos are presented here illustrating how to solve problems in Probability. Problems are solved in detail in real time so that the viewer can (hopefully concentrate and) follow the thought process, the techniques involved. Most of the episodes attempt to solve only one specific problem and get a single idea across, although some of them solve multiple examples. There are enough solved problems even for the lazy student, who has no intention to sit down and watch an entire presentation. The emphasis here is on solving problems entirely, on the derivation details, rather than just going over the results. You keep solving problems, and like driving (for most of us), you should get better at it with practice. That is the hope anyway.
For Lecture Notes, go to www.mhhe.com/engcs/electrical/papoulis/ippt.mhtml
For Lecture Notes, go to www.mhhe.com/engcs/electrical/papoulis/ippt.mhtml
Frequency Modulation and Bessel Differential Equation
A short lecture on FM & Bessel Differential Equation by Prof. Pillai.
Просмотров: 152
Видео
Applications of Bessel Function (Spring & Brownian Motion)
Просмотров 2298 месяцев назад
A short lecture on applications of bessel functions by Prof. Pillai.
Bessel Function and its Applications (FM and Damped-aged Spring)
Просмотров 1558 месяцев назад
A lecture on bessel functions and some of its applications by Prof. Pillai.
Embedded Stochastic Processes
Просмотров 1979 месяцев назад
A short video on Embedded Stochastic Processes by Prof. Pillai
Gibbs' Phenomenon
Просмотров 2529 месяцев назад
Video Lecture by Prof. Pillai on the topic Gibbs' Phenomenon
Autonomous Package Buddy 'See and Go' - Demo 4
Просмотров 3413 года назад
Demo video for Autonomous Package Buddy Vehicle. Goal here is to carry out a package delivery from A to B thru elevators and all. Navigate thru the current environment by 'see and go', no stored maps. Previous videos: Package Buddy Demo Video 1: ruclips.net/video/vftkO3paOgc/видео.html Package Buddy Demo Video 2: ruclips.net/video/WhneSA4Aar0/видео.html Package Buddy Demo Video 3: studio.rucli...
Autonomous Package Buddy Demo - 3
Просмотров 1453 года назад
Demo video for Package Buddy: Autonomous Vehicle Previous videos: Package Buddy Demo Video 1: ruclips.net/video/vftkO3paOgc/видео.html Package Buddy Demo Video 2: ruclips.net/video/WhneSA4Aar0/видео.html
Autonomous Package Buddy Demo2
Просмотров 923 года назад
Demo video for Package Buddy: Autonomous Vehicle
Pillai: Beam Forming
Просмотров 1,1 тыс.3 года назад
Advantages of using multiple receiver sensors are discussed including beam forming and peak sidelobe levels of -13.2 dB under uniform phase steering.
Pillai: High Resolution Methods for Direction Finding
Просмотров 8783 года назад
High resolution methods for direction finding using a set of sensors.
Pillai: High Resolution Receiver Array Processing
Просмотров 3233 года назад
Pillai: High Resolution Receiver Array Processing
Pillai: Capon vs Linear Prediction Processing
Просмотров 2373 года назад
Pillai: Capon vs Linear Prediction Processing
Pillai Lecture 9 Stochastic Processes to Systems and Input-Output Relations Fall20
Просмотров 1,6 тыс.3 года назад
Pillai Lecture 9 Stochastic Processes to Systems and Input-Output Relations Fall20
Pillai Lecture 7 Conditional Probability Distributions and Applications Fall20
Просмотров 1,3 тыс.3 года назад
Pillai Lecture 7 Conditional Probability Distributions and Applications Fall20
Pillai Lecture 6 Two Random Variables and Their functions Fall20
Просмотров 2,2 тыс.3 года назад
Pillai Lecture 6 Two Random Variables and Their functions Fall20
Pillai: Lecture 4 Mean, Variance and Characteristic Functions of a Random Variable Fall20
Просмотров 2,8 тыс.3 года назад
Pillai: Lecture 4 Mean, Variance and Characteristic Functions of a Random Variable Fall20
Pillai Lecture 8 Stochastic Processes Fundamentals Fall20
Просмотров 3,2 тыс.3 года назад
Pillai Lecture 8 Stochastic Processes Fundamentals Fall20
Pillai: Lecture 5 Moments and Functions of Two Random Variables Fall20
Просмотров 1,8 тыс.3 года назад
Pillai: Lecture 5 Moments and Functions of Two Random Variables Fall20
Pillai: Lecture 3 Random Variables and Their Functions Fall20
Просмотров 3,1 тыс.4 года назад
Pillai: Lecture 3 Random Variables and Their Functions Fall20
Pillai: Lecture 2 Repeated Trials Fall20
Просмотров 2,2 тыс.4 года назад
Pillai: Lecture 2 Repeated Trials Fall20
Pillai: Lecture 1 Independence and Bayes' Theorem Fall20
Просмотров 7 тыс.4 года назад
Pillai: Lecture 1 Independence and Bayes' Theorem Fall20
Pillai: Stochastic Processes-6: Stochastic Sampling Theroem and Ergodic Processes
Просмотров 4404 года назад
Pillai: Stochastic Processes-6: Stochastic Sampling Theroem and Ergodic Processes
Pillai: Stochastic Processes-3 "Best Estimators and Best Linear Mean Square Error Estimators"
Просмотров 7154 года назад
Pillai: Stochastic Processes-3 "Best Estimators and Best Linear Mean Square Error Estimators"
Pillai: Stochastic Processes-5 . "Matched Filter Receiver, Hilbert Transfrom and FM"
Просмотров 2624 года назад
Pillai: Stochastic Processes-5 . "Matched Filter Receiver, Hilbert Transfrom and FM"
Pillai: Stochastic Processes-7: "Pulse Compression; Markov Chains"
Просмотров 2654 года назад
Pillai: Stochastic Processes-7: "Pulse Compression; Markov Chains"
Pillai: Stochastic Processes-4 Power Spectrum of Stationary Stochastic Processes
Просмотров 6154 года назад
Pillai: Stochastic Processes-4 Power Spectrum of Stationary Stochastic Processes
Pillai: Nonnegative Definite Matrices, Eigenvalues and Eigenvectors
Просмотров 5814 года назад
Pillai: Nonnegative Definite Matrices, Eigenvalues and Eigenvectors
great lecture sir !! I am reading your book also . So proud of you from India😇
sir can you tell me where i can find video for joint density and joint gaussian random variable
@@vilgax9955 ruclips.net/video/z2O7pBCVDb0/видео.htmlsi=CWkH-pZ5vnByNG0n ruclips.net/video/z2O7pBCVDb0/видео.htmlsi=CWkH-pZ5vnByNG0n
extremely good lecture
sir can I get your lecture notes as the link above in bio isn't opening.
@abdurrasheed4968 Scroll down and look under 'Probabilty Lecture Notes' on my web page below: engineering.nyu.edu/faculty/unnikrishna-pillai
Nive video and well explained. Can I please have a video on independent and identically distributed random variables
@@Henry-b1t It is already there! Check ruclips.net/video/6M_98BAwX3c/видео.htmlsi=cr50GA_w3CXSv4BV
sir please do lectures on your book Probability and Stochastic Processes also, I shall be thankffull
So how do you actually get the prolate spheroidal function solutions?
You can solve the integral equation numerically. Or discretize it, the equation then becomes an eigenvalue/eigenvector problem of a positive definite matrix. Also, there are three classic Bell System Lab older publications on this topic by Slepian, Landau et.al. Good to go through those.
A huevo, sí hay estimador insesgado para la desviación estándar
Great video and gives a very clear explanation of which limits to use, how to find the dividing line etc - many other lectures assume you know that stuff already
if you are coughing throughout, it's better not to attend the class. First you might be transmitting it to others and second you are ruining this gold video plus disturbing thousands of viewers from learning
At 27:14, it should have been d/du(dY/dt).du/dt = ... ... Middle term should be dY/du and not dY/dt, as I have there, although the error is fixed in the next step.
Thanks a lot Professor Pillai , please kindly upload a video for the derivation of signal to noise ratio in FM system , and why FM is better than AM , thanks and best regards .
one of the greatest explanations I have ever seen. I bout his book on random. variables and stochasitc processes so these lectures have been of great assistance. Thanks you for your contributions to students internationally Dr. Pillai🎉🎉
Amazing Lecture !
At 58:11 - 58:58 the negative sign outside the middle term should have been a +. That mistake is corrected later at 1:02:27
Dear Professor Pillai , have a good day , please could you kindly upload a video for signal to noise ratio derivation in analog FM & AM systems , indicating why FM is more better reception than AM , thanks and best regards
Thank you Professor Pillai for the video! Could I ask two quick questions: why can you replace the x in f_xy(x, y) with yz (time 2:40 ), and also why can you move the z^{a-1) out of the integral (time 4:24 ) if z depends on x and y? Maybe my mistake is that I am mistaking the rv's X, Y, and Z with the density function inputs x, y, and z.
I think you are confused for several reasons. First, at 4:24 , look into the remaining integral and the variable there. The variable is y. So all other terms can be pulled out, including z^(a-1). That is standard operation. As for 2:40 substitution, pl study Leibnitz' rule for differentiation under integrals. There are plenty of video on this.
@@probabilitystochasticproce2625 Makes sense. Thank you so much!
Thank you Professor Pillai , eager to watch more videos , really helpful. God bless you
Thank you. Very nice. More next week.
Can you recommend textbooks for embedded stochastic processes? I am a Grade 12 student. I know Probability Theory, Measure Theory, Linear Algebra, Boolean Algebra, and a litle bit of other fields like Graph Theory. I am currently studying Time Series Analysis as part of my research. I encountered stochastic processes, but I think they are just random walks. Also, my research is about utilizing the leftover prediction error from any non-stationary Time Series Models. The error can be commonly approximated by a Gaussian. I will then use parametric estimation and the inverse of the inverse transform to turn it into uniform. I will then binarize this uniform distribution. Finally, I will use Bayesian Inference in Binary. Basically, I am manipulating conditionals and assuming independence to simplify my equations. Well, I don't really know if this is already done though, so I am stilll a bit worried.
My intention here was to show that one can realize better compression properties by playing with x(t) that need not be even stationay, and yet the overall behavior can have stationary structure leading to various applications. Restructuring stochastic processesusing dual freedom.
I have a problem with stationarity and ergodicity. People in the stackmathexchange and even wikipedia can't seem to agree. I also don't understand ergodicity. Can I get your views on this? Thanks.
Ergodicity is a deeper property compared to stationary processes. More conditions on the covarianc matrix of the process need to be met for ergodicity. Essentially, one realization of such a process has to duplicate ALL of its properties. For one, you need to wait long enough ...
It is always treat to listen to your lectures. Very nicely gibbs overshoot, phenonmenon accepted. But it occurs in Only discontinuous functions. No matter where you truncate Your fourier series it will occur.
Thank you. Very nice ...
Ars you going to upload the rest of the lecture ? Looking forward to more lecture videos.
Thanks a lot Professor Pillai , please we need more videos on communications and digital communications
Very clearly explained, Thank you sir
Dear Professor Pillai , have a good day , please could you kindly upload new video series in Stochastic processes and communication engineering , we like these videos , great explanation from you , thanks and best regards
The teacher is very good. I like the speed
Nice try to understand students
Dear Professor Pillai , have a good day , please could you kindly upload new video lectures specially on Stochastic processes , thanks and best regards
Thank you, Professor for your great contents.
Hi, thank you very much for this valuable lesson. I have a question that I would be grateful if you would answer: How do you make the conclusion that you need to maximize the posterior? Shouldn't we consider maximizing the overall integral? Also one last question, when we define the cost R, we do not have a parameter of selection but just a summation of different costs. How did we determine that we need to select some i over another? I would be really grateful if you could elaborate on these parts a little.
Look the second line. The integration region Z_i is to be determined so as to minimize R. The way you pick that region Z_i is as follows: For every vector point r, compute all \Lambda_i as shown there and the lowest among them, points to the index i and the region Z_i to which that data point r would go to for integration. That way overall R will be minimized. For the special case when c_ij=1, c_jj=0, minimization of \Lambda_i is the same as maximization of the f(r|H_i) and thatdefines Z_i to which that r would go for integration In reality, you don't do the intrgeation since you only have one set of data set r. From the above arguments, to minimize R, you maximize the a-posterori pdf of H_i given r to figurecout which hypothesis is most likely behind it . I hope it is clear now.
It's a very good video. Thank you sir
oh!!!! my God who is pillai.I was so exited about him .100% confidence .teaching as a simple task for him wawwwwwwwwwwwwwwwwwwwww
Sir warping space time
Thank you sir
Thank you sir
Studying one day before exam....grt explanation...what a legend
Waos😮
Dear Professor have a good day , please could you kindly upload any new lectures of the topics you teach , we really like the mathematical derivation during explaining the material , thanks and best regards
Mohamad. Ramadan greetings. I am teaching an undergraduate communication theory course this semester: FM, matched filtering, optimal signal design, etc. Next semester, I will teach a graduate course. I can do it then.
@@probabilitystochasticproce2625 Ok , Thanks and best regards , we are very grateful for your helpful and valuable lectures
If theta is constant then what is the meaning of dtheta inside the integration?
That is a mistake, typo, by me. Integration is with respect to the random variavle x, not \theta, so it should be dx.
Thank you for this video, it was very helpful. I'd like to read more on this subject. Is there a name for this distribution ? Papoulis and Pillai (2002) says that the Nakagami distribution is a generalization to the Rayleigh distribution. However the PDFs are different. And wikipedia says that the Noncentral chi distribution is the generalization of the Rayleigh distribution, but the PDF still doesn't match the one from the video.
As I mentioned at the beginning of the video there, 'Generalized Rayleigh' is my mis-characterization. I justify it by showing that if you let n=2 there, you get the ordinary Rayleigh distribution, so in that sense, this is a generalized Rayleigh. There could be other distributions with more paramters that reduce to ordinary Rayleigh if you set some parameter there to zero, etc. That is certainly possible. If you can figure out the analogy, this is similar to Gamma vs. exponential. I derived this out of curiosity, I don't know where to find more on it ... but all this could be somewhere. You need to look ...
My professor made me sleep in the class when he taught us about this topic. It was so boring. I'm really glad I found this on RUclips. 33' RUclips lecture >>>>>>>> 4hrs class lecture. Mad Respect, sir!
can you provide the link to the notes or mention the name of the book?
You can find a lot of my lecture notes at Lecture Slides www.mhhe.com/engcs/electrical/papoulis/ippt.mhtml This video contents are in Lecture 14. Also .... LECTURE NOTES faraday.emu.edu.tr/ee571/lecture_notes.htm Book here is the classic Papouls' Probability, Random Variables and Stochastic Processes, McGraw Hill, 2002.
24:45 why is it z/2?
Look at the small triangle within which we need to integrate f(x,y). Compute the coordinates of the upper vertex of the triangle. If you do it right, you will get (z2, z/2). So the inner integral variable x goes over the horizontal strip marked there, and the outer variable y goes from 0 to z/2.
question please is there a video about the varianz of non indepedent variables
Even more generally, you can find Var(aX+bY) : ruclips.net/video/gytSryh9o7c/видео.html ruclips.net/video/8t5KwypkbTc/видео.html
ruclips.net/video/xxpLVtMF_Hg/видео.html
Dear Sir, How are you? I hope you are doing good. I have recorded very first video in which I am demonstrating construction of trigonometric table. I will feel good if you watch the video.The link is ruclips.net/video/xxpLVtMF_Hg/видео.html
Thank you. I watched your video. Good that you are able to arrange the trigonometric function values in a compact form.
I have not met a probability professor this good. His first principle approach to questions is amazing.
Hello Professor Pillai, I think at 22:20 the second term should be RxyRyy^{-1}R*xy, instead of R*xyRyy^{-1}Rxy.
Yes, you are right. That was a copying mistake on my part. You can fix it easily. Thanks.
Dear Sir I want to learn convolution theorem that you use in solutions of distribution functions
Convolution comes up in linear system theory in finding the output given tthe input f(t) and system impulse response g(t). Please look up any signal analysis or linear system book on how to convolve two functions f(t) and g(t) or its discrete version. There is a formula, you need to learn how to do it thru some examples. Then, the same operation shows up in finding the probability density function of Z =X+Y, when X and Y are independent random variables.
@@probabilitystochasticproce2625 Thank you so much Is there any video of yours
Dear Sir I have got a book 'Analysis of Linear Systems' by David k. Cheng. Now I got what the convolution theorem is.
덕분에 과제했습니다 thx