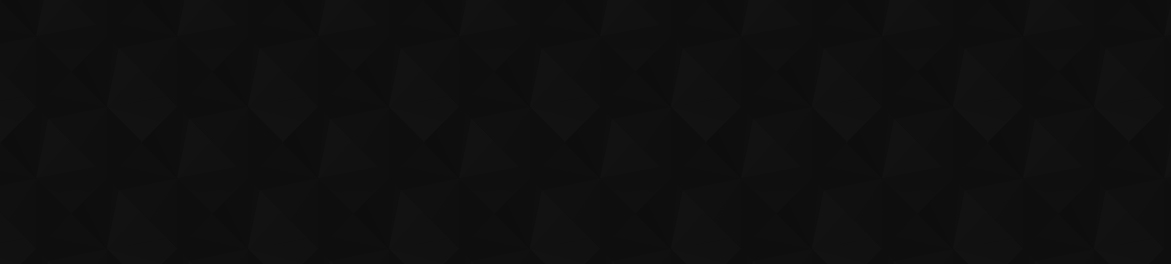
- Видео 295
- Просмотров 390 993
Valence Labs
Канада
Добавлен 18 окт 2021
Harnessing computation to radically improve lives.
Learn more: www.valencelabs.ca/
Learn more: www.valencelabs.ca/
Neural Entropy | Akhil Premkumar
Portal is the home of the AI for drug discovery community. Join for more details on this talk and to connect with the speakers: portal.valencelabs.com/logg
We examine the connection between deep learning and information theory through the paradigm of diffusion models. Using well-established principles from non-equilibrium thermodynamics we can characterize the amount of information required to reverse a diffusive process. Neural networks store this information and operate in a manner reminiscent of Maxwell's demon during the generative stage. We illustrate this cycle using a novel diffusion scheme we call the entropy matching model, wherein the information conveyed to the network during tr...
We examine the connection between deep learning and information theory through the paradigm of diffusion models. Using well-established principles from non-equilibrium thermodynamics we can characterize the amount of information required to reverse a diffusive process. Neural networks store this information and operate in a manner reminiscent of Maxwell's demon during the generative stage. We illustrate this cycle using a novel diffusion scheme we call the entropy matching model, wherein the information conveyed to the network during tr...
Просмотров: 1 016
Видео
scPRINT: pre-training on 50 million cells allows robust gene network predictions | Jérémie Kalfon
Просмотров 48421 день назад
Portal is the home of the AI for drug discovery community. Join for more details on this talk and to connect with the speakers: portal.valencelabs.com/logg A cell is governed by the interaction of myriads of macromolecules. Such a network of interaction has remained an elusive milestone in cellular biology. Building on recent advances in large foundation models and their ability to learn withou...
Learning Equivariant Non-Local Electron Density Functionals | Nicholas Gao
Просмотров 630Месяц назад
Portal is the home of the AI for drug discovery community. Join for more details on this talk and to connect with the speakers: portal.valencelabs.com/logg The accuracy of density functional theory hinges on the approximation of non-local contributions to the exchange-correlation (XC) functional. To date, machine-learned and human-designed approximations suffer from insufficient accuracy, limit...
Consistent Diffusion Meets Tweedie: Training Exact Ambient Diffusion Models with Noisy Data
Просмотров 736Месяц назад
Portal is the home of the AI for drug discovery community. Join for more details on this talk and to connect with the speakers: portal.valencelabs.com/logg Ambient diffusion is a recently proposed framework for training diffusion models using corrupted data. Both Ambient Diffusion and alternative SURE-based approaches for learning diffusion models from corrupted data resort to approximations wh...
BindCraft: one-shot design of functional protein binders
Просмотров 1,5 тыс.Месяц назад
Portal is the home of the AI for drug discovery community. Join for more details on this talk and to connect with the speakers: portal.valencelabs.com/logg Protein-protein interactions (PPIs) are at the core of all key biological processes. However, the complexity of the structural features that determine PPIs makes their design challenging. We present BindCraft, an open-source and automated pi...
Meta Flow Matching: Integrating Vector Fields on the Wasserstein Manifold | Lazar Atanackovic
Просмотров 1,1 тыс.Месяц назад
Portal is the home of the AI for drug discovery community. Join for more details on this talk and to connect with the speakers: portal.valencelabs.com/logg Numerous biological and physical processes can be modeled as systems of interacting entities evolving continuously over time, e.g. the dynamics of communicating cells or physical particles. Learning the dynamics of such systems is essential ...
Derivative-Free Guidance in Continuous and Discrete Diffusion Models | Xiner Li and Masatoshi Uehara
Просмотров 527Месяц назад
Portal is the home of the AI for drug discovery community. Join for more details on this talk and to connect with the speakers: portal.valencelabs.com/logg Diffusion models excel at capturing the natural design spaces of images, molecules, DNA, RNA, and protein sequences. However, rather than merely generating designs that are natural, we often aim to optimize downstream reward functions while ...
A long-context RNA foundation model for predicting transcriptome architecture | Ali Saberi
Просмотров 4592 месяца назад
Portal is the home of the AI for drug discovery community. Join for more details on this talk and to connect with the speakers: portal.valencelabs.com/logg Linking DNA sequence to genomic function remains one of the grand challenges in genetics and genomics. Here, we combine large-scale single-molecule transcriptome sequencing of diverse cancer cell lines with cutting-edge machine learning to b...
Fine-tuning Flow and Diffusion Generative Models | Carles Domingo-Enrich
Просмотров 8842 месяца назад
Portal is the home of the AI for drug discovery community. Join for more details on this talk and to connect with the speakers: portal.valencelabs.com/logg Dynamical generative models that produce samples through an iterative process, such as Flow Matching and denoising diffusion models, have seen widespread use, but there has not been many theoretically-sound methods for improving these models...
Probabilistic Inference in Language Models via Twisted Sequential Monte | Rob Brekelmans
Просмотров 9872 месяца назад
Portal is the home of the AI for drug discovery community. Join for more details on this talk and to connect with the speakers: portal.valencelabs.com/logg Numerous capability and safety techniques of Large Language Models (LLMs), including RLHF, automated red-teaming, prompt engineering, and infilling, can be cast as sampling from an unnormalized target distribution defined by a given reward o...
MolGPS - A Foundational GNN for Molecular Property Prediction
Просмотров 4243 месяца назад
Scaling deep learning models has been at the heart of recent revolutions in language modelling and image generation. Practitioners have observed a strong relationship between model size, dataset size, and performance. However, structure-based architectures such as Graph Neural Networks (GNNs) are yet to show the benefits of scale mainly due to the lower efficiency of sparse operations, large da...
Geometric deep learning framework for de novo genome assembly | Lovro Vrček
Просмотров 6403 месяца назад
Portal is the home of the AI for drug discovery community. Join for more details on this talk and to connect with the speakers: portal.valencelabs.com/logg The critical stage of every de novo genome assembler is identifying paths in assembly graphs that correspond to the reconstructed genomic sequences. The existing algorithmic methods struggle with this, primarily due to repetitive regions cau...
An Open MetaGenomic corpus for mixed-modality genomic language modeling | Andre Cornman
Просмотров 4423 месяца назад
Portal is the home of the AI for drug discovery community. Join for more details on this talk and to connect with the speakers: portal.valencelabs.com/logg Biological language model performance depends heavily on pretraining data quality, diversity, and size. While metagenomic datasets feature enormous biological diversity, their utilization as pretraining data has been limited due to challenge...
Propensity Score Alignment of Unpaired Multimodal Data
Просмотров 4663 месяца назад
Multimodal representation learning techniques typically rely on paired samples to learn common representations, but paired samples are challenging to collect in fields such as biology where measurement devices often destroy the samples. This paper presents an approach to address the challenge of aligning unpaired samples across disparate modalities in multimodal representation learning. We draw...
Tokenized and Continuous Embedding Compressions of Protein Sequence and Structure | Amy X. Lu
Просмотров 7513 месяца назад
Portal is the home of the AI for drug discovery community. Join for more details on this talk and to connect with the speakers: portal.valencelabs.com/logg Existing protein machine learning representations typically model either the sequence or structure distribution, with the other modality implicit. The latent space of sequence-to-structure prediction models such as ESMFold represents the joi...
Discrete Flow Matching | Andrew Campbell
Просмотров 1,4 тыс.4 месяца назад
Discrete Flow Matching | Andrew Campbell
Day 5 - Introducing Bioptic | Vlad Vinogradov
Просмотров 2244 месяца назад
Day 5 - Introducing Bioptic | Vlad Vinogradov
Day 5 - Hackathon Introduction | Cas Wognum
Просмотров 1414 месяца назад
Day 5 - Hackathon Introduction | Cas Wognum
Day 5 - Open-Source Initiatives & Benchmarking Efforts | Karmen Condic-Jurkic
Просмотров 2254 месяца назад
Day 5 - Open-Source Initiatives & Benchmarking Efforts | Karmen Condic-Jurkic
Day 5 - Protein Folding & Design | Alex Tong
Просмотров 7714 месяца назад
Day 5 - Protein Folding & Design | Alex Tong
Day 5 - LLMs in Drug Discovery | Andres M Bran
Просмотров 6754 месяца назад
Day 5 - LLMs in Drug Discovery | Andres M Bran
Lab 4 - Target Deconvolution Explanation | Ali Denton & Kristina Ulicna
Просмотров 2674 месяца назад
Lab 4 - Target Deconvolution Explanation | Ali Denton & Kristina Ulicna
Day 4 - Modeling Population Dynamics | Charlotte Bunne
Просмотров 6404 месяца назад
Day 4 - Modeling Population Dynamics | Charlotte Bunne
Day 4 - Causal Discovery & Representation Learning | Jason Hartford
Просмотров 3804 месяца назад
Day 4 - Causal Discovery & Representation Learning | Jason Hartford
Day 4 - Multimodal Omics & AI | Sébastien Lemieux
Просмотров 3204 месяца назад
Day 4 - Multimodal Omics & AI | Sébastien Lemieux
Day 4 - Phenomics in Drug Discovery: Microscopy and Machine Learning | Anne Carpenter
Просмотров 1,1 тыс.4 месяца назад
Day 4 - Phenomics in Drug Discovery: Microscopy and Machine Learning | Anne Carpenter
Lab 3 - De Novo Generation Explanation
Просмотров 2294 месяца назад
Lab 3 - De Novo Generation Explanation
Day 3 - Harnessing Geometric ML for Molecular Design | Michael Bronstein
Просмотров 3494 месяца назад
Day 3 - Harnessing Geometric ML for Molecular Design | Michael Bronstein
Day 3 - Synthesizability & Molecular Synthesis | Connor Coley
Просмотров 6084 месяца назад
Day 3 - Synthesizability & Molecular Synthesis | Connor Coley
Day 3 - Exploring Molecular Space & Active Learning | Yoshua Bengio
Просмотров 6074 месяца назад
Day 3 - Exploring Molecular Space & Active Learning | Yoshua Bengio