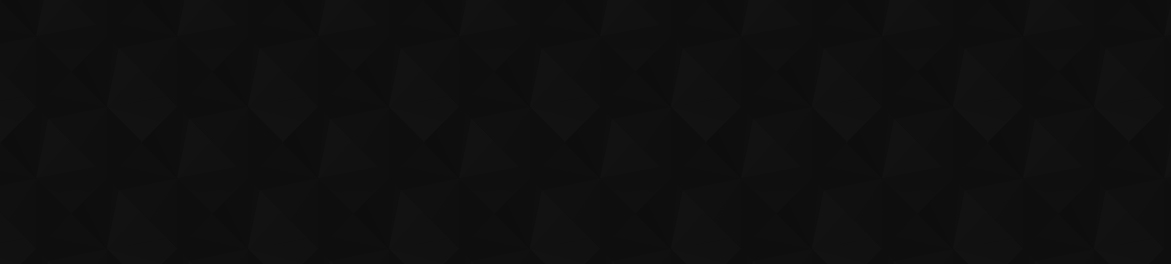
- Видео 71
- Просмотров 36 889
Learning with Limited and Imperfect Data
Добавлен 18 май 2021
Official RUclips channel for the Learning with Limited and Imperfect Data Workshop @ CVPR 2021.
l2id.github.io/
l2id.github.io/
L2ID @ ECCV 2022 Introduction
Workshop introduction by the organizers. Please note that live Q&A sessions have been cancelled. Post all questions directly to the RUclips videos in the comments section.
Просмотров: 618
Видео
Boyi Li: SITTA: Single Image Texture Translation for Data Augmentation
Просмотров 3832 года назад
Recent advances in data augmentation enable one to translate images by learning the mapping between a source domain and a target domain. Existing methods tend to learn the distributions by training a model on a variety of datasets, with results evaluated largely in a subjective manner. Relatively few works in this area, however, study the potential use of image synthesis methods for recognition...
Boshen Zhang: Learning from Noisy Labels with Coarse-to-Fine Sample Credibility Modeling
Просмотров 3052 года назад
Training deep neural network~(DNN) with noisy labels is practically challenging since inaccurate labels severely degrade the generalization ability of DNN. Previous efforts tend to handle part or full data in a unified denoising flow via identifying noisy data with a coarse small-loss criterion to mitigate the interference from noisy labels, ignoring the fact that the difficulties of noisy samp...
Sukmin Yun: OpenCoS: Contrastive Semi-supervised Learning for Handling Open-set Unlabeled Data
Просмотров 3352 года назад
Semi-supervised learning (SSL) has been a powerful strategy to incorporate few labels in learning better representations. In this paper, we focus on a practical scenario that one aims to apply SSL when unlabeled data may contain out-of-class samples - those that cannot have one-hot encoded labels from a closed-set of classes in label data, i.e., the unlabeled data is an open-set. Specifically, ...
Holger Caesar: Autonomous vehicles from imperfect and limited labels
Просмотров 3262 года назад
Abstract: The past decade has seen enormous progress in autonomous vehicle performance due to new sensors, large scale datasets and ever deeper models. Yet this progress is fueled by human annotators manually labeling every object in painstaking detail. Newly released datasets now focus on more specific subproblems rather than fully labelling ever larger amounts of data. In this talk I will tal...
Yu Cheng: Towards data efficient vision-language (VL) models
Просмотров 2412 года назад
Abstract: Language transformers have shown remarkable performance on natural language understanding tasks. However, these gigantic VL models are hard to deploy for real-world applications due to their impractically huge model size and the requirement for downstream fine-tuning data. In this talk, I will first present FewVLM, a few-shot prompt-based learner on vision-language tasks. FewVLM is tr...
SangYun Lee: Learning Multiple Probabilistic Degradation Generators for Unsupervised Superresolution
Просмотров 1102 года назад
Unsupervised real world super resolution (USR) aims to restore high-resolution (HR) images given low-resolution (LR) inputs, and its difficulty stems from the absence of paired dataset. One of the most common approaches is synthesizing noisy LR images using GANs (i.e., degradation generators) and utilizing a synthetic dataset to train the model in a supervised manner. Although the goal of train...
Rabab Abdelfattah: PLMCL: Partial-Label Momentum Curriculum Learning for Multi-label Classification
Просмотров 1152 года назад
Multi-label image classification aims to predict all possible labels in an image. It is usually formulated as a partial-label learning problem, given the fact that it could be expensive in practice to annotate all labels in every training image. Existing works on partial-label learning focus on the case where each training image is annotated with only a subset of its labels. A special case is t...
Nir Zabari: Open-Vocabulary Semantic Segmentation using Test-Time Distillation
Просмотров 2252 года назад
Semantic segmentation is a key computer vision task that has been actively researched for decades. In recent years, supervised methods have reached unprecedented accuracy; however, obtaining pixel-level annotation is very time-consuming and expensive. In this paper, we propose a novel open-vocabulary approach to creating semantic segmentation masks, without the need for training segmentation ne...
Niv Cohen: "This is my unicorn, Fluffy": Personalizing frozen vision-language representations
Просмотров 1522 года назад
Large Vision & Language models pretrained on web-scale data provide representations that are invaluable for numerous V&L problems. However, it is unclear how they can be used for reasoning about user-specific visual concepts in unstructured language. This problem arises in multiple domains, from personalized image retrieval to personalized interaction with smart devices. We introduce a new weak...
Hamza Khan: Timestamp-Supervised Action Segmentation with Graph Convolutional Networks
Просмотров 672 года назад
We introduce a novel approach for temporal activity segmentation with timestamp supervision. Our main contribution is a graph convolutional network, which is learned in an end-to-end manner to exploit both frame features and connections between neighboring frames to generate dense framewise labels from sparse timestamp labels. The generated dense framewise labels can then be used to train the s...
Jiageng Zhu: SW-VAE: Weakly Supervised Learn Disentangled Representation Via Latent Factor Swapping
Просмотров 1402 года назад
Representation disentanglement is one important goal of representation learning that benefits bunches of downstream tasks. To achieve this goal, many unsupervised learning representation disentanglement approaches have been developed. We propose a novel weakly-supervised training approach to train representation disentanglement called SW-VAE. We describe the approach of generating pairs of inpu...
Niv Cohen: Out-of-Distribution Detection Without Class Labels
Просмотров 3412 года назад
Out-of-distribution detection seeks to identify novelties, samples that deviate from the norm. The task has been found to be quite challenging, particularly in the case where the normal data distribution consist of multiple semantic classes (e.g. multiple object categories). To overcome this challenge, current approaches require manual labeling of the normal images provided during training. In ...
Andong Tan: Unsupervised Adaptive Object Detection with Class Label Shift Weighted Local Features
Просмотров 1032 года назад
Due to the high transferability of features extracted from early layers (called local features), aligning marginal distributions of local features has achieved compelling results in unsupervised domain adaptive object detection. However, such marginal feature alignment suffers from the class label shift between source and target domains. Existing class label shift correction methods focus on im...
Leonid Karlinsky: Different facets of limited supervision
Просмотров 3562 года назад
Leonid Karlinsky: Different facets of limited supervision
Vadim Sushko: One-Shot Synthesis of Images and Segmentation Masks
Просмотров 2482 года назад
Vadim Sushko: One-Shot Synthesis of Images and Segmentation Masks
Bharath Hariharan: When life gives you lemons: Making lemonade from limited labels
Просмотров 2852 года назад
Abstract: Many research directions have been proposed for dealing with the limited availability of labeled data in many domains, including zero-shot learning, few-shot learning, semi-supervised learning and self-supervised learning. However, I argue that in spite of the volume of research in these paradigms, existing approaches discard vital domain knowledge that can prove useful in learning. I...
Abhay Rawat: Semi-Supervised Domain Adaptation by Similarity based Pseudo-label Injection
Просмотров 2382 года назад
Abhay Rawat: Semi-Supervised Domain Adaptation by Similarity based Pseudo-label Injection
Ruiwen Li: TransCAM: Transformer Attention-based CAM Refinement for Weakly Supervised Segmentation
Просмотров 2572 года назад
Ruiwen Li: TransCAM: Transformer Attention-based CAM Refinement for Weakly Supervised Segmentation
Ishan Misra: General purpose visual recognition across modalities with limited supervision
Просмотров 8452 года назад
Ishan Misra: General purpose visual recognition across modalities with limited supervision
Sharon Li: How to Handle Data Shifts? Challenges, Research Progress and Path Forward
Просмотров 4862 года назад
Sharon Li: How to Handle Data Shifts? Challenges, Research Progress and Path Forward
Xiuye Gu: Open-Vocabulary Detection and Segmentation
Просмотров 1,3 тыс.2 года назад
Xiuye Gu: Open-Vocabulary Detection and Segmentation
Yinfei Yang: Learning Visual and Vision-Language Model With Noisy Image Text Pairs
Просмотров 2092 года назад
Yinfei Yang: Learning Visual and Vision-Language Model With Noisy Image Text Pairs
L2ID CVPR2021 Panel Sessions - Part 2
Просмотров 1153 года назад
L2ID CVPR2021 Panel Sessions - Part 2
L2ID CVPR2021 Panel Sessions - Part 3
Просмотров 1173 года назад
L2ID CVPR2021 Panel Sessions - Part 3
L2ID CVPR2021 Panel Sessions - Part 1
Просмотров 1613 года назад
L2ID CVPR2021 Panel Sessions - Part 1
A Causal View of Compositional Zero Shot Recognition
Просмотров 953 года назад
A Causal View of Compositional Zero Shot Recognition
Cluster-driven Graph Federated Learning over Multiple Domains
Просмотров 2343 года назад
Cluster-driven Graph Federated Learning over Multiple Domains
Rethinking Ensemble Distillation for Semantic Segmentation Based Unsupervised Domain Adaptation
Просмотров 1473 года назад
Rethinking Ensemble Distillation for Semantic Segmentation Based Unsupervised Domain Adaptation
ProFeat: Unsupervised Image Clustering via Progressive Feature Refinement
Просмотров 2753 года назад
ProFeat: Unsupervised Image Clustering via Progressive Feature Refinement
Heyyy can you please provide source code or repository??
well done! guys. Can I ask some questions about the method you used?
awesome
Amazing work!
Very interesting tutorial. Great work!
Great paper. Any intuition on why the ViT Lite learns better with learnable PEs compared to sinusoidal?
Is this paper accepted by CVPR 2021?
This was a really good talk. In fact, one of the best explanations on entropy minimization. Thank you very much for your good work.
Thanks! I was so confused about why entropy minimization can push the decision boundary
Nice. You are welcome to watch my playlist : ruclips.net/p/PLdkryDe59y4Ze9_12JhWu3cs-lOGYwYeD Eran
Problem with audio between 12:49 and 13:45. Would it be possible to upload a transcription for this part? Thanks.
Thanks for the talk and for sharing the Toronto Annotation Suite. Is there any reason aside from publication time/project timing that StyleGAN was used instead of StyleGAN2?
Very informative! Thanks
plots not starting at 0 on the y axis + no error bars make it quite hard to actually compare performance...
Thanks for the nice talk. Your idea of weighting unlabeled data points in the semi-supervised context is certainly related to learning from "soft" probability distributions as in ReMixMatch. There, you also have some sort of data weighting by potentially less extreme pseudo target distributions (as opposed to hard labeling as for instance in FixMatch). That being said, I think many semi-supervised pseudo-labeling approaches implicitly implement such kind of instance weighting. Nevertheless, I like seeing that you are treating this in a more explicit yet "classical weighting fashion". Great work!
Thanks for the talk. I have a couple questions what other methods are there to promote flat minima and why what this method chosen? How much slower does this make training since there is now a adversarial optimization program to solve?
Hi I really enjoyed the talk. I have two questions, do the transformers introduced have a similar ability as current visual transformers to improve performance with dataset size or do they saturate at some point? Secondly, it appears a core design pattern of this model is combining transformers with convolution what is the right tradeoff here? Do you think there might be some way to control the tradeoff between low bias(transformers) and higher bias(convolutoion) in a way to enable a "smooth" tradeoff?
Thanks for the nice talk. Is there an intuition about why sequence pooling improves performance? It looks like its learned weighting is not dependent on the input. Does this pooling learn some bias like always giving a larger weight to the patches that came from the center of the image?
We can think of the pooling layer as acting similar to an attention layer which (as you correctly point out) weights the results of the transformer architecture. We have not tested which convolutions are being weighted the most, but that's an interesting idea. We have performed some saliency analysis and found that our network focuses on the same parts of an image that we, as humans, would naturally gravitate towards (indicating that our network is learning desired features and not auxiliary features like backgrounds/environmental settings). When we found our network did this better than comparative networks (same number of parameters) like ResNet.
Interesting talk. Did you evaluate your approach on any previously established cross-domain few-shot learning benchmarks?
Very nice talk! There are also many works on using computer game engines, such as GTA, to generate labeled datasets, What do you think of this direction in comparison with GAN-based approaches?
Thanks for sharing the awesome works! I have a question about unsupervised pre-training for object detection works (for both ReSim and DETReg). I am wondering whether these two methods are generic to different detector architectures. If not, is it possible to have a unified self-supervised object detection method, which is generic to any kind of detector architecture? What are the fundamental or key properties of this kind of method?
Great Talk! The idea that aligned and uniformity in representation space generalize across tasks & domains seems pretty powerful, especially when we do not know our downstream task a priori. Can we perhaps use these metrics to dynamically explore the multiview/augmentation space (ie. use the metrics to guide something like AutoAugment during the contrastive learning phase)?
Thank you for the talk, this is very interesting work! How were images selected to be annotated for DatasetGAN (i.e., human versus random versus metric)? I would think that this is a very important step to help get the most from each labeled generated image.
Outstanding talk! In the part concerning data privacy, how did you annotate the faces? After you have shown that face obfuscation does not hurt image classification, I would think that the cost of further annotating datasets would be the only reason that a lab/company might not apply this in their work. I would think the cost is very reasonable, but I am not the one making such decisions :). It seems this could be automated with a face detector, but does that just move the problem into the training of the face detector?
Thanks so much James! Absolutely, we used an automated face detector and then verified using crowdsourcing. This significantly reduced the cost compared to annotating manually from scratch, although the crowdsourcing part was still necessary exactly for the reasons you mentioned, to mitigate bias/errors in automatic face detection. Check out arxiv.org/abs/2103.06191 (sec 3) for details.
Thank you for the wonderful talk! In part 3, the motivation of the idea seems very promising (not all unlabeled data are equal, so we can use a bi-level optimization to weight the unlabeled datapoints). What type of distribution do you observe for the lambda values? I wonder if it would be more close to a bimodal distribution (data is either good or bad), normal distribution, or something else, and what type of insight we could gain from observing this distribution.
Interesting method with impressive results. Two questions: 1) Did you study varying the value of the mixing weight alpha? How does that change results? 2) Did you evaluate your approach in the cross-domain few-shot setting?
thanks for your interest. 1) We do have an experiment varying the alpha values in Figure 4 (openaccess.thecvf.com/content/CVPR2021W/LLID/papers/Zhao_ReMP_Rectified_Metric_Propagation_for_Few-Shot_Learning_CVPRW_2021_paper.pdf). 2) Sorry. We didn't evaluate our method on this task.
Looks like the Convolutional layers are the trick to make the compact transformers faster? What are the effective operators in the transformer to make us choose it over CNNs?
Transformers learn more long range spatial information while CNNs learn more localized information. Both have their advantages. This can lead to some better generalization in some cases but of course you're going to need to consider what works best for your needs.
In order to annotate the radar data as suggested by the talk, how can we effectively align the radar data and the pose estimation predictions in temporal space? Since multiple sensors might have their own FPS, what would be the method to align them to make sure the proposed method works?
Excellent works. Could you provide explanations that why the improvements relative to the baseline of ViViT on Kinetics 600 and SomethingSomething V2 are smaller than that on the other datasets? How can we define the proper dataset size to successfully train a model LIke VIVIT?
Thank you for the excellent talks. I have two questions. For ReSim, did you study comparing spatial regions that are not in the same spatial location, by some small perturbations? Because I wonder if enforcing feature consistency over windows that have some degree of jitter may improve performance? For On-Target Adaptation, on the target data you start with a scratch model before contrastive learning -- did you study the impact of starting with various pre-trained models here?
This is an awesome application of few-shot learning with impressive results. I wonder if the approach can generalize across different subject areas? Have you studied this in any other area besides programming? (i.e. perhaps mathematics)?
Fantastic talk, and very interesting insights. Question: can you speculate as to the implications of these findings in the situations where one may be transferring very large models trained on very large datasets, i.e. CLIP, or ViT-G/14 scale?