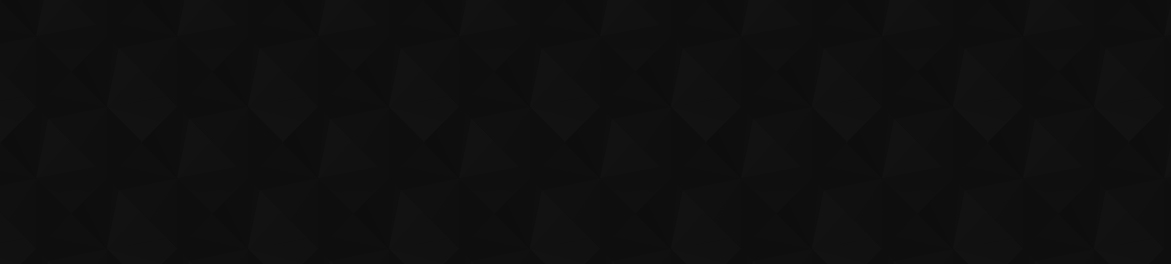
- Видео 9
- Просмотров 51 691
Introduction to Data-Centric AI
Добавлен 5 дек 2022
Lecture 9: Data Privacy and Security
Introduction to Data-Centric AI, MIT IAP 2023.
You can find the lecture notes and lab assignment for this lecture at dcai.csail.mit.edu/lectures/data-privacy-security/.
You can find the lecture notes and lab assignment for this lecture at dcai.csail.mit.edu/lectures/data-privacy-security/.
Просмотров: 1 908
Видео
Lecture 8: Encoding Human Priors: Data Augmentation and Prompt Engineering
Просмотров 1,6 тыс.Год назад
Introduction to Data-Centric AI, MIT IAP 2023. You can find the lecture notes and lab assignment for this lecture at dcai.csail.mit.edu/lectures/human-priors/.
Lecture 7: Interpretability in Data-Centric ML
Просмотров 1,6 тыс.Год назад
Introduction to Data-Centric AI, MIT IAP 2023. You can find the lecture notes and lab assignment for this lecture at dcai.csail.mit.edu/lectures/interpretable-features/.
Lecture 6: Growing or Compressing Datasets
Просмотров 1,8 тыс.Год назад
Introduction to Data-Centric AI, MIT IAP 2023. You can find the lecture notes and lab assignment for this lecture at dcai.csail.mit.edu/lectures/growing-compressing-datasets/.
Lecture 5: Class Imbalance, Outliers, and Distribution Shift
Просмотров 2,9 тыс.Год назад
Introduction to Data-Centric AI, MIT IAP 2023. You can find the lecture notes and lab assignment for this lecture at dcai.csail.mit.edu/lectures/imbalance-outliers-shift/.
Lecture 4: Data-centric Evaluation of ML Models
Просмотров 3,3 тыс.Год назад
Introduction to Data-Centric AI, MIT IAP 2023. You can find the lecture notes and lab assignment for this lecture at dcai.csail.mit.edu/lectures/data-centric-evaluation/.
Lecture 3: Dataset Creation and Curation
Просмотров 4,6 тыс.Год назад
Introduction to Data-Centric AI, MIT IAP 2023. You can find the lecture notes and lab assignment for this lecture at dcai.csail.mit.edu/lectures/dataset-creation-curation/.
Lecture 2: Label Errors
Просмотров 9 тыс.Год назад
Introduction to Data-Centric AI, MIT IAP 2023. You can find the lecture notes and lab assignment for this lecture at dcai.csail.mit.edu/lectures/label-errors/.
Lecture 1: Data-Centric AI vs. Model-Centric AI
Просмотров 24 тыс.Год назад
Introduction to Data-Centric AI, MIT IAP 2023. You can find the lecture notes and lab assignment for this lecture at dcai.csail.mit.edu/lectures/data-centric-model-centric/.
Great lecture on data privacy and security! The content was very informative. Could you share some practical steps individuals can take to enhance their data security in everyday life?
Good lecture. I didn't understand the bit about how/why to incorporate entropy in the selection process, and how that would stop us from ending up with redundant examples, though -- anyone assist?
Awesome, thank you for sharing! One question though: IIUC all of this assumes that the model we plan on training is in fact a good estimator of the phenomenom we're trying to model. I understand how the algorithm works in that case. However, how do we validate that assumption? What if I'm using a terrible model, how would I know? After using confident learning to clean the datased I'd thing that I now have a better dataset, but I don't think that's achievable through a bad model.
An awesome course that doesn't have any substitutes online. Thank you so much for posting.
this course hard to learn, is there anyone just recommend any course should I take and then study this !!
paint > chalk
amazing lecture. thanks a lot
time 32:23 outliers! - look up Platypus ;-) egg laying mammal, D'oh!
What a good course, Thanks so much for sharing.
Thanks a million for making all course materials available, very wonderful course!
Thank you for sharing this course, it's fantastic!
Question: So far these lecture series is focusing on image recognition examples. Is DCAI also applicable to other data for example time series data for a predictive maintenance problem? If so, are there any fundamentals that change that need to be taken into account? Many thanks for putting these lecture online!
thanks for making this available. quality data is so fundamental for any reasoning... human or AI
thank you all!
Excellent course. Thanks a million for sharing.
5:02 Pretty funny that he misclassifies the camel spider again as he's looking at it (it's not a tick, but its not a scorpion either) - just shows how often these kind of errors happen.
Keep the good ideas going!! Employ a company like smzeus!
The formula for the t_j’s is not giving me the same values in the presentation and I have a feeling that I’m probably not applying it correctly. Can anyone explain how to get t_dog = 0.7 based on the given noisy labels and the predicted probabilities, for instance?
None of the threshold's are matching. t_dog = 0.3(1st image)+0.9(6th image)/2 = 0.6. Can someone break down for 1 class if i am wrong
@asdfghjkl743 I am getting the same values for t_j's as you. The slides are incorrect.
sum up the probabilities of each type and divide by the number of images of each type for fox, it is (0.7+0.7+0.9+0.8+0.2)/5 = 0.7 if i am not wrong
@@manigoyal4872 I think the formula on the prev slide means t_fox is only computed based on the records where its noisy label, i.e. y^tilde=fox, so only 4 images (no.2-5)
As the course comes to a close, I would like to take a moment to express my sincerest gratitude for your guidance and support throughout the lectures. It took me about a month to complete all nine lectures, including labs and notes, but I can say that this path has been the most illuminating experience in my educational life. Your unwavering dedication to teaching and commitment to my learning experience has not gone unnoticed. You have inspired me to continue learning and growing beyond the classroom. Your generosity with your time and knowledge has made a significant impact on my journey. Thank you once again for all that you have done for us.
Very informative and concise lecture! Good didactic, involving students (who seem to have good knowledge of the subject matter). Congratulations from 🇲🇽
Thanks for the amazing lecture!
Could anyone told me why the slide in 37:43 align the left most image which has noisy label:dog and higest probability 0.7 as fox to y~ = fox and y* = dog in the table?
Good find, that's a bug in the slides, images 1 and 5 should be swapped. The first image should have \tilde{y}=dog and y^*=fox.
There is a mistake in the slides, the blue circle examples are switched.
@@majovlasanovich9047 i noticed that right now because i explained that to my dad
Good catch
Thank you for sharing
This course covers unsupervised learning?
The course focuses on supervised learning, but many of the topics covered apply to unsupervised learning as well (for example, outlier detection).
Thanks for posting this! Especially a link to the lab and notes :)
Thank you so much for this !!
Thanks for posting!