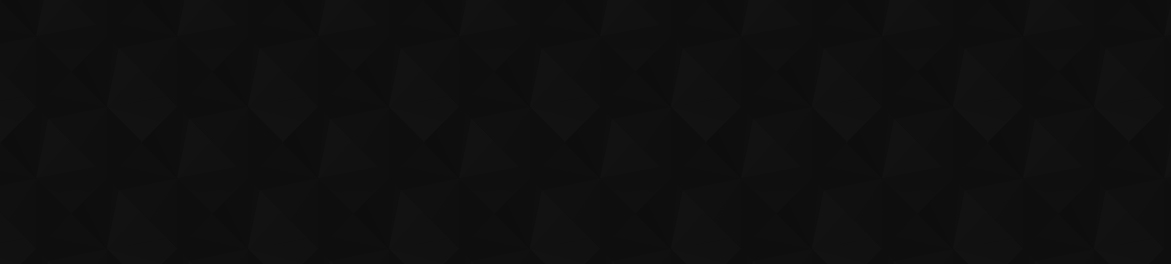
- Видео 63
- Просмотров 44 355
hamannlab
Добавлен 26 окт 2011
Andreas Hamann's Video Channel for Statistics and Data Science
University of Alberta, Department of Renewable Resources
Faculty website: www.ualberta.ca/~ahamann
Applied statistics course websites: tinyurl.com/rr480
Multivariate statistics course website: tinyurl.com/mv690
Contact info:
Office: 733 GSB (open door policy)
Email: andreas.hamann@ualberta.ca
Phone: (780) 492-6429
Fax: (780) 492-4323
University of Alberta, Department of Renewable Resources
Faculty website: www.ualberta.ca/~ahamann
Applied statistics course websites: tinyurl.com/rr480
Multivariate statistics course website: tinyurl.com/mv690
Contact info:
Office: 733 GSB (open door policy)
Email: andreas.hamann@ualberta.ca
Phone: (780) 492-6429
Fax: (780) 492-4323
Building a Deep Neural Network (DNN) core with 20 lines of base-R code from scratch
A fairly rigorous explanation of how neural networks work (with minimal hand-waving), that anyone can understand. We then program the DNN core functionality from scratch in base R, with this bare-bones architecture already being capable of ultra-fast image recognition.
Download the lab here: tinyurl.com/mv690/lab/9
Download data here: tinyurl.com/mv690/lab/9/data
Shrtcut to this video: tinyurl.com/mv690/v/dnn1
Download the lab here: tinyurl.com/mv690/lab/9
Download data here: tinyurl.com/mv690/lab/9/data
Shrtcut to this video: tinyurl.com/mv690/v/dnn1
Просмотров: 397
Видео
Installing Tensorflow-GPU and Keras for R (and Python) on Windows in 2024
Просмотров 2,5 тыс.5 месяцев назад
Installation & Hardware Guide for running Deep Neural Networks (DNNs) in R on Windows with graphics card acceleration Download the lab here: tinyurl.com/mv690/lab/9 Download data here: tinyurl.com/mv690/lab/9/data Shortcut to this video: tinyurl.com/mv690/v/dnn2 # Packages that play ball in 2024 with NVIDIA graphics card acceleration on Windows: Anaconda 2022.10 Python 3.9.13 Tensorflow 2.10.1 ...
11.5. Graphical Tools for Multivariate Statistics (mv690, graphics)
Просмотров 2522 года назад
Graphics Touch-up for Presentations and Publications. Best learning at 1.5x speed, and at 2x for review before the quiz or exam (doi.org/10.1002/acp.3899). Expand description for time stamps. 00:00 Graphical Tools Overview 01:18 High data-to-ink ratio plots 03:46 Examples for touch-up 05:31 Touch-up walk-through 06:30 File format usage in R 09:00 File format quality compared 10:36 Editing .emf ...
2.2. R Setup for Win/Mac and Working Efficiently with Scripts (mv690, r-efficient)
Просмотров 3873 года назад
Working efficiently with R in Windows and MacOS, using shortcuts and scripts. Best learning at 1.5x speed, and at 2x for review before the quiz or exam (doi.org/10.1002/acp.3899). Expand description for time stamps. 00:00 Essential Windows settings 01:51 Mac OS users start here 02:17 Using workspace shortcuts & scripts 06:24 Importing data in CSV format 12:06 Setup in Mac OS and what's differen...
2.1b. An Introduction to the R Programming Environment (mv690, r-intro)
Просмотров 1263 года назад
Introduction to the R programming language and statistical software package: what is it, where to get it, how to use it. Course website and lab downloads: tinyurl.com/mv690.
2.1. An Introduction to the R Programming Environment (rr480, r-intro)
Просмотров 3893 года назад
Introduction to the R programming language and statistical software package: what is it, where to get it, how to use it. Best learning at 1.5x speed, and at 2x for review before the quiz or exam (doi.org/10.1002/acp.3899). Expand description for time stamps. 00:00 R as a programming language 02:42 R memes 04:40 Download R 06:37 The first steps with R Other videos in Playlist 2: ruclips.net/p/PL...
3.1. The Convention of Standard Data Tables (rr480, datatables)
Просмотров 3883 года назад
Design of a standard data table that can be used for statistical analysis. Tips for data management. Best learning at 1.5x speed, and at 2x for review before the quiz or exam (doi.org/10.1002/acp.3899). Expand description for time stamps. 00:00 Experimental designs vs data tables 03:06 Standard data tables 06:33 Fixing categorical variables and IDs 09:25 Using an "Order" variable 12:38 The case...
3.3. Data Table Concepts and Variable Types (rr480, tableconcepts)
Просмотров 3703 года назад
Concepts implied by the standard data table design. Variable types (nominal, continuous) and sub-types (ratio-, interval- and ordinal-scale). Best learning at 1.5x speed, and at 2x for review before the quiz or exam (doi.org/10.1002/acp.3899). Expand description for time stamps. 00:00 Lab 2: Solution to designing a data table 05:19 Vocabulary: indepedent, dependent variables, unit, datum, data ...
1.3. Your Univariate Statistical Toolbox (rr480, toolbox)
Просмотров 2433 года назад
Statistical methods covered in RENR 480 / 580 organized by objectives and data types. Best learning at 1.5x speed, and at 2x for review before the quiz or exam (doi.org/10.1002/acp.3899). Expand description for time stamps. 00:00 Toolbox overview 01:04 Descriptive statistics 02:32 T-tests: 1s, 2s, paired 05:38 ANOVA & effect size stats 06:47 Linear, non-linear regression 07:15 Non-paramtric sta...
1.2. Acid Tests for Real Hypotheses: Science 1, Statistics 0 (rr480, hypotheses)
Просмотров 2943 года назад
Developing scientific hypotheses and research questions. Best learning at 1.5x speed, and at 2x for review before the quiz or exam (doi.org/10.1002/acp.3899). Expand description for time stamps. 00:00 What is a real scientific hypothesis? 02:20 Acid test for great hypotheses 06:28 Counter example for weak hypotheses 08:39 Saving yourself from failure Other videos in Playlist 1: ruclips.net/p/PL...
1.1. Applied Statistics for the Life & Environmental Sciences (rr480, intro)
Просмотров 5103 года назад
Course Introduction to REN R 480/580 - Applied Statistics for the Life & Environmental Sciences. Best learning at 1.5x speed, 2x for review before quiz or exam (doi.org/10.1002/acp.3899). Expand description for time stamps. 00:00 Welcome, overview 01:22 Intended audience 02:02 Course website, eClass 04:13 Course objectives 06:33 Methods overview 07:48 What we do differently 10:00 Bayesian inspi...
14.4. Lab walk trough: Interpretation of CART, MRT, randomForest examples (mv690, cart2)
Просмотров 2303 года назад
Lab walk trough: Interpretation of classification and regression trees: CART, MRT, randomForest examples. Best learning at 1.5x speed, and at 2x for review before the quiz or exam (doi.org/10.1002/acp.3899). Expand description for time stamps. 00:00 Jail-break R 02:58 CART regression example 05:11 CART classification example 06:58 Looking one step ahead 08:37 Alternative predictors 09:43 Multiv...
14.3. Multivariate Classification and Regression Trees: CART, MRT (mv690, cart1)
Просмотров 1 тыс.3 года назад
Multivariate and univariate classification and regression tree analysis: CART, MRT. Best learning at 1.5x speed, and at 2x for review before the quiz or exam (doi.org/10.1002/acp.3899). Expand description for time stamps. 00:00 Overview of methods 02:22 Unimodal associations 06:21 Interactions, predictions 10:35 The CART algorithm 13:08 Class variables as predictors 15:36 Multivariate response ...
14.2. Gradient Analysis Lab: Interpretation of CanCor, CCA, RDA Examples (mv690, gradient2)
Просмотров 1,2 тыс.3 года назад
Gradient analysis lab walk-through and interpretation of CanCor, CCA, RDA examples. Best learning at 1.5x speed, and at 2x for review before the quiz or exam (doi.org/10.1002/acp.3899). Expand description for time stamps. 00:00 Climate-vegetation example 04:29 Direct gradient interpretation 06:45 Indirect gradient interpretation 08:48 Constrained gradient interpretation Other videos in Playlist...
14.1. Multivariate Gradient Analysis: CanCor, CCA, RDA (mv690, gradient1)
Просмотров 1,7 тыс.3 года назад
Direct, indirect, and constraint gradient analyses: canonical correlations, canonical correspondence analysis, redundancy analysis. Best learning at 1.5x speed, and at 2x for review before the quiz or exam (doi.org/10.1002/acp.3899). Expand description for time stamps. 00:00 Two multivariate datasets 01:56 Analyzing associations 03:13 Direct, indirect associations 05:48 Constrained associations...
13.2. Multivariate Statistical Testing - MRPP, perMANOVA (mv690, permanova)
Просмотров 4,4 тыс.3 года назад
13.2. Multivariate Statistical Testing - MRPP, perMANOVA (mv690, permanova)
13.1. Multivariate Inferential Statistics, MANOVA, MRPP, perMANOVA (mv690, inferential)
Просмотров 7543 года назад
13.1. Multivariate Inferential Statistics, MANOVA, MRPP, perMANOVA (mv690, inferential)
12.3. Multivariate Clustering Techniques (mv690, cluster)
Просмотров 5513 года назад
12.3. Multivariate Clustering Techniques (mv690, cluster)
12.2 Distance-based Ordinations - NMDS, MDS, PCoA, PO (mv690, ordination)
Просмотров 4,5 тыс.3 года назад
12.2 Distance-based Ordinations - NMDS, MDS, PCoA, PO (mv690, ordination)
12.1. Distance-based Multivariate Methods (mv690, distance)
Просмотров 1,4 тыс.3 года назад
12.1. Distance-based Multivariate Methods (mv690, distance)
11.7. Assumptions of Classical Multivariate Methods (mv690, assumptions)
Просмотров 3253 года назад
11.7. Assumptions of Classical Multivariate Methods (mv690, assumptions)
11.6. Discriminant Analysis, MANOVA (mv690, discrim)
Просмотров 7953 года назад
11.6. Discriminant Analysis, MANOVA (mv690, discrim)
11.4. Factor Analysis (mv690, factor)
Просмотров 2673 года назад
11.4. Factor Analysis (mv690, factor)
11.2. PCA continued, Matrix Rotation (mv690, pca2)
Просмотров 8613 года назад
11.2. PCA continued, Matrix Rotation (mv690, pca2)
11.3. Lab walk-through: PCA Interpretation (mv690, pca3)
Просмотров 3853 года назад
11.3. Lab walk-through: PCA Interpretation (mv690, pca3)
11.1. The Concept of Coordinate Rotation & PCA (mv690, pca1)
Просмотров 1 тыс.3 года назад
11.1. The Concept of Coordinate Rotation & PCA (mv690, pca1)
Project support - Touch-up of R graphics in Powerpoint
Просмотров 1363 года назад
Project support - Touch-up of R graphics in Powerpoint
Project support - Graphics plots with log scales in R
Просмотров 1053 года назад
Project support - Graphics plots with log scales in R
10.3. Tests for Binomial Data (rr480, binomial)
Просмотров 2473 года назад
10.3. Tests for Binomial Data (rr480, binomial)
10.2. Non-Parametric Rank Sum Tests (rr480, ranksum)
Просмотров 2233 года назад
10.2. Non-Parametric Rank Sum Tests (rr480, ranksum)
Thank you so much for this really well-explained breakdown! I finally got the full picture thanks to you! :)
Thanks for helping me with this shit 🎉 my master thesis will profit from the additional performance boost. Kind greetings from Germany. (Edit: the same versions are working with R 4.4 and Python 3.11.9, version >3.11 is not supported by tensorflow 2.10.1)
Just trying to replicate this. Did you install Python 3.11 via Anaconda? I tried Anaconda-2024-02-1, which contains Python 3.11.7. However, that does not play ball with R.
@@hamannlab i had recently some troubles, too. So I Switched the settings to R 4.4, Python 3.11 and tensorflow 2.14.1, as there were many fatal errors occurring before, which crashed R. So I am currently using solely the CPU, which is tbh more or less as fast as the gpu Version. The bottleneck is any way in most cases the RAM.
@@Bulldogge98 Yes, for small networks (like in my lab and video examples) CPU will work, and you are right that the latest versions of Python and tensorflow are actually the fastest for CPU-based compute. That said, if your network exceeds ~1 million parameters (weights), then it starts getting rather boring, and at 10 million parameters you really need a GPU.
Ok, my gpu feel the heat of the night. 3 weeks, 21 nights for read every post on GitHub, Stackoverflow, Medium. I can’t imagine. You are like god but in better because you know how to do deep learning with R, on gpu. Great thanks, Best regards, Casual R user from France
Thank you, this really help me, a lot!
Bro you saved my ass, thank you very much
Top class mate, thank you very much
Me sirvió mucho, gracias 🤗
Thank you for your video
THANK YOU SO MUCH I HAD TO FOUGHT FOR WEEKS WITH SHIT TUTO YOU ARE THE BEST THANK YOU
Really informative video. Stumbled upon it randomly and didnt expect to gain such an insight into an actual model of neural networks. Really summarizes everything that i had read uptil this point and put that into actual code
hi, thank you very much for your software, I want to obtain the first scatter plot "characterize samples". I have the location and elevation of all my samples but for each sample, the data of collection differs (for example one was recolted in 1962, the other one in 1973 etc...). I want to konw if it's possible to specify the date in the input file so that the software could calculate it one time or if I need to use the software for each sample individually and enter the specific date. Thank you again and have a good day.
No, you have to specify "All variables for multiple years", then specify the full date range, and in the end delete the rows with years that you don't need.
Beautiful Explanation
Well explained, thank you! Do you have a tutorial on how to restrict the permutations and the strata options in adonis2?
This was great, thank you!
👍👍
This was great. Comparing a (null) population against itself to see type 1 errors.
It took me ages to understand perMANOVA, and just in this 15 minutes, I feel confident with my data. THANK YOU SOOO MUCH
Thank you for such an excellent explanation of type 1 error inflation!
Thank you very much!
Thank you! I couldn't find anything close to this online.
How does the strata argument effect this? Take for example, a time-series study. If we set strata to the individual, does this mean the data is only shuffled within an individual's level? If there's then 5 timepoints, we can only shuffle data 5 times?
👏👏very good content. Writing from Brazil. Following the entire course online. Really great material. Great classes. Please, continue to publish online courses for people all around the World.
very interesting indeed. thanks for the upload!
interesting background info, thanks!
Nice 👍
Terrific explanation!
super! you explained it clearly, thanks!
This is super helpful! The material on your site is also helpful...I was really dreading analyzing a dataset I had, but this material has made it a lot easier for me to understand! Thank you! Cheers.
This is the first video where perMANOVA has actually started to make sense to me, and I LOVE the flowchart presented at the end! Thank you so much 🥰
Definitely
Thank you a lot for this video, clear and helpful!
Very helpful! thank you!
Cool! ClimateEU allows to make very site specific data. This is very interesting software especially the merge of traditional software, R and ultra profesional ArcGIS. Greatly appreciated. Some hint how to produce longer time series like gridded data from CRU?